The Cognitive Correlates of Functional Status: A Review From the Committee on Research of the American Neuropsychiatric Association
Despite the clinical relevance of these issues, relatively few studies have explicitly investigated the association between measures of cognition and functional status. It is unclear, for example, how much variance in functional status can be explicitly attributed to cognition independent of major noncognitive covariates. Even less is known about the temporal association between longitudinal changes in cognition and changes in functional status. The relative importance of individual cognitive domains to functional outcomes is unknown, as are the best measures to predict impairment in specific capacities. It is also unknown whether cognition can be associated with functional status generally, or whether individual functional capacities are disproportionately sensitive to cognitive decline.
These issues are particularly relevant to the study of dementia. Both the Diagnostic and Statistical Manual 1 and the National Institute of Neurological, Communicative Disorders, and Stroke (NINCDS) 2 require that dementia-defining cognitive impairments be “associated” with disability. Conversely, increasing attention has been given to so-called “mild cognitive impairment.” It is the lack of disability, rather than cognitive impairment per se, that distinguishes mild cognitive impairment from dementia. Moreover, just as cognitive impairment in mild cognitive impairment occurs, by definition, without significant disability, treatment-associated improvement in cognition has also been observed in the absence of clinically detectable functional gains. Thus, cognitive efficacy alone is not sufficient for clinical drug approval, and pharmaceutical companies are under increasing pressure to demonstrate functional gains as well.
Defining “Functional Impairment”
Although there is tremendous variability in the terminology used to describe functional outcomes, two recent conceptual schemes may help link disability research across disciplines. The first is the Nagi/Institute of Medicine-World Health Organization (WHO) scheme. 3 , 4 The second is the “Disablement Process” of Verbrugge and Jette. 5 Both conceptualize functional outcomes as a hierarchically nested set of impairments that arises from fundamental deficits in “physiological” (including cognitive/behavioral) systems ( Figure 1 ).

For example, the World Health Organization’s (WHO’s) International Classification of Impairments, Disabilities and Handicaps (ICIDH) 4 distinguishes three levels of functional deficit. (A recently revised WHO “International Classification of Function” 4 replaces “impairment” with “impairment of a body function and structure,” replaces “disability” with “impairment of activity,” and replaces “handicap” with “impairment of participation.”) “Impairment” occurs at a physical, structural, organ, or system level and involves a missing or malfunctioning body part or system. Dementia is a syndrome of cognitive impairment due to structural or functional brain disease.
“Disability” occurs at the activity level. It involves disturbed function in performance of usual age-appropriate activities, such as feeding, dressing, shopping, or operating a motor vehicle. Impairments in activities of daily living (ADL) and instrumental activities of daily living (IADL) are examples of disabilities.
“Handicap” occurs at the societal level. It involves disturbed function in the performance of social roles, such as employment. In general, this review seeks to understand how cognitive impairments are related to disability, although some papers may attempt to associate cognitive impairment with handicaps. Throughout this review, we will limit the use of these words to their WHO sense.
The Disablement Process 5 , 6 represents an important modification of the first scheme. It emphasizes the role that environmental, social, and individual adaptations may play in modifying the functional impact of physical/cognitive impairment. While this scheme encourages the view that disability is not an inevitable consequence of physiological impairment, it essentially champions what the clinical literature might label as “tertiary prevention.” This emphasis is inherently less applicable to the present effort in that it obscures the specific association between cognitive impairment and disability. However, it is a useful model because it reminds us that social, environmental and physical variables must be controlled for in order to validly test the specific associations between cognition and functional outcomes.
Activities of Daily Living
Disability has been frequently conceptualized as a hierarchically arranged sequence of self-care abilities. Instrumental activities of daily living refer to capacities that are required for autonomous function within society. 7 Examples include managing finances, transportation, or the telephone. Activities of daily living refer to capacities that are essential to autonomous function at home. 8 Examples include dressing, bathing, and toileting.
The hierarchical relationship among ADLs and IADLs has been the focus of much research. 9 Decline in IADL usually begins after age 80 in community samples, and the impairment of individual activities develops sequentially (i.e., housework > transportation > shopping > meal preparation > finances). 10 There is also a sequential pattern to the loss of ADL in dementia cases. 11
Some authors tend to treat ADL and IADL as interchangeable constructs. 12 However, they may not be. ADL and IADL load on discriminable factors in analyses of community elderly samples. 10 IADL impairment is reported to develop earlier in dementia, 13 , 14 has a higher prevalence, 10 and stronger correlations with cognition 15 than ADL. IADL items rating medication management, finances, and telephone usage have the highest correlations with cognition. 10 ADL assessments suffer from ceiling effects and are not particularly useful in distinguishing levels of functioning in healthy elderly community-dwelling adults. 16 In contrast, IADL can even distinguish among noninstitutionalized levels of care. 17
Defining Cognitive “Impairment”
Another confusing issue is the definition of “impairment” used in the cognitive, as opposed to the functional assessment, literature. Cognitive performance is often judged statistically against that of “normative” groups. Performance is often discussed in terms of standard deviations (SD). By convention, performance ≤2.0 SD below an appropriate norm is widely considered to be “impaired,” although some authors accept lesser thresholds. This convention represents a level of performance which, on a normally distributed measure, has less than a 2.5% chance of being representative of the normative group. Alternatively, percentile ranks are sometimes used to convert raw scores. Scores below the second percentile are often considered “impaired.” This standard corresponds roughly to 2.0 SD below the mean. Scores between the third and eighth percentiles are often considered “borderline.”
The use of age-specific norms allows for a statistically rigorous definition of acquired cognitive impairments. However, this practice provides no information about the clinical significance of these thresholds. This fact is critical when considering cognition’s association with functional outcomes. Specific domains of cognition may vary in the relative strengths of their associations with functional status. Thus, a 1.0 SD change in one domain may have a greater impact on functional status than a 2.0 SD change in another. This explains the tendency of some authors to accept milder thresholds for cognitive “impairment” when testing the clinical significance of cognitive deficits.
Moreover, the conventional practice of using agespecific norms when predicting functional capacity may be flawed. For example, mild cognitive impairment has been defined by some authors as isolated memory deficits ≤1.5 SD below an age adjusted norm and the absence of disability. 18 However, it has been increasingly recognized that nonamnestic patterns of “mild cognitive impairment” may also exist. Isolated deficits in executive control function may be as common as memory impairment. 19 Nonetheless, memory and executive control function have different associations with functional status, and memory may have little association with functional outcomes independent of executive control function. 20 Thus, although memory loss between 2.0 and 1.5 SD below an age adjusted norm may be “mild” in the sense that it has little impact on functional status, executive control function impairment in the same range can be debilitating.
For example, the mean Executive Interview (EXIT25) 21 score among septuagenarian retirement community residents with advanced educations and few comorbid conditions is 14.6/50 (i.e., beyond the fifth percentile for young adults and near the threshold for clinically significant functional disability). 22 This is already a clinically significant level of executive impairment. An EXIT25 score of 15/50 accurately predicts the capacity of elderly patients with chronic obstructive pulmonary disease to learn to manage an inhaler. 23 Nonetheless, only 7% of retirement community residents fail the EXIT25 at 22/50. This represents a statistical threshold of 1.5 SD beyond the age-specific mean. 22 Ironically, this statistically defined “mild” executive impairment is actually near the mean for institutionalized nursing home residents. 24 These observations suggest that cognition may need to be judged against young adult performance when predicting functional outcomes. 25 Such a practice would greatly increase the prevalence of “clinically significant” cognitive impairments in older persons, but might also improve the ability of cognitive measures to predict functional outcomes. Other disciplines have dispensed with age-specific norms as they can be demonstrated to underestimate clinical risks. For example, blood glucose and blood pressure are no longer age-adjusted but instead used as continuous variables when predicting clinical outcomes.
Functional Assessment
There is little consensus regarding how best to measure functional outcomes. General overviews to this literature, including sources for assessment scales, can be found in Kemp and Mitchell, 9 McCue, 26 Park, 15 Gallo et al., 27 and Pearson. 28 Several dichotomies need to be considered when choosing a functional outcome measure.
“Subjective” Versus “Objective” Assessments
First, it is important to distinguish between “subjective”versus “objective” assessments. Functional status is commonly based on self-reported information. Though it is widely understood that cognitive impairment may eventually compromise the validity of self-report, the specific determinants of this capacity have received little study. 29 , 30 Thus, it is common practice to engage care-givers, or others familiar with the patient, to perform the ratings (“proxy”-based measures). However, this, too, is potentially flawed. Caregivers vary widely in their actual contact with the patient and in their own capacities to provide valid information.
“Performance-based” functional assessments have been developed as “objective” alternatives to self-reported measures. “Direct observation” measures require the patient to demonstrate an IADL activity to a trained observer. “Performance-based” measures often involve “motor” tasks, such as a “timed walk,” which are increasingly recognized to have strong cognitive components as well. 31 Though these are more objective, they are also frequently difficult to administer, and thus better suited to academic, as opposed to clinical, applications. Self-reported functional status can differ significantly from “objectively” acquired information, particularly performance-based assessments. 32
“Global” Versus “Specific”
Second, it is important to distinguish between “global” versus “specific” functional capacities. ADL and IADL items are generally summed to estimate “global” functional status across multiple activities. Alternatively, some measures are designed specifically to assess a single functional capacity. These notably include what we might label as “forensic” measures (e.g., driving, financial or medical decision-making, informed consent, or autonomy). The cognitive correlates of specific functional capacities are widely held to differ, both from each other and from general functional capacities, although this premise has seldom been tested empirically.
Univariate Versus Latent Constructs
Finally, it is important to distinguish between univariate functional measures, or indices, and latent multidimensional constructs. Like cognition, functional status is a multidimensional construct. Either can be conceived of as a latent construct, derived from the shared variance across multiple individual measures. 33 – 35 This approach has the advantage of reducing measurement error but has the disadvantage of developing constructs that lack face validity and are unwieldy for clinical applications.
In this article, the American Neuropsychiatric Association’s Committee on Research (ANPACOR) has investigated the published associations between cognitive and functional status measures in order to better understand the objective strength of their association and its implications for clinical diagnosis and assessment.
METHOD
It was the Committee’s intention to review the published specific partial correlations, from adjusted multivariate models, between individual measures of cognition and functional outcomes. Only continuous multivariate models (i.e., linear regression, discriminant analyses and/or factor analyses) were considered. Each of these statistical methods is capable of providing meaningful information on the relative strength of each predictor variable’s independent association with functional status. The limitations to this approach are discussed in detail below. Unadjusted univariate correlations were ignored. Wherever possible, we searched for multivariate models that included multiple cognitive predictor variables. Logistic regressions and analyses of variance (ANOVA) were also ignored, as these methods only produce “odds ratios” of dichotomous outcomes. These results are potentially misleading as adjusted models may produce seemingly high odds of relatively weak or rare effects. Where multiple functional outcomes were modeled, the results from each model were reported separately.
Results are reported as variances (i.e., R 2 ). When only the partial correlation (r) was reported, we squared it to produce this value. Standardized coefficients (β) were interpreted as partial correlations (i.e., as “r”). Unstandardized coefficients (Β) were converted to standardized coefficients before calculating their variances as follows:
β=Β × [SD of the independent/SD of the dependent variable]
Variances are reported to three decimal places. We have included data on each cognitive predictor in these multivariate models, even those that did not achieve statistical significance. In cases where a measure failed to contribute significantly to the variance in functional status independently of covariates, it was assigned an R 2 of 0.0, unless a specific partial r or R 2 was available. Detailed information on the cognitive and functional measures reported in these models, as well as their original sources, are included in a data supplement that accompanies the online version of this article.
The core of this review is based on a literature search conducted in August of 2004. We considered all English language articles and reviews published after 1966 and that were listed in the MEDLINE®, OVID®, or PUBMED® databases, and contained the keywords “ADL,” “autonomy” “capacity,” “competency,” “disability,” “frailty,” “functional capacity,” “functional impairment,” “functional outcomes,” “functional status,” “independence,” “IADLs” or “outcomes,” and “cognition,” “mental status” or “dementia.” Broad terms were used because of our impression that little data would be available at this stage in the literature’s development. This search resulted in 4,731 abstracts. Only peerreviewed analytical articles whose abstracts contained the word “regression” were retained. This resulted in the selection of 352 abstracts. These were reviewed by a member of the project team (D.R.R.), who rejected 252 irrelevant articles. Each of the remaining 100 was pulled and carefully reviewed for relevant data (i.e., multivariate models from which the partial variance in functional outcomes that can be specifically attributed to one or more cognitive measures could be derived).
The original search was then further supplemented by backtracking to original sources, and scholarly reviews of related topics. In addition, the original computer search strategy was repeated in the spring of 2005, to take advantage of the rapidly increasing volume of research in this area.
RESULTS
Our search identified 68 papers, offering 156 separate regression models, comprising 812 individual unique associations between a cognitive measure and a functional outcome. Figure 2 presents a histogram of the distribution of the source documents by publication year.

Twenty-seven references (39.7%) were drawn from the neuropsychiatric literature. Thirty-four (50%) were drawn from the geriatric literature. Seven (10.3%) were drawn from the rehabilitation or other literature.
Figure 3 presents the distribution of total variance in functional outcomes which could be specifically attributed to cognitive measures (N=156 models).

Outcomes could be specifically attributed to cognitive measures (N=156 models)
The total variance explained ranged from 0.0% to 78.0%. On average, cognition explained 21.0% of variance in functional outcomes (SD=20.2%). The median value was slightly less (i.e., 15.9%).
Figure 4 presents the mean unique variance explained as a function of cognitive domain.
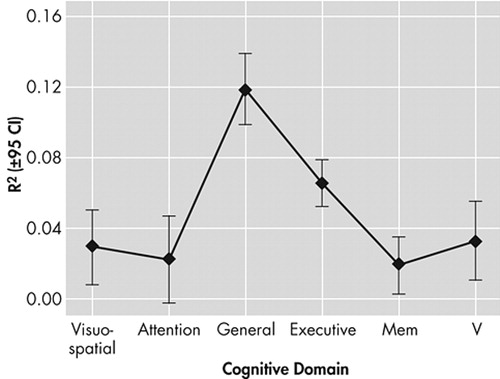
Executive and general cognitive measures explained significantly more variance in functional outcomes than did memory, attention, or verbal measures (by Tukey’s Honest Significant Difference (HSD) test for unequal N, all p<0.001). General cognition also explained significantly more variance (on average) than either executive control function (by Tukey’s honest significant difference, p=0.004) or visuospatial measures (p<0.001).
Figure 5 presents the specific unique variance in functional outcomes associated with N=35 measures of memory function (mean R 2 =1.9 [SD=6.7%]). None contributed more than 20% variance (on average) to functional outcomes.

Figure 6 presents the specific unique variance in functional outcomes associated with N=37 measures of executive function (mean R 2 =6.5 [SD=11.6%]). Only the Executive Interview (EXIT25) 21 (12 models comprising 813 subjects), the face/hand test (one model, including 26 subjects), and the Neuro-Cognitive Status Examination (NCSE) “Judgment” subscale (three models, including 86 subjects), and a “word series” task (two models, including 147 subjects) explained more than 20% of the variance, on average, in functional outcomes.

Figure 7 presents the specific unique variance in functional outcomes associated with 11 measures of general cognition (mean R 2 =11.8 [SD=16.8%]). The Blessed Information Memory Concentration (BIMC) (total score) (two models comprising 338 subjects), MDRS (five models, including 284 subjects), NeuroCog (one model comprising 46 subjects), and a “general cognition composite” (one model, including 65 subjects) 36 each explained more than 20% of the variance, on average, in functional outcomes.

A significant subset of models used either ADLs (N=24) or IADLs (N=56) as outcomes. The type of functional measure employed had no significant effect on the total variance attributable to cognition (by ANOVA: F[1, 78]=0.17, p=0.68). However, there was a trend for the explained variance in functional status to increase if executive measures were employed to predict IADLs (by ANOVA: interaction term F[1, 76]=2.72, p=0.10). In contrast, the variance explained in IADLs tended to go down (relative to ADL models) in those that lacked executive predictors ( Figure 8 ).

In contrast to ADLs and IADLs, cognition explained significantly more variance in models of forensic outcomes (i.e., decision-making capacity) (eight models comprising 214 subjects, mean variance=53.0 [SD=6.2%]; ANOVA: F[1:139]=31.32, p<0.001).
DISCUSSION
We have reviewed the contribution of cognitive measures to models of functional outcomes. Their association appears modest, at best. The median total variance in functional outcomes that could be specifically attributed to cognition was less than 20%, corresponding to a “moderate” correlation of r≤0.40. Most individual cognitive measures made statistically insignificant contributions to functional outcomes. In fact, several potential models were omitted from this review because their authors chose not to include any cognitive predictors after failing to find significant unadjusted associations. 37 , 38 Many bivariate associations were attenuated by the addition of covariates, including cognitive covariates. This suggests colinearity among the predictors and, hence, redundancy in cognitive batteries. A more selective use of psychometric testing might do an equally good job of predicting functional outcomes. In fact, simple cognitive screening tests may be more strongly associated with functional outcomes than are many formal neuropsychometric measures.
However, this analysis may have limitations which constrain the conclusions we can draw from these data. Standardized β regression coefficients only provide information on the relative importance of the predictor variables. We cannot fully describe their absolute contributions to functional outcomes because the variance shared by multiple predictors cannot be easily disentangled. 39 This means that the ranking of a particular predictor is influenced, in part, by other predictors in the model. The same predictor might rank differently in another model with different covariates.
This issue is illustrated in Figure 9 , which represents a multivariate regression model in which ADL (the dependent variable) is regressed onto three dependent variables (i.e., age, executive control function, and memory).

In Figure 9 , a Venn diagram is used to represent the variance in each variable. Age, executive control function, and memory are intercorrelated (i.e., they share variance). Age reduces the fraction of variance in ADL that might otherwise be attributed to cognition. The shaded regions represent the remaining “total” variance in ADL that can be attributed to cognition. This is equivalent to our models’ “total cognitive variances,” presented in Figure 3 .
However, executive control function is more strongly associated with ADL, in this model, than is memory. Thus, executive control function further “attenuates” memory’s apparent unadjusted association with ADL. Memory’s unique contribution to ADL, independent of both age and executive control function, is relatively small, and may not be significant. Figures 4 to 6 represent these “unique” contributions to functional status, independent of all other covariates.
The models we reviewed varied widely with regard to the cognitive measures employed, the functional measures modeled, and the subjects under study. Although any of these factors may affect the variance associated with any particular cognitive measure, we can still be confident of the relative independent contributions of each predictor. We can also test the adequacy of cognition’s total predictive power in models that include multiple cognitive predictors. The fact that a particular cognitive measure/domain might have appeared to be a better predictor than certain covariates is of little comfort, as it would probably be observed only at the expense of the total variance explained (i.e., by “underfitting” the model). Moreover, the fact that these models can never completely disentangle the association between individual cognitive measures’ functional status is mitigated by the fact that we have reviewed a large number of associations (i.e., 156 models, employing 147 cognitive, and 65 functional measures, resulting in over 800 specific unique cognitive × functional associations). In their aggregate, individual differences will tend to even out across studies.
A more serious issue is the order in which individual measures and covariates may have been “forced” into some models, particularly hierarchical stepwise regression models (HSRM). This approach is often used by authors who have a priori assumptions about the associations they are testing. Not only can this introduce a certain bias to the model, it can also affect the apparent strength of the association between each predictor and functional outcome, as a function of the order in which the authors chose to introduce them. 40 However, this is only relevant to “forced” models, as unforced predictors will enter a model in proportion to the relative strength of their associations with functional status. Only 10 of the models we examined used HSRM. The type of regression employed (i.e., HSRM versus unforced stepwise or simple multivariate regression) had no significant effect on the total variance in functional outcomes explained by cognitive predictors (i.e., by ANOVA: df [1, 154] F=2.66, p>0.05). Moreover, the specific variables forced into HSRM models should average out across the entire dataset.
Finally, the total variance in functional status that is specifically attributable to cognition will remain the same, regardless of the order in which two cognitive predictors are entered. To the extent that the associations we report in Figure 3 appear surprisingly small, they reflect a general failure of cognitive measures to attenuate the associations of other covariates and/or their redundancy (i.e., the shared variance) with other cognitive predictors. Either way, it appears that the psychometric prediction of functional outcomes could be improved by increased attention to the specific cognitive domains that are most strongly associated with disability, and a more parsimonious use of cognitive measures to achieve that goal.
A surprising finding was the relatively strong association between tests of “general” cognition and functional status. This category included brief measures, such as the Mattis Dementia Rating Scale (MDRS), 41 the Mini-Mental State Exam (MMSE), 42 and the Short Portable Mental Status Quotient (SPMSQ). 43 Such measures are often dismissed as mere “screening” tests. Nonetheless, on average, they explained significantly more variance in functional outcomes than formal tests of attention, executive control function, memory, verbal, or visuospatial function. General measures often have the added advantage of being cost-effective to deploy, and suitable for community and epidemiological studies.
One explanation for this finding may be that the models that included general measures tended to be older and less well adjusted. Specifically, they may be less likely to employ formal cognitive covariates. Our data could not support the first hypothesis. Models containing general cognitive measures were not significantly older than those without them (by ANOVA: df [1, 60] F=0.97, p<0.05). However, those containing general cognitive measures were less likely to include in addition either a memory (df [1, 151] F=4.3, p=0.04) or an executive control function measure (df [1, 151] F=5.9, p<0.02). Thus, the variance explained by “general” cognitive measures (e.g., the MDRS) in poorly adjusted models can, on occasion, be shown to be fully mediated by the specific cognitive domains they encompass. 44 Nevertheless, the source of these measures’ association with functional outcomes deserves further study. This is especially true, given that many formal measures of the cognitive domains thought to be assessed by general measures do not themselves explain much variance in functional outcomes.
We could not confirm the widely held impression that cognitive measures are more strongly associated with “higher order” IADLs than “basic” ADLs. 14 In general, cognitive measures were equally poor at estimating either IADLs or ADLs. However, the association between cognition and IADLs, as opposed to ADLs, may be domain-specific. We observed a trend for the variance explained in IADL to be increased by the use of executive predictors. In contrast, memory tended to explain less variance in IADL than executive control function, although the two were equally (modestly) associated with ADL. On average, executive control function explained at least three times as much variance in functional outcomes than memory, regardless of the specific measures employed ( Figure 4 ).
In contrast to general functional outcomes, forensic capacities appeared to be much more strongly associated with cognition. Once again, general and executive control function measures dominated these studies. 45 – 48 This is most notable in Dymek et al.’s 48 study of the capacity to give informed consent for medical care among 20 patients with Parkinson’s disease. They administered a comprehensive battery of cognitive measures and then used these data to construct multivariate regression models of increasingly restrictive standards of consent, as measured by the Capacity to Consent to Treatment Instrument. 45 , 46 , 49 Only the EXIT25 and MDRS Memory contributed significantly, independently of any other cognitive measures. The EXIT25 was the only significant correlate of a “rational reasons” standard of consent, explaining 45% of the variance. Similarly, it explained 56% of the variance in an “understanding treatment” standard. MDRS Memory explained an additional 12% of variance, and was the only significant predictor of the least restrictive “evidencing choice” standard. Although relatively few studies have specifically addressed the cognitive correlates of forensic capacities, the available studies already suggest both that cognition makes a robust contribution, and that the major cognitive correlates are similar to those that best explain general functional abilities.
Finally, we note that measures of behavior often appeared to be more strongly associated with disability than cognitive measures. 50 , 51 Thus, Norton et al. 51 found that dysexecutive behaviors, as rated by the Frontal Lobe Personality Scale (FLOPS), 52 were more strongly related to ADLs than was executive function per se, as rated by the MDRS initiation/perseveration subscale. 41 In fact, the MDRS’s association was completely mediated by measures of apathy and depression rather than by executive function. The relatively strong association between behavior and functional status is possibly an artifact of need for a single rater to measure both the dependent and the independent variables in many such models. However, it is also possible that behavioral assessments may have stronger validity as predictors of functional outcomes than do cognitive measures. This is consistent with the rather modest range of associations between cognition and disability found in this review, and might have important implications for dementia case-definitions and clinical trial outcome selection. Such studies suggest that behavior mediates cognition’s association with disability. This focuses attention on the cognitive correlates of behavior and opens up the possibility of behaviorally based mild cognitive impairment and dementia case definitions. At the very least, it implies that cognition should seldom be used alone to predict functional capacity. More accurate predictions might be obtained through multidimensional assessments.
Implications
These findings appear to leave neuropsychiatry on the horns of a dilemma. Either cognition is not as strongly associated with disability as we might imagine, or currently available measures are inadequate to demonstrate their true association. There might be many reasons for the latter interpretation. Cognitive testing can be criticized for educational, linguistic, or cultural biases and low ecological validity. Alternatively, the validity of functional status measures may be questioned, particularly those that rely on patient self-report or the report of cognitively impaired, or socially distant relatives. Future research should target both domains for the development of new assessment methods ( Table 1 ).
![]() |
Though it is clear that we are not likely to demonstrate the “true” association between cognition and functional status until valid “gold standards” are available in both domains, this review reveals that the current state of the art fails to support the widespread use of cognitive measures to predict measurable changes in functional outcomes.
This does not preclude the use of cognitive testing for clinical purposes. In clinical practice, the utility of a predictive measure is often judged by its sensitivity, specificity and adequate positive and negative predictive power given the anticipated base-rates of impairment in specific populations. When functional impairment can be dichotomized, techniques such as logistic regression or receiver operating characteristic (ROC) curves can provide useful actuarial information to the clinician. A significant limitation of this review is that it does not address the utility of cognitive measures as predictors of dichotomous clinical outcomes. However, the small amount of shared variance in the models we reviewed does speak to possible inadequacies in our models of cognitively mediated functional declines.
Another explanation for the generally weak associations we found might be that cognition and functional status are nonlinearly related. 22 A growing body of latent growth curve models shows significant nonlinearity in the rates of change in cognition. 20 , 22 , 53 – 57 Natural systems are often characterized by nonlinear transitions. It may be that there exist thresholds for clinically significant cognitive impairments that are not well described by linear regression models (of the types reviewed in these studies).
The true association between cognition and functional status may also be obscured by potent baseline covariates (such as age). Longitudinal studies may offer a better approach to studying the specific association between cognition and functional status. Stuck et al. 58 have reviewed a small number of such studies and found cognitive impairment to be a relatively strong predictor of functional decline. However, their analysis assessed only the quality of the statistical association between these variables. It did not address the strength of the association (as in this study). Moreover, few of the reviewed studies longitudinally covaried measures of cognition and functional status. However, when this is actually attempted, the rates of change in cognition and functional status can be shown to be more strongly associated with each other than their cross-sectional baseline associations suggest. 20 , 59
Another explanation may include selection biases that accrue from certain patterns of cognitive impairment. For example, severely cognitively impaired persons may resist study recruitment and/or follow-up. Conversely, mild executive impairments may favor subject recruitment and/or alter subject assessment of perceived study risks. 60
Finally, it may be that our conceptualization of “cognition” is still not comprehensive, and that there are more relevant aspects waiting to be described. After all, the psychometric enterprise is itself barely a century old, and many of the measures used in these studies have origins in cognitive models that are decades old. Executive function itself escaped clinical attention until the 1990s, although it is one of the stronger predictors of functional status we identified. 61
Factor analyses suggest a psychometric bias toward measures of memory and verbal abilities. This may render such batteries insensitive to functional outcomes, as these cognitive dimensions proved to be poor predictors of variance in functional status in this review. For example, Loewenstein et al. 62 factor-analyzed an extensive battery of psychometric and functional status measures among 166 patients with Alzheimer’s disease. Six factors were extracted. However, none of the psychometric measures in Loewenstein et al.’s battery loaded on the factors associated with functional outcomes.
A bias toward measures of memory and verbal abilities, at the expense of nonverbal and executive function, may also render psychometric batteries less sensitive to right hemisphere and/or frontal lesions. These are relatively strongly associated with age and functional status. 53 , 59 Ardila and Pineda 63 factor-analyzed a battery of 32 nonverbal measures in 156 subjects ages 26 to 60. Five factors explained 72.6% of the variance in this battery. Executive control function emerged as the dominant factor and accounted for 30% of the total variance. In contrast, “memory” explained <8% of the variance. Pursuing the cognitive correlates of behavior might be another approach to the detection and description of other “dark regions” of cognition.
Our review also suggests that the neuropsychiatric and geriatric/rehabilitation literatures could benefit from closer collaboration. For example, the neuropsychiatric literature might benefit from a more rigorous treatment of functional status. Despite the fact that there is an extensive literature on this subject, including formal conceptual schemes 4 , 5 and well validated assessment methods, there is still no consensus within the neuropsychiatric field regarding 1) the types of functional deficits which are most strongly associated with cognition, 2) the dimension(s) of cognition which is/are most relevant to functional outcomes, 3) the relevance of different types of disability or handicap to dementia case finding, or 4) the minimum severity of disability required to establish certain diagnoses (notably mild cognitive impairment and dementia). Seven of 26 (27%) models we identified in the neuropsychiatric literature used self-reported ADL or IADL as their only functional outcome.
On the other hand, the disability literature also suffers from inattention to neuropsychiatry. Sixty-nine percent of the models that included global cognitive screening measures were derived from the geriatric/rehabilitation literature. Although “general” cognitive measures are relatively strong predictors of functional outcomes ( Figure 7 ), they may underestimate executive control function impairment and are attenuated by executive control function measures. 64 Models that are limited to general cognitive screening measures may underestimate both cognition’s true association with functional status and the prevalence of disabling cognitive decline. For example, Spector and Kemper 65 estimate that 58% of cognitively impaired persons with IADL impairments require >21 hours of home care/week. However, 45% of supposedly “noncognitively impaired” persons present with IADL limitations that require a similar level of supervision. It remains to be seen if such “noncognitively impaired” persons with disabilities are truly free of executive control function impairments. If they are not, then the class of persons with “all cause dementia” may have to be substantially revised upward.
Neuropsychiatry can also inform the development of functional status measures. For example, although it is widely understood in neuropsychiatry that cognitive impairment may compromise the validity of self-report, the specific determinants of the capacity to give report on functional outcomes have received little study. 30 Loss of “insight” into functional capacities may be related to other types of anosognosia, which in turn has been associated with right hemisphere lesions and nonverbal tests of executive control, such as clock-drawing. 66
Caregiver ratings may not fare much better than patient self-rated measures of functional status. Wadley et al. 67 examined the reliability associated with caregiver versus patient self-rated versions of financial capacity in Alzheimer’s disease. As expected, caregiver ratings were judged as more valid than patient self-ratings. However, even caregiver ratings had poor test-retest reliability. Performance-based functional measures such as the Direct Assessment of Functional Status (DAFS), 68 the Independent Living Scale (ILS) 69 and the Structured Assessment of Independent Living Scales (SAILS) 70 may offer an alternative to traditional self-report. Alternatively, it may be feasible to screen for the capacity to provide self/informant-rated functional outcome information using relatively simple screening measures. 71 Functional outcome measures might also benefit from more rigorous empirical testing of their cognitive requirements.
The question of cognition’s association with functional outcomes is particularly relevant to dementia research. Current diagnostic schemes universally agree that cognitive impairment alone is insufficient to justify a diagnosis of dementia. Instead, these impairments must “account for” disability. Unfortunately, diagnostic criteria do not specify how this disability is to be assessed and whether disability alone (as opposed to handicap) is sufficient for the diagnosis.
Moreover, the fact that specific dimensions of cognition appear to disproportionately account for the observable variance in disability has the potential to revolutionize dementia case finding. This is because current case definitions insist on memory loss as an “essential” feature of dementia. However, memory impairment does not appear to contribute much variance to multivariate models of disability ( Figures 4 and 5 ). Thus, memory impairment does not appear to satisfy the requirement for a “disabling” cognitive decline. Executive control function appears more likely to explain the disability of demented cases 20 and thus remains a candidate for dementia’s “essential” cognitive impairment. However, the prevalence of disabling executive control function impairment is high and might significantly alter our conceptualization of who is or is not “demented.” 61
Similarly, mild cognitive impairment has been defined by cognitive impairment in the absence of disability. 72 However, there has been little rigorous attention to the empirical validation of the “absence of disability.” Thus, mild cognitive impairment samples vary widely with regard to their observed levels of disability. 73 , 74 In particular, so-called “amnestic” mild cognitive impairment might be expected to have relatively little disability given memory’s weak effects on functional outcomes ( Figure 3 ). In contrast, isolated executive control function impairment, which cannot now be coded as “dementia” in the absence of memory impairment, risks to be labeled as “subclinical” despite its stronger empirical association with functional outcomes. 17
Finally, it is most likely that many “amnestic” mild cognitive impairment cases actually do have both comorbid executive control function impairments and disability. 19 Such cases could plausibly meet criteria for “dementia” if either their functional status or executive control function were more rigorously assessed. At present, mild cognitive impairment cases are often distinguished from dementia solely on the basis of a Clinical Dementia Rating (CDR) Scale score. 75 However, this measure has not been validated as a measure of disability and may select for mild cognitive impairment cases that cannot be distinguished from dementia on the basis of their IADL. 76
In summary, the variance in functional status that can be specifically attributed to cognition is surprisingly modest. Psychometric batteries may have to be redesigned to maximize their capacity to capture the variance in functional outcomes, and to minimize redundancy and respondent burden. Some cognitive domains may be more relevant to functional capacity than others. This is of particular significance to dementia case finding, epidemiology, and treatment. Moreover, executive control function measures are particularly strong correlates of forensic capacities, including medical or financial decision making. “General” cognitive screening tests are also relatively strong correlates of functional status. This observation deserves further study. Progress may be made by integrating the extensive literature on functional status with the equally extensive literature on cognitive assessment.
1 . American Psychiatric Association: Diagnostic and Statistical Manual of Mental Disorders, 4th ed. Washington, DC, American Psychiatric Publishing, 1994Google Scholar
2 . McKhann G, Drachman D, Folstein M, et al: Clinical diagnosis of Alzheimer’s disease: report of the NINCDS-ADRDA work group under the auspices of the Department of Health and Human Services Task Force on Alzheimer’s Disease. Neurology 1984; 34:939–944Google Scholar
3 . Nagi S: Some conceptual issues in disability and rehabilitation, in Sociology and Rehabilitation. Edited by Sussman M. Washington, DC, American Sociological Association, 1965Google Scholar
4 . World Health Organization: International Classification of Impairments, Disability and Handicaps. Geneva, Switzerland, 1993Google Scholar
5 . Verbrugge LM, Jette AM: The disablement process. Soc Sci Med 1994; 38:1–14Google Scholar
6 . Pope AM, Tarlov AR (eds): Disability in America: Towards a National Agenda for Prevention. Institute of Medicine, Division of Health Promotion and Disease Prevention, Washington, DC, National Academy Press, 1991Google Scholar
7 . Katz S, Ford AB, Moskowitz RW, et al: Studies of illness in the aged: the index of ADL: a standardized measure of biological and psychosocial functioning. JAMA 1963; 185:914–919Google Scholar
8 . Lawton MP, Brody EM: Assessment of older people: self-maintaining and instrumental activities of daily living. Gerontologist 1979; 9:179–186Google Scholar
9 . Kemp BJ, Mitchell JM: Functional assessment in geriatric mental health, in Handbook of Mental Health and Aging, 2nd ed. Edited by Birren JE, Sloane B, Cohen GD. New York, Academic Press, 1992, pp 671–697Google Scholar
10 . Fillenbaum GG: Screening the elderly: a brief instrumental activities of daily living measure. J Am Geriatr Soc 1985; 33:698–706Google Scholar
11 . Morris J, Morris S: ADL assessment measures for use with frail elders. J Ment Health Aging 1997; 3:19–45Google Scholar
12 . Spector WD, Fleishman JA: Combining activities of daily living with instrumental activities of daily living to measure functional disability. J Gerontol B Psychol Sci Soc Sci 1998; 53:S46–S57Google Scholar
13 . Asberg K, Sonn U: The cumulative structure of personal and instrumental ADL. Scand J Rehabil Med 1988; 21:171–177Google Scholar
14 . Spector WD, Katz S, Murphy JB, et al: The hierarchical relationship between activities of daily living and instrumental activities of daily living. J Chronic Dis 1987; 40:481–489Google Scholar
15 . Park DC: Psychological issues related to competence: cognitive aging and instrumental activities of daily living, in Societal Mechanisms for Maintaining Competence in Old Age. Edited by Willis SL, Schaie KW, Hayward M. New York, Springer, 1997, pp 66–80Google Scholar
16 . Ward G, Jagger C, Harper W: A review of instrumental ADL assessments for use in elderly people. Rev Clin Gerontol 1998; 8:65–71Google Scholar
17 . Royall DR, Chiodo LK, Polk MJ: Correlates of disability among elderly retirees with “sub-clinical” cognitive impairment. J Gerontol A Biol Sci Med Sci 2000; 55A:M541–546Google Scholar
18 . Petersen RC, Stevens JC, Ganguli M, et al: Practice parameter: early detection of dementia: mild cognitive impairment (an evidence based review): report of the quality standards subcommittee of the American Academy of Neurology. Neurology 2001; 56:1133–1142Google Scholar
19 . Royall DR, Chiodo LK, Polk, MJ: Missclassification is likely in the assessment of mild cognitive impairment. Neuroepidemiology 2004; 23:185–191Google Scholar
20 . Royall DR, Palmer R, Chiodo LK, et al: Executive control mediates memory’s association with change in functional status: the Freedom House study. J Am Geriatr Soc 2005; 53:11–17Google Scholar
21 . Royall DR, Mahurin RK, Gray K: Bedside assessment of executive dyscontrol: the executive interview (EXIT25). J Am Geriatr Soc 1992; 40:1221–1226Google Scholar
22 . Royall DR, Palmer R, Chiodo LK, et al: Declining executive control in normal aging predicts change in functional status: the Freedom House study. J Am Geriatr Soc 2004; 52:346–352Google Scholar
23 . Allen SC, Jain M, Ragab S, et al: Acquisition and short-term retention of inhaler techniques require intact executive function in elderly subjects. Age Ageing 2003; 32:299–302Google Scholar
24 . Royall DR, Mahurin RK, True J, et al: Executive impairment among the functionally dependent: comparisons between schizophrenic and elderly subjects. Am J Psychiatry 1993; 150:1813–1819Google Scholar
25 . Royall DR, Chiodo LK, Polk MJ: An empiric approach to level of care determinations using executive control measures. J Gerontol A Biol Sci Med Sci 2005; 60:1059–1064Google Scholar
26 . McCue M: The relationship between neuropsychology and functional assessment in the elderly, in Handbook of Neuropsychology and Aging. Edited by Nussbaum PD. New York, Plenum, 1997, pp 398–401Google Scholar
27 . Gallo JJ, Fulmer T, Paveza GJ, et al: Functional assessment, in Handbook of Geriatric Assessment, 3rd ed. Edited by Gallo JJ. Gaithersburg, Md, Aspen, 2000, pp 101–147Google Scholar
28 . Pearson VI: Assessment of function in older adults, in Assessing Older Persons. Edited by Kane RL, Kane RA. New York, Oxford University Press, 2000, pp 17–48Google Scholar
29 . Dorevitch MI, Cossar RM, Bailey FJ, et al: The accuracy of self and informant ratings of physical functional capacity in the elderly. J Clin Epedemiol 1992; 45:791–798Google Scholar
30 . Harper G: Assessing older adults who cannot communicate, in Assessing Older Persons. Edited by Kane RL, Kane RA. New York, Oxford University Press, 2000, pp 483–518Google Scholar
31 . Camicioli R, Moore MM, Sexton G, et al: Age-related brain changes associated with motor function in healthy older people. J Am Geriatr Soc 1999; 47:330–334Google Scholar
32 . Glass TA: Conjugating the “tenses” of function: discordance among hypothetical, experimental, and enacted function in older adults. Gerontologist 1998; 38:101–112Google Scholar
33 . Salthouse TA: Theories of cognition, in Handbook of Theories of Aging. Edited by Bengtson VL, Schaie KW. New York, Springer, 1999, pp 196–208Google Scholar
34 . Fried L, Tengen C, Walson J, et al: Frailty in older adults: evidence for a phenotype. J Gerontol A Biol Sci Med Sci 2001; 56:146–156Google Scholar
35 . Fried LP, Ferrucci L, Darer J, et al: Untangling the concepts of disability, frailty, and comorbidity: implications for improved targeting and care. J Gerontol A Biol Sci Med Sci 2004; 59:255–263Google Scholar
36 . Willis S, Allen-Burge R, Dolan MM, et al: Everyday problem solving among individuals with Alzheimer’s disease. Gerontologist 1998; 38:569–577Google Scholar
37 . Hayjek VE, Gagnon S, Ruderman JE: Cognitive and functional assessments of stroke patients: an analysis of their relation. Arch Phys Med Rehabil 1997; 78:1331–1337Google Scholar
38 . Provinciali L, Ceravolo MG, Bartolini M, et al: A multidimensional assessment of multiple sclerosis: relationships between disability domains. Acta Neurologica Scand 1999; 100:156–162Google Scholar
39 . Kachigan SK: Multivariate Satistical Analysis. New York, Radius Press, 1982Google Scholar
40 . Stevens J: Applied Multivariate Statistics for the Social Sciences, 2nd ed. Hillsdale, NJ, Lawrence Erlbaum Associates, 1992Google Scholar
41 . Mattis S: Dementia Rating Scale. Odessa, Fla, Psychological Assessment Resources, 1988Google Scholar
42 . Folstein M, Folstein S, McHugh PR: “Mini-mental state”: a practical method for grading the cognitive state of patients for the clinician. J Psychiatr Res 1975; 12:189–198Google Scholar
43 . Pfeiffer EA: Short portable mental status questionnaire for the assessment of organic brain deficit in elderly patients. J Am Geriatr Soc 1975; 23:433–441Google Scholar
44 . Baird A, Podell K, Lovell M, et al: Complex real-world functioning and neuropsychological test performance in older adults. Clin Neuropsychol 2001; 15:369–379Google Scholar
45 . Marson DC, Ingram KK, Cody HA, et al: Assessing the competency of patients with Alzheimer’s disease under different legal standards. Arch Neurol 1995; 52:949–954Google Scholar
46 . Marson DC, Chatterjee A, Ingram KK, et al: Toward a neurologic model of competency: cognitive predictors of capacity to consent in Alzheimer’s disease using three different legal standards. Neurology 1996; 46:666–672Google Scholar
47 . Royall DR, Cordes J, Polk MJ: Executive control and the comprehension of medical information by elderly retirees. Exp Aging Res 1997; 23:301–313Google Scholar
48 . Dymek MP, Atchison P, Harrell L, et al: Competency to consent to medical treatment in cognitively impaired patients with Parkinson’s. Neurology 2001; 56:17–24Google Scholar
49 . Marson DC, Cody HA, Ingram KK, et al: Neuropsychologic predictors of competency in Alzheimer’s disease using a rational reasons legal standard. Arch Neurol 1995; 52:955–959Google Scholar
50 . Boyle PA, Paul R, Moser D, et al: Cognitive and neurologic predictors of functional impairment in vascular dementia. Am J Geriatr Psychiatry 2003; 11:103–106Google Scholar
51 . Norton LE, Malloy PF, Salloway S: Impact of behavioral symptoms on activities of daily living in patients with dementia. Am J Geriatr Psychiatry 2001; 9:41–48Google Scholar
52 . Grace J, Stout J, Malloy P: Assessing frontal behavior syndromes with the frontal lobe personality scale. Assessment 1999; 6:269–284Google Scholar
53 . Royall DR, Chiodo LK, Polk MJ: Executive dyscontrol in normal aging: normative data, factor structure, and clinical correlates. Curr Neurol Neurosci Rep 2003; 3:487–493Google Scholar
54 . Royall DR, Palmer R, Chiodo LK, et al: Wisconsin Card Sort Performance fails to predict change in functional status in old age: the Freedom House study. J Clin Exp Neuropsychol, in press.Google Scholar
55 . Hebert LE, Scherr PA, Bennett DA, et al: Blood pressure and late-life cognitive function change: a biracial longitudinal population study. Neurology 2004; 62:2021–2024Google Scholar
56 . Ghisletta P, Lindenberger U: Static and dynamic longitudinal structural analyses of cognitive changes in old age. Gerontology 2004; 50:12–16Google Scholar
57 . Wilson RS, Li Y, Aggarwal NT, et al: Education and the course of cognitive decline in Alzheimer disease. Neurology 2004; 63:1198–1202Google Scholar
58 . Stuck AE, Waqlthert JM, Nikolaus T, et al: Risk factors for functional status decline in community-living older people: a systematic review of the literature. Soc Sci Med 1999; 48:445–449Google Scholar
59 . Artero S, Touchon J, Ritchie K: Disability and mild cognitive impairment: a longitudinal population-based study. Int J Geriatr Psychiatry 2002; 16:1092–1097Google Scholar
60 . Royall DR, Chiodo LK: Executive control and the validity of survey data. Int J Geriatr Psychiatry 2004; 19:969–968Google Scholar
61 . Royall DR, Palmer R, Mulroy A, et al: Pathological determinants of clinical dementia in Alzheimer’s disease. Exp Aging Res 2002; 28:143–162Google Scholar
62 . Loewenstein DA, Ownby R, Schram L, et al: An evaluation of the NINCDS-ADRDA neuropsychological criteria for assessment of Alzheimer’s disease: a confirmatory factor analysis of single versus multi-factor models. J Clin Exp Neuropsychol 2001; 23:274–284Google Scholar
63 . Ardila A, Pineda DA: Factor structure of non-verbal cognition. Int J Neurosci 2000; 104:125–144Google Scholar
64 . Royall DR: Executive cognitive function: a novel perspective on dementia. Neuroepidemiology 2000; 19:293–299Google Scholar
65 . Spector WD, Kemper P: Disability and cognitive impairment criteria: targeting those who need the most care. Gerontologist 1994; 34:640–651Google Scholar
66 . Ott BR, LaFleche G, Whelihan WM, et al: Impaired awareness of deficits in Alzheimer’s disease. Alzheimer Dis Assoc Disord 1996; 2:68–76Google Scholar
67 . Wadley VG, Harrell LE, Marson DC: Self- and informant report of financial abilities in patients with Alzheimer’s disease: reliable and valid? J Am Geriatr Soc 2003; 51:1621–1626Google Scholar
68 . Loewenstein DA, Amigo E, Duara R, et al: A new scale for the assessment of functional status in Alzheimer’s disease. J Gerontol B Psychol Sci Soc Sci 1989; 44:P114–121Google Scholar
69 . Ashley MJ, Persel CS, Clark MC: Validation of an independent living scale for post-acute rehabilitation applications. Brain Inj 2001; 15:435–442Google Scholar
70 . Mahurin RK, DeBettignies B, Pirozollo FJ: Structured assessment of independent living skills: preliminary report of a performance measure of functional abilities in dementia. J Gerontol B Psychol Sci Soc Sci 1991; 46:58–66Google Scholar
71 . Fan E, Royall DR, Chiodo LK, et al: Insight into financial capacity in non-institutionalized retirees. J Am Geriatr Soc 2003; 50:S80Google Scholar
72 . Petersen RC: Mild cognitive impairment: transition between aging and Alzheimer’s disease. Neurologia 2000; 15:93–101Google Scholar
73 . Nygård L: Instrumental activities of daily living: a stepping-stone towards Alzheimer’s disease diagnosis in subjects with mild cognitive impairment? Acta Neurologica Scand 2003; 107(suppl 179):42–46Google Scholar
74 . Palmer K, Fratiglioni L, Winlad B: What is mild cognitive impairment? variations in definitions and evolution of non-demented persons with cognitive impairment. Gerontologist 1998; 38:114–115Google Scholar
75 . Hughes CP, Berg L, Danziger WL, et al: A new clinical scale for the staging of dementia. Br J Psychiatry 1982; 140:566–572Google Scholar
76 . Albert SM, Michaels K, Padilla M, et al: Functional significance of mild cognitive impairment in elderly patients without a dementia diagnosis. Am J Geriatr Psychiatry 1999; 7:213–220Google Scholar
77 . Vitaliano PP, Breen AR, Albert MS: Memory attention, and functional status in community-residing Alzheimer type dementia patients and optimally healthy aged individuals. J Gerontol 1984; 39:58–54Google Scholar
78 . Mysiw JW, Beegan JG, Gatens PF: Prospective cognitive assessment of stroke patients before inpatient rehabilitation. Am J Phys Med Rehabil 1989; 68:168–171Google Scholar
79 . Ostwald SK, Snowdon DA, Rysavy DM, et al: Manual dexterity as a correlate of dependency in the elderly. J Am Geriatr Soc 1989; 37:963–969Google Scholar
80 . Reed B, Jagust WJ, Seab JP: Mental status as a predictor of daily function in progressive dementia. Gerontologist 1989; 29:804–807Google Scholar
81 . Kaye K, Grigsby J, Robbins LJ, et al: Prediction of independent functioning and behavior problems in geriatric patients. J Am Geriatr Soc 1990; 38:1304–1310Google Scholar
82 . McCue M, Rogers JC, Goldstein G: Relationships between neuropsychological and functional assessment in elderly psychiatric patients. Rehabil Psychol 1990; 35:91–99Google Scholar
83 . Jason Aronson MK, Post DC, Guastadisegni P: Dementia, agitation and care in the nursing home. J Am Geriatr Soc 1993; 41:507–512Google Scholar
84 . Fitzgerald JF, Smith DM, Martin DK, et al: Replication of the multidimensionality of activities of daily living. J Gerontology 1993; 48:S28–31Google Scholar
85 . Galski T, Bruno RL, Zorowitz R, et al: Predicting length of stay, functional outcome, and aftercare in the rehabilitation of stroke patients: the dominant role of higher-order cognition. Stroke 1993; 24:1794–1800Google Scholar
86 . Fitz AG, Teri L: Depression, cognition and functional ability in patients with Alzheimer’s disease. J Am Geriatr Soc 1994; 42:186–191Google Scholar
87 . Kaplan CP, Corrigan JD: The relationship between cognition and functional independence in adults with traumatic brain injury. Arch Physical Med Rehabil 1994; 75:643–647Google Scholar
88 . Rose L, Bakal DA, Fung TS, et al: Tactile extinction and functional status after stroke: a preliminary investigation. Stroke 1994; 25:1973–1976Google Scholar
89 . Seeman TE, Charpentier PA, Berkman LF, et al: Predicting changes in physical performance in a high-functioning elderly cohort: MacArthur studies of successful aging. J Gerontol A Biol Sci Med Sci 1994; 49:M97–108Google Scholar
90 . Seidel GK, Millis SR, Lichtenberg PA, et al: Predicting bowel and bladder continence from cognitive status in geriatric rehabilitation patients. Arch Phys Med Rehabil 1994; 75:590–593Google Scholar
91 . Diehl M, Willis SL, Schaie KW: Practical problem solving in older adults: obersvational assessment and cognitive correlates. Psychol Aging 1995; 10:478–491Google Scholar
92 . Hill RD, Bäckman L, Fratiglioni L: Determinants of functional abilities in dementia. J Am Geriatr Soc 1995; 43:1092–1097Google Scholar
93 . Loewenstein DA, Rubert MP, Argüelles T, et al: Neuropsychological test performance and prediction of functional capacities among Spanish-speaking and English-speaking patients with dementia. Arch Clin Neuropsychol 1995; 10:75–88Google Scholar
94 . Ford GR, Haley WE, Thrower SL, et al: Utility of Mini-Mental State Exam scores in predicting functional impairment among white and African American dementia patients. J Gerontol A Biol Sci Med Sci 1996; 51:M185–188Google Scholar
95 . Laukkanen P, Heikkinen E, Schroll M, et al: A comparative study of factors related to carrying out physical activities of daily living (PADL) among 75-year-old-men and women in two Nordic localities. Aging Clin Exp Res 1997; 9:258–267Google Scholar
96 . Velligan DI, Mahurin RK, Diamond PL, et al: The functional significance of sytmptomatology and cognitive function in schizophrenia. Schizophrenia Res 1997; 25:21–31Google Scholar
97 . Chen ST, Sultzer DL, Hinkin CH: Executive dysfunction in Alzheimer’s: association with neuropsychiatric symptoms and functional impairment. J Neuropsychiatry Clin Neurosci 1998; 10:426–432Google Scholar
98 . Grigsby J, Kaye K, Baxter J, et al: Executive cognitive abilities and functional status among community-dwelling older persons in the San Luis Valley health and aging study. J Am Geriatr Soc 1998; 46:590–596Google Scholar
99 . Rapport LJ, Hanks RA, Millis SR, et al: Executive functioning and predictors of falls in the rehabilitation setting. Arch Phys Med Rehabil 1998; 79:629–633Google Scholar
100 . Royall DR, Cabello M, Polk MJ: Executive dyscontrol: an important factor affecting the level of care received by elderly retirees. J Am Geriatr Soc 1998; 46:1519–1524Google Scholar
101 . Velligan DI, Bow-Thomas CC, Mahurin R, et al: Concurrent and predictive validity of the Allen Cognitive Levels Assessment. J Psychiatry Res 1998; 80:287–298Google Scholar
102 . Zanetti O, Frisoni GB, Rozzini L, et al: Validity of direct assessment of functional status as a tool for measuring Alzheimer’s disease severity. Age Ageing 1998; 27:615–622Google Scholar
103 . Binder EF, Storandt M, Birge SJ: The relation between psychometric test performance and physical performance in older adults. J Gerontol A Biol Sci Med Sci 1999; 54:M428–32Google Scholar
104 . Carlson MC, Fried LP, Xue QL, et al: Association between executive attention and physical functional performance in community-dwelling older women. J Gerontol B Psychol Sci Soc Sci 1999; 54:S262–270Google Scholar
105 . LaBuda J, Lichtenberg P: The role of cognition, depression, and awareness of deficit in predicting geriatric rehabilitation patients’ IADL performance. Clin Neuropsychol 1999; 13:258–267Google Scholar
106 . Ofstedal MB, Zimmer ZS, Lin HS: A comparison of correlates of cognitive functioning in older persons in Taiwan and the United States. J Gerontology B Psychol Sci Soc Sci 1999; 54:S291–301Google Scholar
107 . Poole JH, Ober BA, Shenaut GK, et al: Independent frontal system deficits in schizophrenia: cognitive, clinical, and adaptive implications. Psychiatry Res 1999; 85:161–176Google Scholar
108 . Solari A, Filippini G, Mendozzi L, et al: Validation of Italian multiple sclerosis quality of life (MSQOL)–54 questionnaire. J Neurol Neurosurg Psychiatry 1999; 67:158–162Google Scholar
109 . Cahn-Weiner DA, Malloy PF, Boyle PA, et al: Prediction of functional status from neuropsychological tests in community-dwelling elderly individuals. Clin Neuropsychol 2000; 14:187–195Google Scholar
110 . Kiosses DN, Alexopoulos GS, Murphy C: Symptoms of striatofrontal dysfunction contribute to disability in geriatric depression. Int J Geriatr Psychiatry 2000; 15:992–999Google Scholar
111 . Putzke JD, Williams MA, Daniel FJ, et al: Activities of daily living among heart transplant candidates: neuropsychological and cardiac function predictors. J Heart Lung Transplant 2000; 19:995–1006Google Scholar
112 . Velligan DI, Bow-Thomas CC, Mahurin R, et al: Do specific neurocognitive deficits predict specific domains of community function in schizophrenia? J Nerv Ment Dis 2000; 188:518–524Google Scholar
113 . Espiritu DA, Rashid H, Mast BT, et al: Depression, cognitive impairment and function in Alzheimer’s disease. Int J Geriatr Psychiatry 2001; 16:1098–1103Google Scholar
114 . Ito H, Tachimori H, Miyamoto Y, et al: Are the care levels of people with dementia correctly assessed for eligibility of the Japanese long-term care insurance? Int J Geriatr Psychiatry 2001; 16:1078–1084Google Scholar
115 . Bell-McGinty S, Podell K, Franzen M, et al: Standard measures of executive control in predicting instrumental activities of daily living in older adults. Int J Geriatr Psychiatry 2002; 17:828–834Google Scholar
116 . Beloosesky Y, Grinblat J, Epelboym B, et al: Functional gain of hip fracture patients in different cognitive and functional groups. Clin Rehabil 2002; 16:321–328Google Scholar
117 . Cahn-Weiner DA, Boyle PA, Malloy PF: Tests of executive function predict instrumental activities of daily living in community dwelling older individuals. Appl Neuropsychology 2002; 9:187–191Google Scholar
118 . Ertugrul A, Ulug B: The influence of neurocognitive deficits and symptoms on disability in schizophrenia. Acta Psychiatrica Scand 2002; 105:196–201Google Scholar
119 . Giovannetti T, Libon DJ, Buxbaum LJ, et al: Naturalistic action impairments in dementia. Neuropsychologia 2002; 40:1220–1232Google Scholar
120 . Malla AK, Norman RM, Manchanda R: Symptoms, cognition, treatment adherence and functional outcome in first-episode psychosis. Psychol Med 2002; 32:1109–1119Google Scholar
121 . Mayer SA, Kreiter KT, Copeland D, et al: Global and domain-specific cognitive impairment and outcome after subarachnoid hemorrhage. Neurology 2002; 59:1750–1758Google Scholar
122 . Pollice R, Roncone R, Falloon IR, et al: Is theory of mind in schizophrenia more strongly associated with clinical and social functioning than with neurocognitive deficits? Psychopathology 2002; 35:280–288Google Scholar
123 . Zawacki TM, Grace J, Paul R, et al: Behavioral problems as predictors of functional abilities of vascular dementia patients. J Neuropsychiatry Clin Neurosci 2002; 14:296–302Google Scholar
124 . Boyle PA, Malloy PF, Salloway S, et al: Executive dysfunction and apathy predict functional impairment in Alzheimer disease. Am J Geriatr Psychiatry 2003; 11:214–221Google Scholar
125 . Cahn-Weiner DA, Ready RE, Malloy PF: Neuropsychological predictors of everyday memory and everyday functioning in patients with mild Alzheimer’s disease. J Geriatr Psychiatry Neurol 2003; 16:84–89Google Scholar
126 . Gallo JJ, Rebok GW, Tennstedt S, et al: Linking depressive symptoms and functional disability in late life. Aging Ment Health 2003; 7:469–480Google Scholar
127 . McCall WV, Dunn AG: Cognitive deficits are associated with functional impairment in severely depressed patients. Psychiatry Res 2003; 121:179–184Google Scholar
128 . Tran M, Bedard M, Molloy DW, et al: Associations between psychotic symptoms dependence in activities of daily living among adults with Alzheimer’s disease. Int Psychogeriatr 2003; 15:171–179Google Scholar
129 . Mok VC, Wong A, Lam WW, et al: Cognitive impairment and functional outcome after stroke associated with small vessel disease. J Neurol Neurosurg Psychiatry 2004; 75:560–566Google Scholar
130 . Rapp MA, Beeri MS, Schmeidler JS, et al: Relationship of neuropsychological performance to functional status in nursing home residents and community dwelling older adults. Am J Geriatr Psychiatry 2005; 13:450–459Google Scholar