Construction of a Potential Telephone Assessment of Dementia Prevalence and Severity
Abstract
The “δ” (for “dementia”) is a latent dementia phenotype that can be constructed by a unique confirmatory bifactor model in a structural equation model framework. Because it is derived from Spearman’s general intelligence factor, “g,” δ can be constructed from any cognitive battery. This may allow for accurate dementia case-finding by telephone and in the absence of expert clinical evaluation or review. The authors constructed a new δ homolog in a large ethnically diverse convenience sample: the Texas Alzheimer’s Research and Care Consortium, comprising 2,016 participants (Alzheimer’s disease [AD], N=920; mild cognitive impairment, N=277; normal controls, N=819). A δ composite (“dTEL”) was extracted from informant-rated Instrumental Activities of Daily Living and a brief battery of verbal cognitive measures. The entire battery was engineered to be administered over the telephone. dTEL’s model had excellent fit. dTEL correlated strongly with dementia severity, as measured by the Clinical Dementia Rating “sum of boxes” scale (r=0.78, p<0.001). The dTEL composite’s area under the receiver operating characteristic curve for the discrimination between control subjects and AD patients was 0.97 (95% CI=0.964–0.975). This was superior to all dTEL indicators. Therefore, the authors have demonstrated that a δ homolog composite constructed entirely from verbal measures is strongly associated with dementia severity, can accurately diagnose dementia, and outperforms all observed measures from which it is constructed. Future studies are required to assess dTEL’s performance relative to evaluation by expert clinicians when obtained by lay psychometricians over the telephone.
“δ” (for “dementia”) is a latent phenotype of dementia itself, as distinct from impairment(s) in domain-specific cognitive performance. δ’s confirmatory bifactor model explicitly extracts the fraction of cognitive performance variance that is shared with one or more measures of functional status (i.e., in a structural equation model [SEM] framework). δ is relatively free of measurement error, continuously distributed, strongly related to Instrumental Activities of Daily Living (IADLs),1 agnostic to dementia’s etiology,2,3 and it can be modeled in almost any cognitive battery that also includes a measure of IADL. Thus, we further distinguish between δ (i.e., “the cognitive correlates of functional status”) and “d” (i.e., δ’s reification as a composite score in a specific cognitive battery or analysis).
δ’s bifactor model defines it as a subdivision of “general intelligence,” as operationalized by Spearman’s “g” factor (as opposed to observed “IQ” tests).4 Spearman showed that g is “indifferent to its indicators.” δ shares this property. It can be constructed from almost any ad hoc combination of cognitive and functional status measures (i.e., by confirmatory factor analysis in SEM). Each d composite is therefore likely to be calculated from a unique set of indicators. Nevertheless, they all estimate δ and are homologous to each other regardless of their indicators. We therefore refer to each d composite as a δ “homolog.” Because δ is “indifferent to its indicators,” δ homologs can be engineered to satisfy any agenda (e.g., brevity, availability in translation, administration via the Internet, etc.).
In the present article, we describe a new δ homolog, “dTEL,” derived from a brief cognitive battery that could be administered over the telephone by lay psychometricians. dTEL might allow for the accurate assessment of dementia severity, regardless of the patient’s location and in the absence of an experienced clinician.
Methods
Subjects
The methodology of the Texas Alzheimer’s Research and Care Consortium (TARCC) project has been described in detail elsewhere.5 Each participant underwent a standardized annual examination that included a medical evaluation, neuropsychological testing, and clinical interview. Diagnosis of Alzheimer’s disease (AD) status was based on National Institute of Neurological Communicative Disorders and Stroke-Alzheimer’s Disease and Related Disorders Association criteria.6 Control subjects performed within normal limits on their psychometric assessments. Institutional review board approval was obtained at each site, and written, informed consent was obtained from all participants.
dTEL’s Cognitive Indicators
Executive control function.
Categorical animal fluency (animal):7 Animal fluency assesses the spontaneous production of words restricted to a semantic category. In TARCC, a subject is asked to name as many animals as possible within an interval of one minute.
Memory.
Logical Memory I and II:8 The subject is asked to recall two paragraphs read aloud, immediately and following a 30-minute delay. Delayed paragraph recall has been useful clinically in identifying dementia and tracking progression of the disease.
dTEL’s “Target” Indicator
Instrumental Activities of Daily Living.
IADLs were assessed using informant ratings.1 The ability to use the telephone, go shopping, prepare food, perform housekeeping and laundry, use transportation, handle finances, and be responsible for medication adherence were each rated on a Likert scale ranging from 0 (no impairment) to 3 (specific incapacity).
Clinical Variables
The Clinical Dementia Rating Scale “sum of boxes” (CDR-SOB):9 The CDR-SOB is used to evaluate dementia severity. The rating assesses the patient’s cognitive ability to function in six domains: memory, orientation, judgment and problem solving, community affairs, home and hobbies, and personal care. Information is collected during an interview with the patient’s caregiver. Optimal CDR-SOB ranges corresponding to global CDR scores are 0.5–4.0, for a global score of 0.5; 4.5–9.0, for a global score of 1.0; 9.5–15.5, for a global score of 2.0; and 16.0–18.0, for a global score of 3.0.10
The Mini-Mental State Examination11 is a well-known and widely used test for screening cognitive impairment.12 Scores range from 0 to 30. Scores less than 24 reflect cognitive impairment.
The Geriatric Depression Scale (GDS): Depressive symptoms were assessed using the short GDS.13,14 GDS scores range from 0 to 30. Higher scores indicate worse performance. A threshold of 9–10 best differentiates clinically depressed from nondepressed elderly individuals.
Statistical Analyses
This analysis was performed by using Analysis of Moment Structures software.15 A latent δ homolog was constructed by confirmatory bifactor analysis in a SEM framework. The latent dTEL homolog was adjusted for age, education, ethnicity, and gender. The homolog’s factor determinacy was tested by using Grice’s method.16 The homolog was validated by its correlation with clinician-rated dementia severity, as measured by the CDR-SOB.
The dTEL homolog was then reified as a composite dTEL score. The composite dTEL score was validated by receiver operating characteristic (ROC) analysis (i.e., AD case subjects compared with normal control subjects [NCs]) and compared to each of its indicators’ age, education, ethnicity, and gender-adjusted ROC performance (i.e., by using DeLong’s17 method with MedCalc software).18
Missing data.
Only the ROC analyses were limited to complete cases. Elsewhere, we used full-information maximum likelihood (FIML) methods to address missing data. FIML uses the entire observed data matrix to estimate parameters with missing data. In contrast to listwise or pairwise deletion, FIML yields unbiased parameter estimates, preserves the overall power of the analysis, and is arguably superior to alternative methods (e.g., multiple imputation).19,20
Fit indices.
The validity of structural models was assessed by using two common test statistics. A nonsignificant chi-square signifies that the data are consistent with the model.21 The comparative fit index (CFI), with values ranging between 0 and 1, compares the specified model with a model of no change.22 CFI values below 0.95 suggest model mis-specification. Values of 0.95 or greater indicate adequate-to-excellent fit. A root mean-square error of approximation (RMSEA) of 0.05 or less indicates a close fit to the data, with models below 0.05 considered a “good” fit and up to 0.08 considered “acceptable.”23All three fit statistics should be simultaneously considered to assess the adequacy of the models to the data.
ROC curves.
The diagnostic performance or accuracy of a test to discriminate non-healthy from normal subjects can be evaluated by using ROC curve analysis.24,25 Briefly, the true positive rate (sensitivity) is plotted as a function of the false positive rate (100% specificity) for different cut-off points of a parameter. Each point on the ROC curve represents a sensitivity/specificity pairing corresponding to a particular decision threshold. The area under the ROC curve is a measure of how well a parameter can distinguish between two diagnostic groups (non-healthy and normal). The analysis was performed with the Statistical Package for the Social Sciences.26
Results
Descriptive statistics are presented in Table 1. dTEL’s adjusted bifactor model (Figure 1) had excellent fit (minimum χ2=60.92; p<0.001; RMSEA=0.076; CFI=1.00). The dTEL factor weights are summarized in Table 2. All dTEL’s cognitive indicators loaded significantly, ranging from Logical Memory I (r=−0.93, p<0.001) to animal naming (r=−0.53, p<0.001). Additionally, IADL loaded strongly (r=0.59). All g’ indicators loaded significantly on that construct (Table 2).
Variable | TARCC Total | Normal Controls | Alzheimer’s Disease | |||
---|---|---|---|---|---|---|
N | Mean (SD) | N | Mean (SD) | N | Mean (SD) | |
Age (years) | 3,381 | 70.88 (9.48) | 1,384 | 66.48 (9.05) | 1,240 | 75.47 (8.36) |
APOE e4 allelesb | 3,154 | 0.46 (0.62) | 1,332 | 0.24 (0.42) | 1,180 | 0.60 (0.49) |
CDR (sum of boxes) | 3,306 | 2.42 (3.35) | 1,383 | 0.01 (0.07) | 1,235 | 5.78 (3.31) |
Animals | 3,385 | 14.67 (5.15) | 1,384 | 17.73 (4.40) | 1,240 | 11.19 (3.84) |
Education | 3,381 | 13.24 (4.25) | 1,384 | 12.86 (4.65) | 1,240 | 14.00 (3.72) |
Ethnicityc | 3,381 | 0.36 (0.47) | 1,384 | 0.51 (0.50) | 1,239 | 0.14 (0.35) |
GDS-30 (observed) | 3,005 | 5.60 (5.25) | 1,317 | 4.54 (4.79) | 1,005 | 6.06 (5.16) |
Genderd | 3,312 | 0.39 (0.49) | 1,384 | 0.32 (0.47) | 1,240 | 0.44 (0.50) |
IADL (summed) | 3,381 | 10.48 (4.52) | 1,384 | 7.80 (1.16) | 1,240 | 14.44 (5.16) |
MMSE | 3,311 | 25.52 (4.76) | 1,384 | 28.54 (1.90) | 1,240 | 21.47 (4.94) |
WMS LM-II | 3,381 | 8.05 (4.30) | 1,384 | 11.44 (3.00) | 1,240 | 4.21 (2.51) |
WMS LM-I | 3,385 | 7.78 (3.95) | 1,384 | 10.61 (3.24) | 1,240 | 4.59 (2.56) |
Complete cases | 2,861 |
TABLE 1. Descriptive Characteristicsa
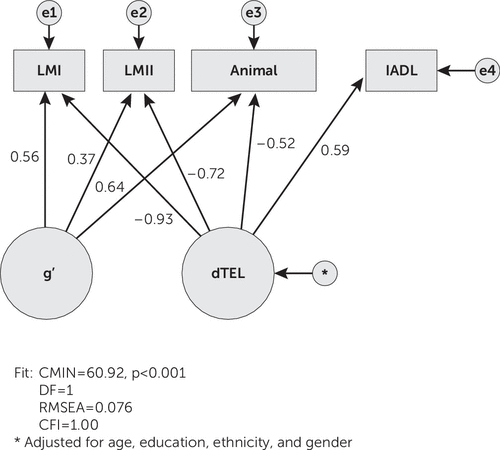
FIGURE 1. dTEL Modela
a Animal=animal naming; IADL=Instrumental Activities of Daily Living; RMSEA=root mean square error of association; WMS LM-I=Wechsler Memory Scale Logical Memory I-immediate; WMS LM-II=Wechsler Memory Scale Logical Memory II-delayed. Asterisks indicates that dTEL is adjusted for age, education, ethnicity, and gender.
Item | Factor | β | S.E. | Beta | p |
---|---|---|---|---|---|
Measure | |||||
g’ Parameter | |||||
Animal naming | g’ | 3.28 | 0.22 | 0.64 | <0.001 |
WMS LM-I | g’ | 2.21 | 0.18 | 0.56 | <0.001 |
WMS LM-II | g’ | 1.56 | 0.25 | 0.38 | <0.001 |
dTEL Parameter | |||||
Animal naming | dTEL | –1.02 | 0.10 | –0.53 | <0.001 |
WMS LM-I | dTEL | –1.08 | 0.08 | –0.72 | <0.001 |
WMS LM-II | dTEL | –1.49 | 0.09 | –0.93 | <0.001 |
IADL | dTEL | 1.00 | 0.59 | ||
Fit indices | |||||
χ2 | 60.92 (df=1) | ||||
CMIN | 60.92 (p<0.001) | ||||
CFI | 1.0 | ||||
RMSEA | 0.076 |
TABLE 2. dTEL and g’ Parameters in the Texas Alzheimer’s Research and Care Consortium Cohort (All Participants)a
The adjusted dTEL factor correlated strongly with CDR-SOB (r=0.78, p<0.001). Determinacy was acceptable (multiple R=0.94; total item-squared multiple correlation=0.89; minimum correlation=0.78), and dTEL was reified as a composite dEQ score. The adjusted composite’s area under the curve (AUC) for the discrimination between the NC group and the AD group was 0.97 (95% confidence interval [CI]=0.964–0.975) (Table 3). This was superior to all of dTEL’s indicators (all p values <0.05) (Table 3) (Figure 2).
Item | Area Under the Curve | 95% CI |
---|---|---|
dTEL | 0.97 | 0.964–0.975 |
Animal naming | 0.82 | 0.808–0.839 |
LM-I | 0.89 | 0.876–0.900 |
LM-II | 0.91 | 0.901–0.922 |
IADL | 0.83 | 0.815–0.848 |
TABLE 3. Receiver Operating Characteristic Curve Analysis of dTEL and its Indicators as Predictors of Normal Cases Compared With Alzheimer’s Disease Casesa
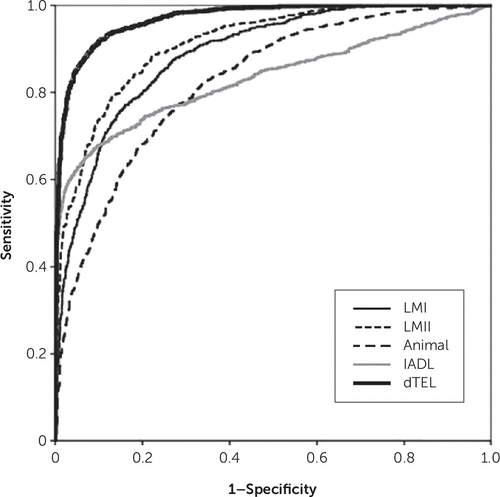
FIGURE 2. Receiver Operating Characteristic Curvesa
a Animal=animal naming; IADL=Instrumental Activities of Daily Living; ROC=receiver operating characteristic; WMS LM-I=Wechsler Memory Scale Logical Memory I-immediate; WMS LM-II=Wechsler Memory Scale Logical Memory II-delayed. Asterisk indicates that dTEL and its indicators are adjusted for age, education, ethnicity, and gender.
Discussion
In this analysis, the latent variable δ was constructed from a set of verbal cognitive measures. Two features of this model are remarkable. First, to our knowledge, this is the twelfth δ homolog constructed to date. Regardless of their indicators, all have exhibited strong associations with IADL and/or CDR scores, as well as high AUCs for the discrimination between AD patients and control subjects.2,27–33 Second, the indicators for this model could be easily obtained over the phone, possibly by paraprofessional psychometricians and/or mid-level care providers.
dTEL’s AUC was higher than those of any of its observed indicators. This helps justify the administration of dTEL’s battery relative to any single dTEL indicator. This finding supports our claim that d homologs necessarily perform “greater than the sum of their parts,” regardless of whether they are constructed from comprehensive psychometric batteries,28,30 brief screening batteries (as in this example), or the item sets of individual measures.28,31 Similarly, the AUC we achieved here is superior to those reported for other telephone-administered psychometric measures.34–37
We previously validated a brief d homolog constructed from the 5-item Telephone Executive Assessment Scale (TEXAS).31,38 The dTEXAS homolog had an AUC of 0.92 (CI=0.880–0.968) for the discrimination between NCs and AD patients, superior to the entire 25-item Executive Interview,39 from which the TEXAS is derived.31 While both dTEL and dTEXAS might be administered over the phone, dTEXAS’s ease of administration must be weighed against its significantly poorer diagnostic accuracy.
Like dTEL, dTEXAS correlated strongly with IADL (r=−0.861, p<0.001).31 Either composite can be constructed from its cognitive indicators alone and used to predict IADL (i.e., δ’s “target” indicator). The use of such “restricted” composites frees d homologs from the need for an intact patient-caregiver dyad. dTEXAS’s restricted composite correlated with IADL (r=−0.51, p<0.001). A restricted dTEL composite might also be deployed among subjects without informants.
δ recasts dementia as “the cognitive correlates of functional status.” Our approach converts dementia from a category to a continuous dimension. The dementia severity of any two persons can thereby be compared, even persons performing within the normal range on δ’s indicators. Even small changes in the d-scores of persons without dementia appear to increase the risk of prospective conversion to clinical dementia.29 By using this method, the progress toward dementia in a patient without dementia can be followed over time, or in response to intervention.
dTEL’s validity outside of clinical AD has yet to be determined. Although TARCC purports to be a study of “AD,” an estimated 20% of patients diagnosed with clinical “AD” by experienced clinicians may be without beta-amyloid as determined with positron emission tomography.40,41
Moreover, δ homologs appear to be “agnostic” to the etiology of dementia. Two recent studies (by an independent group of investigators) confirm that δ is agnostic to dementia’s etiology. Both were conducted with the National Alzheimer’s Coordinating Center’s Unified Dataset (N=26,000). Gavett et al.2 found δ’s AUC to be 0.96 for the discrimination between individuals with dementia of any etiology (i.e., AD, Lewy body disease, fronto-temporal dementia, and “vascular dementia”) and individuals without dementia (patients with mild cognitive impairment and control subjects). δ also confirms the disabling and therefore inherently “dementing” cognitive effects of depression.42 John et al.3 demonstrated that δ scores are unable to distinguish between any combination of dementing conditions. These findings suggest that clinical dementia arises from the sum of independent δ-related processes, regardless of etiology.
Since δ is essentially the sole cognitive determinant of dementia severity, both cross-sectionally (e.g., r=0.99 versus the CDR-SOB) and longitudinally (e.g., r=0.94 × ΔCDR-SOB),2,43 it seems likely that an optimal dTEL diagnostic threshold could be applied to a wide range of potentially dementing conditions (e.g., traumatic brain injury). Whether this can be accomplished over the telephone by lay psychometricians awaits demonstration.
The advantages of dTEL may be incrementally superior to that of its alternatives. Results similar to ours have been achieved by other measures. Manly et al.44 reported the diagnostic accuracy of telephone screening with the Telephone Interview for Cognitive Status (TICS)45 and the Dementia Questionnaire (DQ)46 in a large, ethnically diverse community sample (33.7% Hispanic, i.e., similar to TARCC). The TICS demonstrated an AUC of 0.94 for discrimination between participants with and without dementia (sensitivity=0.88, specificity=0.87). The DQ did less well (i.e., AUC=0.84, sensitivity=0.66, and specificity=0.89, respectively). In the Alzheimer’s Disease Anti-inflammatory Prevention Trial,47 the TICS group did not perform as well (i.e., sensitivity=0.73 and specificity=0.95). Instead, it took a telephone assessment battery, composed of five measures, including the TICS, to achieve a comparable diagnostic accuracy (i.e., sensitivity=0.83 and specificity=0.82 for the diagnosis of dementia). That battery’s administrative burden would be similar to that of dTEL.
Regardless, any of the above assessments might be reengineered as δ homologs and thereby improve upon their diagnostic accuracy. The diagnostic accuracy, demonstrated by multiple δ homologs to date, suggests that a wide range of δ homologs might be engineered to meet the needs of a wide range of users and in a wide range of settings. δ homologs might be engineered for their brevity, low cost, availability in translation, or mode of administration (e.g., telephone, computer, website, etc.). Dementia might then be diagnosed far from memory clinics, without expert adjudication and by a larger range of professionals.
In summary, the dTEL homolog achieves a very high AUC for discrimination between AD patients and control subjects. It offers the potential convenience of administration by telephone. dTEL, or similar δ homologs, might allow high-quality dementia diagnoses to be made in minority or rural populations, far from tertiary care centers and without expert adjudication.
1 : Assessment of older people: self-maintaining and instrumental activities of daily living. Gerontologist 1969; 9:179–186Crossref, Medline, Google Scholar
2 : The δ latent dementia phenotype in the uniform data set: cross-validation and extension. Neuropsychology 2015; 29:344–352Crossref, Medline, Google Scholar
3 : The effectiveness and unique contribution of neuropsychological tests and the δ latent phenotype in the differential diagnosis of dementia in the uniform data set. Neuropsychology 2016; 30:946–960Crossref, Medline, Google Scholar
4 : Human Ability. London, Macmillan, 1951Google Scholar
5 : The Texas Alzheimer’s Research Consortium longitudinal research cohort: study design and baseline characteristics. Texas Public Health Journal 2008; 60:9–13Google Scholar
6 : Clinical diagnosis of Alzheimer’s disease: report of the NINCDS-ADRDA Work Group under the auspices of Department of Health and Human Services Task Force on Alzheimer’s Disease. Neurology 1984; 34:939–944Crossref, Medline, Google Scholar
7 : The Consortium to Establish a Registry for Alzheimer’s disease (CERAD), part 1: clinical and neuropsychological assessment of Alzheimer’s disease. Neurology 1989; 39:1159–1165Crossref, Medline, Google Scholar
8 : Wechsler Memory Scale, 3rd ed. San Antonio, Tex, Psychological Corporation, 1997Google Scholar
9 : A new clinical scale for the staging of dementia. Br J Psychiatry 1982; 140:566–572Crossref, Medline, Google Scholar
10 : Staging dementia using Clinical Dementia Rating Scale Sum of Boxes scores: a Texas Alzheimer’s research consortium study. Arch Neurol 2008; 65:1091–1095Crossref, Medline, Google Scholar
11 : “Mini-Mental State”: a practical method for grading the cognitive state of patients for the clinician. J Psychiatr Res 1975; 12:189–198Crossref, Medline, Google Scholar
12 : The Mini-Mental State Examination: a comprehensive review. J Am Geriatr Soc 1992; 40:922–935Crossref, Medline, Google Scholar
13 : Geriatric Depression Scale (GDS): recent evidence and development of a shorter version. Clin Gerontol 1986; 5:165–173Crossref, Google Scholar
14 : A comparison of two depression scales in a geriatric assessment clinic. Am J Geriatr Psychiatry 1995; 3:60–67Crossref, Medline, Google Scholar
15 Arbuckle JL: Analysis of Moment Structures-AMOS (Version 7.0). Chicago, SPSS, 2006Google Scholar
16 : Computing and evaluation factor scores. Psychol Methods 2001; 6:430–450Crossref, Medline, Google Scholar
17 : Comparing the areas under two or more correlated receiver operating characteristic curves: a nonparametric approach. Biometrics 1988; 44:837–845Crossref, Medline, Google Scholar
18 MedCalc Statistical Software: htto://www.medcalc.orgGoogle Scholar
19 : Missing data: our view of the state of the art. Psychol Methods 2002; 7:147–177Crossref, Medline, Google Scholar
20 : Missing data analysis: making it work in the real world. Annu Rev Psychol 2009; 60:549–576Crossref, Medline, Google Scholar
21 : Testing Structural Equation Model. Thousand Oaks, Calif, Sage Publications, 1993Google Scholar
22 : Comparative fit indexes in structural models. Psychol Bull 1990; 107:238–246Crossref, Medline, Google Scholar
23 : Alternative ways of assessing model fit, in Testing Structural Equation Models. Edited by Bollen KA, Long JS. Thousand Oaks, Calif, Sage Publications, 1993, pp 136–162Google Scholar
24 : Basic principles of ROC analysis. Semin Nucl Med 1978; 8:283–298Crossref, Medline, Google Scholar
25 : Receiver-operating characteristic (ROC) plots: a fundamental evaluation tool in clinical medicine. Clin Chem 1993; 39:561–577Medline, Google Scholar
26 : 18, Release Version 18.0.0. Chicago, SPSS, 2009, pp 27Google Scholar
27 ; Validation of a latent variable representing the dementing process. J Alzheimers Dis 2012; 30:639–649Crossref, Medline, Google Scholar
28 : The latent dementia phenotype δ is associated with cerebrospinal fluid biomarkers of Alzheimer’s disease and predicts conversion to dementia in subjects with mild cognitive impairment. J Alzheimers Dis 2016; 49:547–560Crossref, Medline, Google Scholar
29 : δ scores predict MCI and AD conversions from non-demented states. Alzheimers Dement (Amst) 2017; 6:214–221Medline, Google Scholar
30 : Greater than the sum of its parts: δ Improves upon a battery's diagnostic performance. Neuropsychology 2015; 29:683–692Crossref, Medline, Google Scholar
31 : Greater than the sum of its parts: δ can be constructed from item level data. J Alzheimers Dis 2016; 49:571–579Crossref, Medline, Google Scholar
32 : Getting past “g”: testing a new model of dementing processes in persons without dementia. J Neuropsychiatry Clin Neurosci 2012; 24:37–46Link, Google Scholar
33 : Validation of a latent construct for dementia in a population-wide dataset from Singapore. J Alzheimers Dis 2017; 55:823–833Crossref, Medline, Google Scholar
34 : Alzheimer’s disease in the NAS-NRC Registry of Aging Twin Veterans, IV: performance characteristics of a two-stage telephone screening procedure for Alzheimer’s dementia. Psychol Med 1995; 25:1211–1219Crossref, Medline, Google Scholar
35 : Screening for dementia by telephone using the memory impairment screen. J Am Geriatr Soc 2003; 51:1382–1390Crossref, Medline, Google Scholar
36 : Validation study of a French version of the Modified Telephone Interview for Cognitive Status (F-TICS-m) in elderly women. Int J Geriatr Psychiatry 2010; 25:1142–1149Crossref, Medline, Google Scholar
37 : Montreal Cognitive Assessment 5-minute protocol is a brief, valid, reliable, and feasible cognitive screen for telephone administration. Stroke 2015; 46:1059–1064Crossref, Medline, Google Scholar
38 : Telephone screening for mild cognitive impairment in Hispanics using the Alzheimer’s Questionnaire. Exp Aging Res 2014; 40:129–139Crossref, Medline, Google Scholar
39 : Bedside assessment of executive cognitive impairment: the Executive Interview (EXIT). J Am Geriatr Soc 1992; 40:1221–1226Crossref, Medline, Google Scholar
40 : Association between clinical measures and florbetapir F18 PET neuroimaging in mild or moderate Alzheimer’s disease dementia. J Neuropsychiatry Clin Neurosci 2014; 26:214–220Link, Google Scholar
41 : Florbetapir F18 PET amyloid neuroimaging and characteristics in patients with mild and moderate Alzheimer dementia. Psychosomatics 2016; 57:208–216Crossref, Medline, Google Scholar
42 : The default mode network may be the key substrate of depression-related cognitive changes. J Alzheimers Dis 2013; 34:547–560Crossref, Medline, Google Scholar
43 : Future dementia severity is almost entirely explained by the latent variable δ’s intercept and slope. J Alzheimers Dis 2016; 49:521–529Crossref, Medline, Google Scholar
44 : Telephone-based identification of mild cognitive impairment and dementia in a multicultural cohort. Arch Neurol 2011; 68:607–614Crossref, Medline, Google Scholar
45 : The Telephone Interview for Cognitive Status. Neuropsychiatry Neuropsychol Behav Neurol 1988; 1:111–117Google Scholar
46 : A validation study of the Dementia Questionnaire. Arch Neurol 1994; 51:901–906Crossref, Medline, Google Scholar
47 : Screening by telephone in the Alzheimer’s disease anti-inflammatory prevention trial. J Alzheimers Dis 2013; 36:433–443Crossref, Medline, Google Scholar