Man Versus Machine: Comparison of Radiologists’ Interpretations and NeuroQuant® Volumetric Analyses of Brain MRIs in Patients With Traumatic Brain Injury
Abstract
NeuroQuant® is a recently developed, FDA-approved software program for measuring brain MRI volume in clinical settings. The purpose of this study was to compare NeuroQuant with the radiologist’s traditional approach, based on visual inspection, in 20 outpatients with mild or moderate traumatic brain injury (TBI). Each MRI was analyzed with NeuroQuant, and the resulting volumetric analyses were compared with the attending radiologist’s interpretation. The radiologist’s traditional approach found atrophy in 10.0% of patients; NeuroQuant found atrophy in 50.0% of patients. NeuroQuant was more sensitive for detecting brain atrophy than the traditional radiologist’s approach.
Decades of research have shown that traumatic brain injury (TBI) causes brain atrophy.1,2 In recent years, with advances in technology, there has been a shift from qualitative descriptions to measurement of the volume abnormalities. Despite the advances in the research settings, MRI brain volumetry generally was not available in routine clinical practice.
This situation changed in 2007 with the introduction of NeuroQuant®, a computer-automated method for measuring brain MRI volume (http://www.cortechs.net/products/neuroquant.php). The U.S. Food and Drug Administration (FDA) approved NeuroQuant for the routine clinical measurement of brain MRI volume in human subjects.
In addition to the evidence supplied in the FDA application, several peer-reviewed studies have supported the reliability and validity of NeuroQuant for measuring brain volume in neuropsychiatric patients and normal-control subjects.3–7 These included one previous peer-reviewed study in which NeuroQuant detected hippocampal atrophy in a patient with mild TBI.3 Otherwise, there are no peer-reviewed published data regarding NeuroQuant’s application to patients with TBI. More generally, little is known about the relationship between the results of NeuroQuant analyses and the radiologist’s traditional interpretation, which is based solely on visual inspection.
The purpose of this study was to explore these areas and test the hypothesis that, in comparison with the radiologist’s traditional approach, NeuroQuant would be more sensitive for detecting atrophy in patients with TBI.
Methods
Subjects
Patients
Included in this study were outpatients consecutively admitted to the Virginia Institute of Neuropsychiatry who met the selection criteria. Selection criteria required that each patient 1) was diagnosed with traumatic brain injury by a board-certified neuropsychiatrist (DER) according to the criteria of Menon et al;8 2) had a mild-or-moderate level of brain injury according to the criteria of Rao and Lyketsos;9 3) agreed to be in the study and signed the informed consent form; 4) had no contraindications to obtaining an MRI, such as having magnetic metal in the head or being pregnant; 5) had an MRI without artifacts (such as motion artifacts) which would preclude accurate identification of brain structures by the NeuroQuant software. Also, each patient was matched with a normal-control to have a similar level of education (within 3 years), in order to minimize the potentially confounding effect of education on brain volume; three patients were excluded using this method because they had very low levels of education. This study was approved by the New England Institutional Review Board and satisfied the requirements of the Code of Ethics of the World Medical Association (Declaration of Helsinki) for human research.
Twenty patients met the selection criteria; 19 had mild TBI, and 1 had moderate TBI. Demographic characteristics were as follows: 8 men and 12 women; mean age (years): 46.2 (SD: 13.9; range: 19.9–66.2); mean number of years of education was 14.6 (SD: 2.6; range: 11–19).
Normal-control subjects
The NeuroQuant computer-automated analysis routinely provides volume data on 11 brain regions, left and right sides, for a total of 22 volume measurements (http://www.cortechs.net/products/neuroquant.php).4 However, it provides comparisons to a normal-control group for only three brain regions (averaged across left and right sides). In order to assess NeuroQuant’s ability to detect atrophy in all 22 brain regions, this study used a group of normal-controls different from the NeuroQuant normal-controls. For these extended analyses, normal-control data were obtained from a larger group previously studied as part of the Alzheimer’s Disease Neuroimaging Initiative (ADNI).10–12 The ADNI normal-control data were made publicly available (http://adni.loni.ucla.edu).
The ADNI was launched in 2003 by the National Institute on Aging (NIA), the National Institute of Biomedical Imaging and Bioengineering (NIBIB), the Food and Drug Administration (FDA), private pharmaceutical companies, and nonprofit organizations, as a $60 million, 5-year public/private partnership. The primary goal of ADNI has been to test whether serial magnetic resonance imaging (MRI), positron emission tomography (PET), other biological markers, and clinical and neuropsychological assessment can be combined to measure the progression of mild cognitive impairment (MCI) and early Alzheimer's disease (AD). The Principal Investigator of this initiative is Michael W. Weiner, M.D., VA Medical Center and University of California–San Francisco. ADNI is the result of efforts of many co-investigators from a broad range of academic institutions and private corporations, and subjects have been recruited from over 50 sites across the United States and Canada.
For the NeuroQuant extended analyses reported herein, a subgroup of 20 normal-control subjects (10 men, 10 women) were chosen from the ADNI database. The mean age was 68.3 years (SD: 3.6 years; range: 60.0–71.5), and the mean number of years of education was 16.0 (SD 3.1; range: 9–20).
The groups of patients and ADNI normal-controls did not differ significantly with respect to sex (chi square likelihood ratio: 0.19, df=1,35; NS). In order to compare the two groups with respect to age, a nonparametric test (Wilcoxon rank-sum test) was chosen because the normal-control group’s data were not normally distributed. The two groups differed significantly with respect to age (χ2=25.7; p <0.01), with ADNI normal-controls older than the patient group. The two groups did not differ significantly with respect to years of education (independent t-test, t=1.55, df=1,38; NS).
Brain Imaging
Magnetic resonance imaging
Each patient had a 3.0-tesla MRI of the brain performed at one of three local radiology centers, using the scanning protocol recommended for allowing later NeuroQuant analysis; this protocol is described in detail on the NeuroQuant website (http://www.cortechs.net/products/neuroquant.php) and was the same protocol used for the ADNI subjects. In addition to the general requirements for having an MRI (e.g., having no magnetic metal in the head), the NeuroQuant protocol required, at a minimum, the following:
•. | Supported MRI scanner (GE, Siemens or Phillips) | ||||
•. | MRI scanning protocol based on the ADNI scanning protocol | ||||
•. | T1 timing sequence | ||||
•. | Noncontrast | ||||
•. | Sagittal acquisition | ||||
•. | 3D inverse Fourier-transform scanning protocol | ||||
•. | Scan included nose, ears, and vertex without wraparound |
As part of the standard clinical procedure at the Virginia Institute of Neuropsychiatry and nearby radiology centers, several other MRI sequences were obtained on each patient in order to allow a thorough evaluation of the effects of traumatic brain injury on brain structure. Accordingly, in addition to the above “NeuroQuantable” MRI, each MRI evaluated by the radiologists included the following sequences: 1) T1, 3D, saggital, noncontrast (which also was used for the NeuroQuant-based volumetric analyses); 2) coronal T2 sequence; 3) axial FLAIR sequence; 4) susceptibility-weighted imaging (SWI, preferably) or gradient-recall echo (if SWI unavailable); and 4) diffusion tensor imaging.
NeuroQuant automated brain MRI segmentation
The brain MRI data for each patient or ADNI normal-control was uploaded to the NeuroQuant server, which processed and analyzed the brain-imaging data. This computer-automated analysis involved several steps, including stripping the brain of scalp, skull, and meninges; inflating the brain to a spherical shape; mapping the spherical brain to a common spherical space shared with the Talairach Atlas brain;13 identification of brain segments (that is, regions); and deflation of the patient’s brain back to its original shape while retaining the identifying information for brain segments. The output of the NeuroQuant computer-automated analysis included a report containing volumetric information, and a set of DICOM-formatted brain images that were segmented, with each region identified by a distinctive color.
Segmentation Errors
The NeuroQuant segmented DICOM images were inspected for errors, a step recommended by the makers of NeuroQuant in order to ensure accurate identification of brain regions by the software. The left and right counterparts for each of the 11 brain regions were segmented. Therefore, for each subject, there were 22 brain regions segmented. The segmentation results for each region were visually inspected by two of the authors (DER and ALO). The table shows the results of inspection for errors. For determining rates of errors, there were 20 patients, with each brain region measured on right and left sides, for a total of 40 measurements per brain region. The mean rate of inaccurate identification across regions was 10.2% (SD: 11.1%; range: 0.0%–32.5%). Examples of segmentation errors are shown in Figure 1.
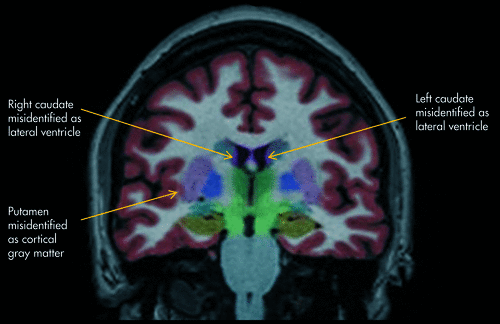
This segmented MRI image created by NeuroQuant shows three examples of brain regions being misidentified as adjacent brain regions.
NeuroQuant Analysis
Each brain region volume was corrected for interindividual differences in head size by dividing by intracranial volume, with the result being expressed as a percentage. The result was compared with the normal-control data, and the patient’s brain region was classified as abnormally small if it fell below the 5th normative percentile.
For the ADNI normal-controls, the results from the NeuroQuant standard analysis were used to determine means and standard deviations (SDs) for each of the 11 brain regions (left and right sides analyzed separately). Each patient’s data were compared with the data from the normal-controls in order to calculate z-scores, which were converted to normative percentile ranks. Results were considered to be consistent with (although not probative of) parenchymal atrophy if they met one of the following criteria: 1) a parenchymal region ≤5th normative percentile; or 2) a ventricular region ≥95th normative percentile, consistent with atrophy of the surrounding parenchyma.
Radiologist’s Traditional Interpretation
For each patient, the MRI was interpreted by one of five local, board-certified radiologists on the basis of simple visual inspection, per the usual clinical practice. The radiologists were blind to the NeuroQuant results. The attending radiologist’s interpretation was examined to determine whether atrophy or ventricular enlargement had been noted.
Statistical Analysis
A two-tailed paired sign test was used to test the hypothesis that the NeuroQuant findings differed from the radiologist’s interpretations. JMP software was used to perform the statistical analysis.
Results
A two-tailed paired sign test showed that a significantly higher percentage of patients had atrophy identified by the Neuroquant extended analysis (10 of 20 patients; 50.0%) than by the radiologist’s traditional method of visual inspection (2 of 20 patients; 10.0%) (test statistic M, two-tailed = −4.00; p=0.02; Figure 2).
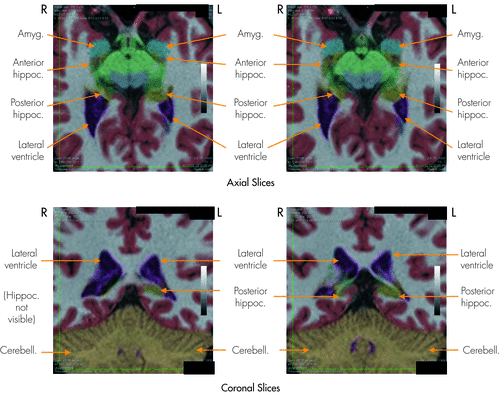
The results of the NeuroQuant analysis showed that the right hippocampus was abnormally small, with a volume of 0.18% of intracranial volume (<0.1 normative percentile). The NeuroQuant-generated segmented images above show that the right hippocampus was abnormally small, especially in its posterior segment, and smaller than its left-sided counterpart. Adjacent to the right hippocampus, the right lateral ventricle appeared larger than its left-sided counterpart, possibly due to right hippocampal atrophy leading to right lateral ventricle ex vacuo enlargement. The attending radiologist interpreted the associated gray-scale MRI scan (upon which this NeuroQuant analysis was based) as showing normal sizes of the hippocampi and lateral ventricles.
R: right; L: left; Amyg: amygdala; Hippoc: hippocampus; Cerebell: cerebellum.
Discussion
Main Findings
The main finding of this study was that NeuroQuant was more sensitive for detecting brain atrophy on MRI than the method of visual inspection traditionally used by radiologists.
The NeuroQuant software approach was a practical and useful approach for measuring brain MRI volume in patients with traumatic brain injury. The authors found that it was important to follow exactly the guidelines for collecting MRIs recommended by the manufacturer of NeuroQuant and summarized in the Methods section above. After uploading the MRI images to the NeuroQuant server, it took about 20 minutes for the computer-automated software to analyze the brain images and report volumetric data. This rate of analysis was a vast improvement over the older method based on a human operator’s determining regions of interest; even with partial computer assistance, the older method often took many hours to complete volumetric analysis for one subject.
NeuroQuant identified most of the brain regions accurately, but there was a fair rate of segmentation errors (mean of 10.2%; range: 0%–32.5%) detected by visual inspection of the NeuroQuant segmented images (Table 1). The authors agree with the manufacturer of NeuroQuant that the results of the brain segmentation always should be verified by visual inspection to ensure valid results. Nevertheless, since the large majority of structures were identified accurately by NeuroQuant, the computer-automated approach remained useful.
Segmentation Errors | ||
---|---|---|
N | % | |
Forebrain parenchyma | 0/40 | 0.0 |
Cortical gray matter | 3/40 | 7.5 |
Lateral ventricles | 6/40 | 15.0 |
Inferior lateral ventricle | 0/40 | 0.0 |
Hippocampus | 1/40 | 2.5 |
Amygdala | 0/40 | 0.0 |
Caudate | 11/40 | 27.5 |
Putamen | 13/40 | 32.5 |
Pallidum | 2/40 | 5.0 |
Thalamus | 5/40 | 12.5 |
Cerebellum | 4/40 | 10.0 |
Mean | 4.1/40 | 10.2 |
The radiologist’s traditional approach found at least one sign of atrophy in 10.0% of patients; in contrast, NeuroQuant found at least one sign of atrophy in 50.0% of patients. This difference was statistically significant, indicating that similar results probably would be found in other settings involving patients with mild-or-moderate traumatic brain injury.
The finding of a 10.0% rate of atrophy detected by the radiologists was consistent with many years of clinical experience in which the brain MRIs of most patients with mild-or-moderate traumatic brain injury were read as normal or as having nonspecific findings. Lacking objective findings of brain injury on the MRI, most of these patients had little-to-no other objective findings of injury. Often, patients reported that the existence of their injury was viewed with skepticism by others, including defense experts, employers, or, sometimes, even family members.
The finding of a 50.0% rate of atrophy by NeuroQuant suggested that the use of such computer-automated techniques will make it much more common in the near future to find abnormal brain structure in patients with mild-or-moderate TBI. Previously, a question was raised about the effect on patients of learning about the results of brain structural analysis (comments made by Jonathan Silver, M.D. at the annual meeting of the American Neuropsychiatric Association; Denver, CO, March 2011). In our clinic, it is customary for the attending neuropsychiatrist (DER) to review the brain images and volumetric results with each patient. Our clinical experience suggests that patients are interested in knowing if they have abnormal brain structure that may be important for understanding their brain injury or neuropsychiatric symptoms. Each case needs to be evaluated individually. In cases in which it seemed likely that the atrophy was caused by the traumatic brain injury, patients rarely expressed distress at learning about the results (“I already knew something was wrong with me.”) and, in fact, often feel vindicated or even relieved by such evidence (“Now other people will know it, too.”). In some cases, being aware of brain structural abnormalities led to greater compliance with recommended treatment (“I guess I’ll have to wear that CPAP mask now.”)
Study Limitations
Although brain atrophy commonly is caused by TBI, it is not always true that atrophy was caused by the TBI in a given patient. Patients with persistent symptoms from TBI (like the patients in this study) often have pre-accident neurological or psychiatric disorders that can cause abnormal brain structure. The longitudinal design (obtaining serial MRIs) is more powerful than the cross-sectional design for determining whether TBI causes brain atrophy,14 and it is recommended for studies of groups or individual patients when possible. For example, in a case in which the brain atrophied markedly over the several-week period after the TBI, it may be more likely that the atrophy was caused by the TBI than by a pre-accident problem such as alcohol abuse in remission.
In comparison with the group of patients, the ADNI normal-controls used in this study for the NeuroQuant extended analyses were significantly older. It is well known that increasing age, especially being over 50 years old, is associated with brain atrophy.15–17 Because the ADNI normal-controls were older than the patients in the current study, it was quite possible that the patients had a higher rate of atrophy than was revealed by the NeuroQuant analyses. Therefore, this was a conservative limitation. It was unlikely that NeuroQuant would have found atrophy in the patients when it did not actually exist, or that the results would have been biased in favor of NeuroQuant finding more atrophy than that found by the radiologists, who knew each patient’s age and could factor that information into their decision about presence of atrophy.
Similarly, the radiologists had a potential advantage over Neuroquant (which was based only on a T1 MRI sequence) because they had a greater range and amount of brain imaging data available (including T2, FLAIR, etc., in addition to the T1 sequence). Therefore, the results could have been biased in the favor of the radiologists’ finding atrophy. The fact that the results actually showed the opposite make it somewhat more remarkable that NeuroQuant was more sensitive for detecting atrophy than was the traditional approach used by the radiologists.
In the current study, radiologists’ interpretations were based on simple visual inspection, not qualitative ratings. It is true that qualitative ratings are a useful approach for evaluating MRI brain atrophy; see, for example, Victoroff et al (1994).18 Furthermore, it seems likely that such an approach combined with comparison of ratings between observers would improve upon the traditional approach. However, we chose not to utilize that approach in the current study because our goal was not to compare NeuroQuant to the best possible method based upon visual inspection. In contrast, our goal was to compare NeuroQuant to standard radiological practice. In our clinical experience, despite the period of at least the last 18 years during which such approaches have been available, we have never seen an MRI report on a patient that used a qualitative rating scale to assess level of atrophy or ventricular enlargement. With the rapid advances in computer-based technology, instead of focusing on understanding and developing the approach based on qualitative ratings, it may be more advantageous to focus on the computer-automated approaches.
This study was limited to patients with mild-or-moderate TBI. It may be less advantageous to use NeuroQuant in patients with severe TBI for the following reasons: 1) it is more likely that the radiologist would note signs of atrophy; and 2) cranial or brain structural abnormalities may be more severe and therefore more likely to cause problems with the NeuroQuant software algorithms, which expect the head and brain to appear close to a predefined normal. Nevertheless, this topic deserves further study.
Conclusions
NeuroQuant was a practical and useful technique for measuring brain MRI volume in typical clinical settings. It was more sensitive for finding brain atrophy than the traditional radiologist’s approach. However, the radiologist’s approach is better for finding nonvolume-related abnormalities. Therefore, the two approaches are complementary.
1 Bigler ED: Structural Imaging. Edited by Silver JM, McAllister TW, Yudofsky SC. Washington, DC, American Psychiatric Publishing, Inc., 2005, pp 79–105Google Scholar
2 Bigler ED: Structural Imaging. Edited by Silver JM, McAllister TW, Yudofsky SC. Washington, DC, American Psychiatric Publishing, Inc., 2011, pp 73–90Google Scholar
3 : NeuroQuant® revealed hippocampal atrophy in a patient with traumatic brain injury. J Neuropsychiatry Clin Neurosci 2012; 24:E33Link, Google Scholar
4 : Fully-automated volumetric MRI with normative ranges: translation to clinical practice. Behav Neurol 2009; 21:21–28Crossref, Medline, Google Scholar
5 : Fully-automated quantification of regional brain volumes for improved detection of focal atrophy in Alzheimer disease. AJNR Am J Neuroradiol 2009; 30:578–580Crossref, Medline, Google Scholar
6 : High-throughput, fully automated volumetry for prediction of MMSE and CDR decline in mild cognitive impairment. Alzheimer Dis Assoc Disord 2009; 23:139–145Crossref, Medline, Google Scholar
7 : Intra- and interscanner variability of automated voxel-based volumetry based on a 3D probabilistic atlas of human cerebral structures. Neuroimage 2010; 49:2216–2224Crossref, Medline, Google Scholar
8 : Position Statement: Definition of Traumatic Brain Injury. Arch Phys Med Rehabil 2010; 91:1637–1640Crossref, Medline, Google Scholar
9 : Neuropsychiatric sequelae of traumatic brain injury. Psychosomatics 2000; 41:95–103Crossref, Medline, Google Scholar
10 Jack CR Jr, Bernstein MA, Fox NC, et al: The Alzheimer's Disease Neuroimaging Initiative (ADNI): MRI methods, J Magn Reson Imaging 2008; 27:685–691Google Scholar
11 : Alzheimer’s Disease Neuroimaging Initiative (ADNI): clinical characterization. Neurology 2010; 74:201–209Crossref, Medline, Google Scholar
12 : The Alzheimer’s disease neuroimaging initiative: progress report and future plans. Alzheimers Dement 2010; 6:202–211, e7Crossref, Medline, Google Scholar
13 Talairach J, Tournoux P: Co-Planar Stereotaxic Atlas of the Human Brain: An Approach to Cerebral Imaging. Edited by Schuenke, Schulte, Schumacher, et al. Thieme, Germany, Thieme Publishers, 1988Google Scholar
14 : Review of longitudinal studies of MRI brain volumetry in patients with traumatic brain injury. Brain Inj 2011; 25:1271–1278Crossref, Medline, Google Scholar
15 : [Brain volumetric MRI study in healthy elderly persons using statistical parametric mapping]. Seishin Shinkeigaku Zasshi 2004; 106:138–151Medline, Google Scholar
16 : Brain aging and its modifiers: insights from in-vivo neuromorphometry and susceptibility-weighted imaging. Ann N Y Acad Sci 2007; 1097:84–93Crossref, Medline, Google Scholar
17 : Trajectories of brain aging in middle-aged and older adults: regional and individual differences. Neuroimage 2010; 51:501–511Crossref, Medline, Google Scholar
18 : A method to improve interrater reliability of visual inspection of brain MRI scans in dementia. Neurology 1994; 44:2267–2276Crossref, Medline, Google Scholar