Seizure Control and Memory Impairment Are Related to Disrupted Brain Functional Integration in Temporal Lobe Epilepsy
Abstract
Brain functional integration can be disrupted in patients with temporal lobe epilepsy (TLE), but the clinical relevance of this disruption is not completely understood. The authors hypothesized that disrupted functional integration over brain regions remote from, as well as adjacent to, the seizure focus could be related to clinical severity in terms of seizure control and memory impairment. Using resting-state functional MRI data acquired from 48 TLE patients and 45 healthy controls, the authors mapped functional brain networks and assessed changes in a network parameter of brain functional integration, efficiency, to examine the distribution of disrupted functional integration within and between brain regions. The authors assessed whether the extent of altered efficiency was influenced by seizure control status and whether the degree of altered efficiency was associated with the severity of memory impairment. Alterations in the efficiency were observed primarily near the subcortical region ipsilateral to the seizure focus in TLE patients. The extent of regional involvement was greater in patients with poor seizure control: it reached the frontal, temporal, occipital, and insular cortices in TLE patients with poor seizure control, whereas it was limited to the limbic and parietal cortices in TLE patients with good seizure control. Furthermore, TLE patients with poor seizure control experienced more severe memory impairment, and this was associated with lower efficiency in the brain regions with altered efficiency. These findings indicate that the distribution of disrupted brain functional integration is clinically relevant, as it is associated with seizure control status and comorbid memory impairment.
Epilepsy is considered a network-level disease involving abnormal connectivity in brain circuits, which is responsible for the initiation and evolution of seizures.1,2 As both the ictal and interictal states share some common features of abnormal connectivity,3 interictal abnormalities could be informative of clinical characteristics of seizures and, furthermore, comorbid cognitive dysfunction4,5 in epilepsy.
Application of functional MRI (fMRI) in the ictal period is constrained by practical limitations: it is impossible to predict when seizures will occur except in cases of special epileptic syndromes that can be deliberately induced, such as musicogenic epilepsy.6 As an alternative, resting-state fMRI (rsfMRI) has been used simultaneously with electroencephalography (EEG) in the interictal period7 to infer causality related to seizure initiation and propagation.8 However, such a combined approach is not generally available or technically easily feasible, thus making it worthwhile to assess whether rsfMRI alone can be used to investigate clinically relevant aspects of epilepsy.9
Cumulative works using rsfMRI have revealed altered or abnormal connectivity in the interictal state. In temporal lobe epilepsy (TLE), abnormalities in brain regions in the seizure focus were revealed with seed-based time series correlation10,11 and independent component analysis.12 Although these findings provide evidence that abnormalities can be present at the network level beyond the seizure focus in the interictal state of TLE, whether the distribution of abnormalities is related to clinical factors such as seizure control and cognitive dysfunction has not been thoroughly examined.
Graph-theoretical analysis has been used successfully to identify abnormalities of network configuration in patients with various neuropsychiatric diseases.13–15 In TLE, changes in brain functional networks in the interictal state have been characterized as disruption to functional integration over the whole brain.16–18 In the present study, we investigated the distribution of interictal abnormalities in TLE patients in terms of alterations in functional integration at the regional level, as well as hemispheric and whole-brain levels, to elucidate the clinical relevance of disrupted brain functional integration. We hypothesized that the extent of disrupted brain functional integration could be related to seizure control status and/or comorbid cognitive dysfunction, specifically memory impairment.
Methods
Subjects
The study subjects were 48 patients with TLE (mean age: 41.4 years [±12.6 years], 31 females) and 45 age- and gender-matched healthy controls (mean age: 41.2 years [±13.5 years], 23 females). Epilepsy was diagnosed clinically and based on detailed laboratory investigations, including long-term video EEG monitoring, structural and functional brain imaging, and neuropsychological evaluations. The clinical diagnosis of TLE was made according to criteria of the International League Against Epilepsy.19 Lateralization of the seizure focus was determined in the traditional manner by assessing seizure semiology, epileptiform discharges on ictal or interictal EEG, and hippocampal sclerosis or atrophy on structural MRI. Mean age at epilepsy onset was 24.6 years (±13.0 years), and the duration of epilepsy was 17.0 years (±12.7 years). Among the 48 TLE patients, 26 patients were determined to have left TLE (LTLE), and the other 22 patients had right TLE (RTLE). All healthy controls had no history of neurological disorders and no abnormalities on structural MRI. Written, informed consent was obtained from all participants in accordance with the Declaration of Helsinki and its later amendments, and the study protocol was approved by the Ethical Committee of Ewha Medical Center, Seoul, Korea.
Assessment
Seizure control status was assessed based on the Engel classification.20 Each patient was assigned to one of four classes: class I, seizure free or auras only; class II, no more than twice per year; class III, no more than once per month; and class IV, more than once per month. Based on this classification, we divided the TLE patients into two subgroups: 1) a good seizure control (TLEGSC) group consisting of the class I and II patients and 2) a poor seizure control (TLEPSC) group consisting of the class III and IV patients.
Neuropsychological tests were performed to assess verbal memory with a Korean version of the California Verbal Learning Test (K-CVLT)21 and nonverbal memory with the Rey-Osterrieth Complex Figure Test (ROCFT).22 For each test, immediate recall (IR), delayed recall (DR), and recognition (R) scores were recorded and adjusted for age and education level.
Acquisition of MRI Data
MRI data were acquired with a 3 Tesla Achieva system (Philips, Best, the Netherlands). For each subject, 150 volumes of rsfMRI data were obtained with blood-oxygen-level-dependent (BOLD) contrast in the axial plane using a T2*-weighted gradient echo echo-planar imaging sequence: repetition time=2,000 ms, echo time=32 ms, voxel size=1.67 mm×1.67 mm×5.00 mm, matrix size=144×144, and slice number=32. During scanning, subjects were instructed to close their eyes and remain as motionless as possible. Structural MRI data were obtained in the coronal plane using a three-dimensional sensitivity encoding sequence: voxel size=0.50 mm×0.50 mm×1.00 mm, matrix size=448×448, and slice number=160.
Preprocessing of rsfMRI Data
Preprocessing of rsfMRI data were performed using the routines in SPM8 (http://www.fil.ion.ucl.ac.uk/spm/) and DPARSF (http://rfmri.org/DPARSF/). The preprocessing steps were performed in the order of spatial realignment for correcting for head movement, normalization into a coordinate frame matching that of a template brain conforming to the Montreal Neurological Institute space, and spatial smoothing with a Gaussian kernel of 4-mm full width at half maximum. Transformation parameters for spatial normalization were derived from segmentation of the high-resolution structural image coregistered to the mean functional image.
In addition, linear trends mainly due to systematic increases or decreases in signal were removed, and nuisance covariates including six head movement parameters estimated during spatial realignment and cerebrospinal fluid and white matter signals were regressed out to reduce the effects of head motion and nonneuronal fluctuations. The functional images were then band-pass filtered at 0.01 Hz–0.08 Hz to reduce the effects of very low- and high-frequency physiological noise.
Construction of Brain Functional Networks
A brain functional network was constructed by defining nodes and estimating edges between them. Nodes were defined in 90 brain parcellations of the whole-brain gray matter determined by the automated anatomical labeling brain atlas.23 The list of the 45 brain parcellations in each hemisphere is presented in Table S1 in the data supplement accompanying the online version of this article. Following the procedure in SPM8, the principal or first eigenvariate from singular value decomposition of the voxel signals within each brain parcellation was assigned as the representative signal of each node. Edges were estimated by calculating the Pearson’s correlation-based functional connectivity between the representative signals of the 90 nodes.
Functional connectivity between all pairs of the 90 nodes composed a 90×90 matrix of correlation coefficients, which was then converted to adjacency matrices with a range of sparsity by applying a set of threshold values. Each adjacency matrix represented a brain functional network with unweighted and undirected edges in such a way that 1s corresponded to presence of edges, and 0s corresponded to absence of edges. To increase the number of patients in the subgroups defined by seizure control status, RTLE and LTLE patients were grouped together by flipping the functional brain networks of RTLE patients at the midsagittal plane such that the affected hemisphere (AH) was placed on the left for all TLE patients.
Calculation of Average Efficiency
We wanted to reveal abnormalities in terms of disrupted functional integration in the interictal state of TLE patients. We employed the network parameter “efficiency” (for details, see the Methods section in the online data supplement) to assess the functional integration of brain networks.
The efficiency can be calculated for each pair of nodes in a functional brain network. For any subnetwork composed of nodes, the average efficiency is calculated as the average of efficiency for every pair of nodes in the subnetwork.
We calculated the average efficiency at hierarchical spatial levels (see Figure S1 and Table S2 in the online data supplement): at the whole-brain level, the hemispheric level, and the regional level. In particular, we were interested in searching for the extent of altered average efficiency at the regional level. We therefore specified 296 subnetworks according to 12 brain regions in each hemisphere: central (Cnt), lateral frontal (FrnL), medial frontal (FrnM), orbital frontal (FrnO), temporal (Tmp), lateral parietal (PrtL), medial parietal (PrtM), lateral occipital (OccL), medial occipital (OccM), limbic (Lmb), insular (Ins), and subcortical (Sbc) regions.
Comparison of Average Efficiency
Abnormalities of brain functional networks in epilepsy were revealed by comparing average efficiency between healthy controls and TLE patients at each spatial level. Cost-integrated measures of average efficiency (see the Methods section in the online data supplement) were compared using two-sample t tests for each subnetwork. At the whole-brain level, we checked whether the average efficiency was altered in TLE patients compared with healthy controls and whether such changes were dependent on seizure control status. That is, we conducted a comparison between healthy controls and all TLE patients and then compared the healthy control group with the TLEGSC and TLEPSC subgroups. At the hemispheric and regional levels, we examined which subnetworks contributed to the whole-brain changes.
Furthermore, to examine how seizure lateralization might influence topological changes according to seizure control status, we checked whether seizure lateralization (LTLE and RTLE) interacted with seizure control status (GSC and PSC) by conducting two-way analysis of variance. In the inferences, statistical significance was determined with the p value set at 0.05, corrected for multiple comparisons at each spatial level by following the rough false discovery rate procedure.
Relationship Between Average Efficiency and Memory Performance
The association between average efficiency values and scores on individual measures of the K-CVLT and ROCFT was assessed using Spearman’s rank correlation at each spatial level. We were interested in whether the extent of altered average efficiency involved memory impairment. Thus, we sought correlations within the mask of group differences in the average efficiency, only for subnetworks in which the average efficiency was altered in TLE patients compared with healthy controls. Statistical significance was determined with the p value set at 0.05, corrected for multiple comparisons at each spatial level.
Results
Classification of Patients
Of the 48 TLE patients, 33 were assessed as having GSC and the other 15 were assessed as having PSC. Characteristics of individual TLE patients are presented in Table S3 in the online data supplement, and summarized characteristics of the two subgroups are presented in Table 1. Age at epilepsy onset and duration of epilepsy were not different between the two subgroups. When comparing memory performance between the two subgroups, TLEPSC patients experienced more severe memory impairment than TLEGSC patients as measured by the ROCFT. Specifically, differences were observed in ROCFT-IR scores (t(46)=1.8829, p=0.0330) and ROCFT-DR scores (t(46)=2.5754, p=0.0066).
Characteristic | TLEGSC | TLEPSC | TLEGSC versus TLEPSC | |
---|---|---|---|---|
Age (years) | 42.61±13.70 | 38.67±9.42 | NS | |
Age at onset (years) | 26.24±13.72 | 20.87±10.70 | NS | |
Duration (years) | 16.64±12.82 | 17.80±12.98 | NS | |
M:F | 13:20 | 4:11 | NS | |
LTLE:RTLE | 17:16 | 9:6 | NS | |
K-CVLT | ||||
IR (%) | 40.53±26.30 | 32.93±29.35 | NS | |
DR (%) | 40.20±27.61 | 32.83±29.47 | NS | |
R (%) | 51.05±24.82 | 43.90±28.73 | NS | |
ROCFT | ||||
IR (%) | 41.72±33.96 | 22.53±29.70 | TLEGSC >TLEPSC | |
DR (%) | 41.98±31.76 | 18.09±24.68 | TLEGSC >TLEPSC | |
R (%) | 29.90±27.64 | 20.01±28.42 | NS |
TABLE 1. Clinical Characteristics of Temporal Lobe Epilepsy (TLE) Patients According to Seizure Control Statusa
Comparison of Average Efficiency: Healthy Controls Versus TLE Patients
At the whole-brain level, the average efficiency was reduced in TLE patients compared with healthy controls (t(91)=2.0318, p=0.0451) (Figure 1A), and at the hemispheric level, the average efficiency between hemispheres was reduced in TLE patients compared with healthy controls (t(91)=2.1993, p=0.0304) (Figure 1B). At the regional level, the average efficiency was altered in both hemispheres, primarily for subnetworks near the subcortical region of the AH (AH Sbc) in TLE patients compared with healthy controls (Figure 1C).
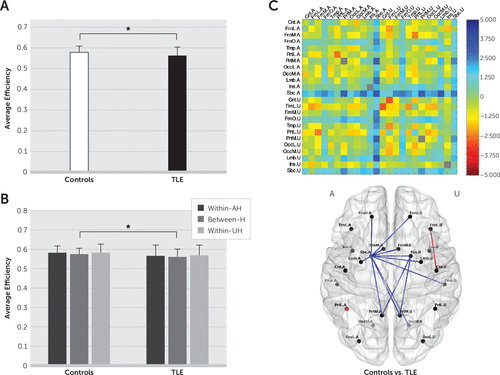
FIGURE 1. Comparison of the Average Efficiency Between Healthy Controls and Temporal Lobe Epilepsy (TLE) Patientsa
a The average efficiency (A) at the whole-brain level, (B) at the hemispheric level, and (C) at the regional level is shown. In (A) and (B), error bars represent standard deviations, and asterisks indicate statistical significance. In (C), the upper panel depicts a map of t values acquired from two-sample t tests, and the lower panel shows subnetworks with statistically significant differences, colored in red for increases and in blue for decreases in the average efficiency in TLE patients relative to healthy controls. The region labels are as presented in Table S1 in the online data supplement. AH, affected hemisphere; Between-H, between hemispheres; UH, unaffected hemisphere; Within-AH, within the affected hemisphere; Within-UH, within the unaffected hemisphere.
Comparison of Average Efficiency: Healthy Controls Versus TLEGSC and TLEPSC Patients
At the whole-brain level, a reduction in the average efficiency compared with healthy controls was observed in TLEPSC patients (t(58)=2.9798, p=0.0042), but not in TLEGSC patients (Figure 2A). In comparing at the hemispheric level, the whole-brain changes in TLEPSC patients could be attributed to reductions in the average efficiency within the AH (t(58)=2.6574, p=0.0102) and between hemispheres (t(58)=3.0662, p=0.0033) (Figure 2B).
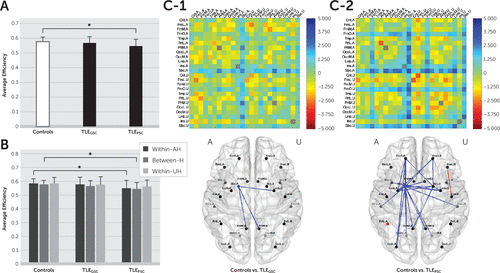
FIGURE 2. Comparison of the Average Efficiency Between Healthy Controls and Temporal Lobe Epilepsy Patients With Good (TLEGSC) or Poor (TLEPSC) Seizure Controla
a The average efficiency (A) at the whole-brain level, (B) at the hemispheric level, and (C) at the regional level are shown. In (A) and (B), error bars represent standard deviations, and asterisks indicate statistical significance. In (C), the upper panels depict maps of t values acquired from two-sample t tests, and the lower panels show subnetworks with statistically significant differences, colored in red for increases and in blue for decreases in the average efficiency in TLEGSC (C–1) and TLEPSC (C–2) patients relative to healthy controls. The region labels are as presented in Table S1 in the online data supplement. AH, affected hemisphere; Between-H, between hemispheres; UH, unaffected hemisphere; Within-AH, within the affected hemisphere; Within-UH, within the unaffected hemisphere.
At the regional level, an obvious contrast between TLEGSC and TLEPSC subgroups was observed in the extent of alterations in the average efficiency. Abnormalities were observed commonly near the AH Sbc in both subgroups. However, the extent of abnormalities was greater in TLEPSC patients, reaching the frontal, temporal, occipital, and insular cortices. In contrast, abnormalities were relatively restricted to the limbic and parietal cortices in TLEGSC patients (Figure 2C and 2D). A full list of subnetworks that were identified to be abnormal is provided in Table S4 in the online data supplement.
Interaction Between lateralization and Seizure Control Status
At the whole-brain and hemispheric levels, no interaction between seizure lateralization (LTLE and RTLE) and seizure control status (GSC and PSC) was observed for any subnetwork. Additionally, no interaction was seen for any subnetwork at the regional level.
Relationship Between Altered Average Efficiency and Memory Impairment
At the whole-brain and hemispheric levels, correlations between the average efficiency and memory impairment were not observed in TLE patients or in the subgroups of TLEGSC and TLEPSC patients. At the regional level, the average efficiency within the AH Sbc correlated positively with ROCFT-IR scores in TLE patients (Figure 3A). Correlations between the average efficiency and memory impairment were not observed for any subnetwork in TLEGSC patients, but correlations between the average efficiency and ROCFT measures were observed for some of the subnetworks with abnormalities in TLEPSC patients (Figure 3B and 3C). The average efficiency correlated positively with ROCFT-IR and ROCFT-DR scores particularly in subnetworks near the AH Sbc in TLEPSC patients. A full list of correlations is provided in Table S5 in the online data supplement.
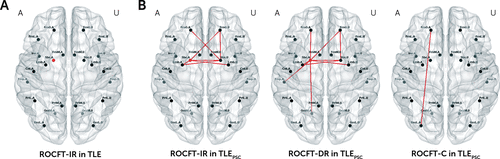
FIGURE 3. Correlation of the Average Efficiency With Memory Performance in Temporal Lobe Epilepsy (TLE) Patientsa
a Correlations between Rey-Osterrieth Complex Figure Test (ROCFT) scores and the average efficiency at the regional level are shown as follows: (A) immediate recall (IR) scores in all TLE patients, and (B) IR, delayed recall (DR), and recognition (R) scores in TLE patients with poor seizure control (TLEPSC). Dots and lines represent subnetworks specified within and between regions, respectively, and red indicates positive relationships. The region labels are as presented in Table S1 in the online data supplement. AH, affected hemisphere; UH, unaffected hemisphere.
Discussion
In this study, we used rsfMRI to examine the distribution of interictal abnormalities in TLE patients, as measured by alterations in functional integration at the regional level, as well as the hemispheric and whole-brain levels. We demonstrated that 1) brain functional integration was disrupted in TLE patients, more extensively at the regional level in TLEPSC patients than in TLEGSC patients, and 2) there was an association between greater reduction in brain functional integration and more severe memory impairment in regional subnetworks with abnormalities in TLEPSC patients.
Graph theoretical analysis allowed the characterization of topological changes in brain functional networks in TLE and provided evidence that TLE is a disorder of abnormal brain networks, rather than a focal epileptogenic abnormality. Our finding of reduced average efficiency at the whole-brain level is in agreement with previous observations from graph theoretical analysis of fMRI or electrophysiological data in TLE,16–18 which included observations of increased average shortest path length or decreased average efficiency over the whole brain. Such disruption to brain functional integration may be related to a shift in connection topology to a regularized pattern16,17 or to an accompanying disruption to functional segregation18 in TLE.
We further demonstrated that topological changes in functional brain networks were dependent on clinical severity as reflected by seizure control status. In TLEPSC patients, but not in TLEGSC patients, a reduction in the average efficiency was exhibited at the whole-brain level, which could be attributed to declines in the average efficiency within the AH and between hemispheres at the hemispheric level. For TLE patients included in this study, the ratio of GSC to PSC was not statistically different between LTLE and RTLE patients. Thus, the topological changes in functional brain networks seemed independent of seizure lateralization (see Figure S2 in the online data supplement).
By tracking down sub-brain contributions at the regional level, we revealed the distribution of abnormalities in terms of disrupted functional integration within and between regions. All TLE patients had disruption of functional integration near the AH Sbc, but regional involvement was diffuse in TLEPSC patients, reaching the frontal, temporal, occipital, and insular cortices, whereas disrupted functional integration was restricted to the limbic and parietal cortices in TLEGSC patients. Between the two subgroups with GSC and PSC, the difference in the extent of interictal abnormalities at the regional level seems to have determined whether topological alterations were seen or not at the hemispheric level and further at the whole-brain level. TLE is often related to reorganization of extratemporal regions, as well as mesial temporal structures adjacent to the epileptogenic zone.24 More frequent seizures in TLE patients with poorer seizure control may be caused by, or result in, pathological reorganization of brain connectivity involving a wide extent of abnormalities beyond the mesial temporal structures.25
Together with the wider extent of abnormalities, TLEPSC patients experienced more severe impairment especially in nonverbal memory, as revealed by their ROCFT-IR and ROCFT-DR scores. Furthermore, an association between greater disruption to functional integration and more severe memory impairment was observed only in TLEPSC patients. Brain regional correlates of memory impairment were not completely matched with the distribution of abnormalities, but the wider extent of abnormalities appears to be related to greater vulnerability to memory impairment. Cognitive impairment (e.g., memory impairment) may be concomitant with the extensive distribution of abnormalities as a manifestation of pathological reorganization.
The extent of abnormalities in terms of disrupted functional integration at the regional level seems to be strongly related to clinical severity, including poor seizure control and subsequent severe memory impairment in TLE, although mechanisms underpinning this connection need to be further investigated. We admit that the approaches in this study may not be used directly to make a diagnosis of seizure control status and memory impairment in TLE. Rather than proposing measuring disrupted brain functional integration to predict clinical factors, we hoped to ascertain its applicability and eligibility for being informative about clinical severity. Future steps to incorporate potentially crucial interictal features into a formal prediction model for seizure control or cognitive impairment would be warranted.
When we searched for disrupted brain functional integration in the interictal state, the distribution of abnormalities was mainly observed near the AH Sbc for both TLEGSC and TLEPSC patients. Concerning the reason why the AH Sbc was commonly involved in TLE patients regardless of seizure control status, the roles of the amygdala and thalamus in the AH Sbc should be mentioned. Both the amygdala and thalamus are involved in the initiation of seizures in TLE, as mesial temporal sclerosis often involves the amygdala26 and the thalamus is a critical component of the initiating circuit that supports the beginning of seizures.27 In addition, alterations in connectivity between the thalamus and other structures have been seen even in the interictal state.28 Identifying circuits or networks related to seizure initiation and propagation is one of the main concerns in epilepsy.29 Although interictal abnormalities tend to provide limited information, the sharing of common features of abnormalities between ictal and interictal states3 still suggests the possibility of applying information about interictal abnormalities to infer the seizure focus and paths of seizure spread.
Several limitations may be raised about the current study. First, it should be noted that the connection topology of brain functional networks is determined based on brain parcellation schemes.30 Our composition of subnetworks at the regional level may be arbitrary, but it is likely that a range of ways to construct functional brain networks and adequate numbers of subnetworks would produce similar abnormality profiles. Second, we dealt with brain functional networks of all TLE patients by placing the AH in the same side, as we were mainly interested in the effects of seizure control status, conceding a certain vagueness in determining seizure lateralization. In spite of confounding effects related to hemispheric lateralization of brain functions, neuroimaging studies for neurological diseases, such as stroke with unilateral lesions, 31 have often been conducted by locating the AH in the same side. Third, factors that may influence BOLD signals have not been fully taken into account. For example, seizure-related alterations in vascular structures and blood flows could affect BOLD signals, and thus resting-state functional connectivity and functional brain networks. In addition, we used the efficiency as a network parameter of interest on the grounds of consistent previous findings of disruption to functional integration over the whole brain, but alternate network parameters, such as degree32 and centrality,33 may be further tested to examine clinical relevance of abnormal connectivity in TLE. Finally, several patients had structural brain lesions, such as hippocampal sclerosis, which might have influenced our results on functional connectivity analysis. We could not thoroughly assess effects of structural alterations on seizure control status or changes in functional brain networks. Such effects should be investigated in a future study including more patients with various structural lesions.
In summary, we investigated clinical relevance of interictal abnormalities based on disrupted brain functional integration in TLE. We demonstrated that more extensive alterations in functional integration within and between brain regions could be related to poorer seizure control and more severe memory impairment. Such clinical relevance of disrupted brain functional integration represents a step toward employing rsfMRI for assessing clinical characteristics, possibly related to treatment outcomes or cognitive prognosis, in TLE patients.
1 : Altered fMRI connectivity dynamics in temporal lobe epilepsy might explain seizure semiology. Front Neurol 2014; 5:175Crossref, Medline, Google Scholar
2 : Influence of resting-state network on lateralization of functional connectivity in mesial temporal lobe epilepsy. AJNR Am J Neuroradiol 2015; 36:1479–1487Crossref, Medline, Google Scholar
3 : Ictal propagation of high frequency activity is recapitulated in interictal recordings: effective connectivity of epileptogenic networks recorded with intracranial EEG. Neuroimage 2014; 101:96–113Crossref, Medline, Google Scholar
4 : Gray and white matter volumes and cognitive dysfunction in drug-naïve newly diagnosed pediatric epilepsy. Biomed Res Int 2015; 2015;
5 : Alterations in white matter microstructures and cognitive dysfunctions in benign childhood epilepsy with centrotemporal spikes. Eur J Neurol 2014; 21:708–717Crossref, Medline, Google Scholar
6 : Multimodal effective connectivity analysis reveals seizure focus and propagation in musicogenic epilepsy. Neuroimage 2015; 113:70–77Crossref, Medline, Google Scholar
7 : Present and future of simultaneous EEG-fMRI. MAGMA 2010; 23:309–316Crossref, Medline, Google Scholar
8 : Causality within the epileptic network: an EEG-fMRI study validated by intracranial EEG. Front Neurol 2013; 4:185Crossref, Medline, Google Scholar
9 : Network connectivity in epilepsy: resting state fMRI and EEG-fMRI contributions. Front Neurol 2014; 5:93Crossref, Medline, Google Scholar
10 : Resting-state functional connectivity predicts the strength of hemispheric lateralization for language processing in temporal lobe epilepsy and normals. Hum Brain Mapp 2015; 36:288–303Crossref, Medline, Google Scholar
11 : Functional connectivity of hippocampal networks in temporal lobe epilepsy. Epilepsia 2014; 55:137–145Crossref, Medline, Google Scholar
12 : Specific resting-state brain networks in mesial temporal lobe epilepsy. Front Neurol 2014; 5:127Crossref, Medline, Google Scholar
13 : Human brain networks in health and disease. Curr Opin Neurol 2009; 22:340–347Crossref, Medline, Google Scholar
14 : Graph theoretical analysis of structural and functional connectivity MRI in normal and pathological brain networks. MAGMA 2010; 23:409–421Crossref, Medline, Google Scholar
15 : Structural brain networks and neuropsychiatric disorders. Curr Opin Psychiatry 2011; 24:219–225Crossref, Medline, Google Scholar
16 : State dependent properties of epileptic brain networks: comparative graph-theoretical analyses of simultaneously recorded EEG and MEG. Clin Neurophysiol 2010; 121:172–185Crossref, Medline, Google Scholar
17 : Characterization of functional and structural integrity in experimental focal epilepsy: reduced network efficiency coincides with white matter changes. PLoS One 2012; 7:e39078Crossref, Medline, Google Scholar
18 : Loss of network efficiency associated with cognitive decline in chronic epilepsy. Neurology 2011; 77:938–944Crossref, Medline, Google Scholar
19 : A proposed diagnostic scheme for people with epileptic seizures and with epilepsy: report of the ILAE Task Force on Classification and Terminology. Epilepsia 2001; 42:796–803Crossref, Medline, Google Scholar
20 : Update on surgical treatment of the epilepsies: summary of the second international palm desert conference on the surgical treatment of the epilepsies (1992). Neurology 1993; 43:1612–1617Crossref, Medline, Google Scholar
21 : Korean-California Verbal Learning Test (K-CVLT): a normative study. Korean J Clin Psychol 1997; 16:379–396Google Scholar
22 : Adult norms for the Rey-Osterrieth Complex Figure Test and for supplemental recognition and matching trials from the extended complex figure test. Clin Neuropsychol 1999; 13:30–47Crossref, Medline, Google Scholar
23 : Automated anatomical labeling of activations in SPM using a macroscopic anatomical parcellation of the MNI MRI single-subject brain. Neuroimage 2002; 15:273–289Crossref, Medline, Google Scholar
24 : Extratemporal white matter abnormalities in mesial temporal lobe epilepsy demonstrated with diffusion tensor imaging. Epilepsia 2006; 47:1360–1363Crossref, Medline, Google Scholar
25 : Seizure frequency can alter brain connectivity: evidence from resting-state fMRI. AJNR Am J Neuroradiol 2015; 36:1890–1898Crossref, Medline, Google Scholar
26 : Progression in temporal lobe epilepsy: differential atrophy in mesial temporal structures. Neurology 2005; 65:223–228Crossref, Medline, Google Scholar
27 : Neuronal circuits in epilepsy: do they matter? Exp Neurol 2013; 244:67–74Crossref, Medline, Google Scholar
28 : Thalamic functional connectivity predicts seizure laterality in individual TLE patients: application of a biomarker development strategy. Neuroimage Clin 2014; 7:273–280Crossref, Medline, Google Scholar
29 : Altered functional connectivity in seizure onset zones revealed by fMRI intrinsic connectivity. Neurology 2014; 83:2269–2277Crossref, Medline, Google Scholar
30 : Parcellation-dependent small-world brain functional networks: a resting-state fMRI study. Hum Brain Mapp 2009; 30:1511–1523Crossref, Medline, Google Scholar
31 : Longitudinal changes of resting-state functional connectivity during motor recovery after stroke. Stroke 2011; 42:1357–1362Crossref, Medline, Google Scholar
32 : Ictal-onset localization through connectivity analysis of intracranial EEG signals in patients with refractory epilepsy. Epilepsia 2013; 54:1409–1418Crossref, Medline, Google Scholar
33 : Are high frequency oscillations associated with altered network topology in partial epilepsy? Neuroimage 2013; 82:564–573Crossref, Medline, Google Scholar