A Video Ethnography Approach for Linking Naturalistic Behaviors to Research Constructs of Neurocognition in Schizophrenia
Abstract
Few methods are available to explore the impact of neurocognition in schizophrenia on behaviors performed in usual contexts. The authors developed a video ethnography approach to examine the relationship between naturalistic behaviors and research constructs of neurocognition. Video ethnographers accompanied subjects through usual routines gathering continuous video data. Researchers developed codes to measure four behavioral domains observed on video. This paper describes the psychometric characteristics to be considered in the development of observational approaches. It also highlights differences between behaviors performed in usual environments and neuropsychological constructs. The authors demonstrate that everyday behaviors that have been shown to correspond to neurocognitive skills in a pilot feasibility study can be identified and rated. They further suggest that observational methods could provide novel strategies for linking research findings and clinical concerns.
Hundreds of recent studies have examined the neuropsychological features of schizophrenia. The neurocognitive deficits associated with schizophrenia have been identified as key pathological factors in the disease and as strong contributors to functional outcomes.2 However, whether and in what ways measures of neurocognition predict the everyday behaviors of those with schizophrenia remain largely unknown. Almost no studies have compared standard assessments of cognition with assessments based directly on what patients do day-to-day in naturalistic settings.1,3,4 As a result, we do not know whether and in what manner the syndrome of cognitive deficits affects day-to-day behaviors, and the ways in which cognitive deficits correspond to visible symptom domains is still unclear.5–9 Overall, our research constructs of neurocognition remain largely decoupled from the lived experience of the illness.
There is growing interest in linkingdimensions of observable behaviors to markers of disease pathology.10,11 Data on the everyday behaviors that correspond to neurocognition could help characterize endophenotypes of disorders, decompose clinical diagnostic categories, and facilitate new insights into etiology. Such data may also clarify the impact of cognitive deficits on the everyday behaviors that treatment interventions aim to improve. After all, mental health treatments are not intended to change how an individual behaves in a laboratory or interview setting.12,13 However, few observational methods are available to document the everyday behaviors in context that are the ultimate target of treatments and that could facilitate basic research in schizophrenia.
In this article, we describe a novel method to measure everyday behaviors and to test their relationship to constructs of neurocognition in schizophrenia. The method uses video ethnography, a naturalistic, nondirective technique for recording everyday behaviors as they unfold in community settings. We developed a theoretically-driven measurement strategy with which to rate a range of everyday behaviors that seem relevant to the skills assessed with standardized measures of neurocognition. Findings from 93 hours of naturalistic data from 9 subjects with schizophrenia are presented elsewhere.1 In this article, we describe the rationale for a nondirective, observational approach to the study of everyday behaviors. We detail the process used to measure domains of behavior seen in the video, and we present the psychometric characteristics of the behavioral observation measures. We demonstrate that everyday behaviors that we have shown to correspond to neurocognitive measures in a pilot feasibility study1 can be identified and rated. We also highlight significant distinctions between the skills measured in the laboratory and the skills used in day-to-day environments, and we argue that this method can be used to bridge clinical and research questions in translational science.
Naturalistic Inquiry
Video ethnography involves discrete videorecording of a subject as he or she pursues regular daily activities in usual contexts. An ethnographer carries a video camera while following along with subjects during their everyday routine. As described below, video ethnography is naturalistic, in that it samples subjects’ spontaneous everyday behaviors in their moment-to-moment flow. It is also nondirective, such that no instructions are given to guide the subject as he makes choices. Ethnographers provide space for the subject to structure his behaviors within the options normally available to him, with only the incentives that normally predominate in his everyday life.14 Video ethnography can be grouped with other methods of ecological momentary assessment, such as experience sampling and diary methods.15 However, video ethnography differs from these approaches because it is entails direct observation. Observational approaches like video ethnography are understood to be the gold-standard approach to the assessment of ecological validity.16
Ecological validity11,17,18 concerns the predictive relationship between performance on standardized tests and behavior in real-world settings.19 Two approaches to ecological validity are available: veridicality and verisimilitude. Most approaches to ecological validity use a verisimilitude approach,20,21 in which tests are constructed to mimic the tasks an individual might perform in life. For instance, some functional measures assess individuals’ capacity to perform cognitive skills in simulated settings, such as using a bus map to plan a trip to a standard location (e.g., the zoo).22 In contrast, veridicality approaches assess the degree to which existing tests are empirically associated with everyday experiences.23 Veridicality is very rarely assessed because it requires systematic evaluation of everyday behaviors.24 The observational method described here is an example of a veridicality approach to ecological validity. A recent review concludes that, in establishing ecological validity, naturalistic observation is “technically the ideal” (p. 813) approach4 because it shows how behaviors manifest in the real world.25
Because they capture behavior as it unfolds in natural contexts, observational methods have been used as a benchmark in the study of complex social and psychological behaviors since the 1930s.26–29 In addition to their widespread use in ethology,30,31 naturalistic observation methods have been used extensively to characterize the dynamics of children’s behavior,32–37 pathological and normal family interactions,38–46 the quality of medical care,47–49 and a range of aspects of education,50 such as teacher quality51 and curriculum pacing.52,53 Advances in video technology have facilitated this work because video offers an exceptionally high degree of behavioral detail for observational analyses. The video record can be reviewed and rereviewed by multiple researchers. Videotaped behaviors can be objectively measured and/or rated through video coding. Video observational approaches have been used rarely in the study of psychosis to analyze social problem-solving54 and social interaction.55–57 Taken as a whole, this literature suggests that observational approaches may hold untapped promise in the study of everyday functioning in schizophrenia.
Video ethnography captures cognition and functioning not as static capacities, but as dynamic, flexibly-deployed resources that unfold minute-by-minute. They are tracked as naturalistic behaviors: spontaneous, fluent, and performed as a matter of course,58 or what qualitative researchers call naturally-organized ordinary activities.59 The observational record includes, rather than eliminates, the environmental and interactional factors that form part of everyday behavior.60–62 Aspects of the subject's world are not external to cognition, but are integral to it, to the extent that the environment is replete with tools, foils, and motivators for action.63–65 Naturalistic data can be contrasted with data that emerge through the suppression of normal routines (such as in the lab) or the structure imposed by research tools.66 Some argue that naturalistic inquiry provides a particularly apt strategy for investigating causality because it characterizes “the qualitative nature of social objects and relations on which causal mechanisms depend (p. 4).”67 Indeed, many moderators of outcome in schizophrenia (e.g., social support, motivation) probably function contingently rather than deterministically, exerting salutary or detrimental—and substantial or minimal—effects, depending on adjacent factors. Observations are also closely tied to the types of specific behaviors that could be targeted for intervention or that serve as indicators of treatment outcomes.29
Psychometric Considerations
Several psychometric properties are critical to consider in the development of observational approaches. In general, face and content validity are the most important to establish in behavioral observation29,36,46,68 because they clarify how well the targeted phenomena have been operationalized in the data collection. Face validity is a judgment based on how reasonable the connection is between a construct and its measurement. Content validity addresses the degree to which the measure captures important aspects of the construct as it has been defined, and is often based on expert opinion or theoretical consensus. Questions such as, “To what degree is the behavior coded in the data reflective of the daily life situations that are most relevant to patients’ progress?” and “To what degree do the codes capture meaningful aspects of daily life that we would expect the pathological condition to impact?” reflect issues of face and content validity. Because of method variance, convergent validity with structured assessments of related constructs may not be a critical attribute of the validity of observational approaches; but demonstrating that observational coding strategies can distinguish between subjects who score high or low on structured assessments of related constructs can support their concurrent validity, as we describe below. Adequate behavioral sampling (also called time-sampling) is another critical aspect of observational method validation, as it indicates that researchers have observed for a sufficient duration to capture the true variation in the sample.69,70 Interrater reliability, which ascertains that multiple coders rate behavior similarly, must also be established to verify the consistency of the protocol. Test–retest reliability (i.e., day-to-day stability of coded behaviors across observation sessions) is important to establish because it can suggest that the data collection has captured consistent attributes, rather than situation-dependent or state-related individual differences.71,72 Finally, the use of strategies to minimize participant reactivity43 to the data collection can improve the ecological validity of the data, although multiple studies that have assessed it empirically have not found evidence of participant reactivity to natural observation.40,73–78
The detail and validity of video ethnography distinguish it from other approaches to tracking everyday behaviors. We attempted naturalistic observation using audiorecording and field notes but behaviors could not be captured with audio alone, and the observer struggled to take adequate field notes. Methods that do not use direct observation (e.g., ecological momentary assessment) can assess the affective and behavioral dynamics of everyday contexts through self-report,79–81 and they are feasible in individuals with schizophrenia.82 But the data collected through these methods lack the detail of the video record and only include reports of actions after the fact. In contrast, video ethnography directly records everyday activities, thus minimizing recall- and response-bias. Video ethnography also avoids other limitations of laboratory- or interview-based measures of functioning, including retrospective recall and state-dependent biases and the artificiality of simulations used in performance-based assessments.83 Moreover, many behaviors indicating cognitive skill are likely performed spontaneously without explicit thought; most of the coded actions described below reflect behaviors or interactional patterns that were not accessible to subjects themselves and thus could not have been reported.40,46 Finally, video allows for what Schwabe et al. call “unmotivated looking (p. 715).”84 Given the paucity of data on the everyday manifestations of cognitive deficits, a protocol that assesses deficits through prompts would require countlessa priori assumptions, and potentially crucial behaviors would inevitably be missed.
Using methods that have special validity in the assessment of everyday behaviors could have significant clinical and research impact. We see three potential implications of establishing empirical links between research constructs and everyday behaviors. First, these links can demonstrate the relevance of research findings to clinicians, facilitating translation and dissemination.Clinicians focus on global, visible, or articulable aspects of an illness, such as hallucinations or poor insight.85,86 Both self-87 and clinician88reports of cognition are poorly correlated with laboratory-based measures. Second, empirical links between neurocognitive constructs and everyday behaviors could facilitate biological research into intermediate phenotypes of schizophrenia and psychosis. For instance, neurocognition has emerged as a promising intermediate endophenotype of schizophrenia,89–91 and the everyday behaviors that correlate with cognitive deficits may be visible or downstream markers of genetic liability. Finally, clarifying the link between research constructs and everyday behavior can establish the ecological validity of measures that are widely used in clinical research.4,92
Methods
Here, we present our approach to the video data collection and data analysis in order to describe the choices required in the development of these methods. Because we recognize that many other choices could have been made, we include substantial methodological detail so that readers may evaluate the validity and potential value of our approach and naturalistic observation in general. Study procedures were approved by the Institutional Review Board of the University of Southern California. The full pilot protocol is described elsewhere.1 We recruited community-dwelling subjects who had completed a diagnostic interview and neurocognitive battery as part of an ongoing study. Subjects with SCID-diagnosed schizophrenia or schizoaffective disorder were approached for enrollment if their MATRICS Consensus Cognitive Battery (MCCB) composite score fell within the top or bottom ⅓ of all subjects (N=155) in the ongoing study. We approached 12 eligible clients to enroll 9 subjects: 5 scoring in the bottom ⅓ and 4 scoring in the top ⅓ of the cohort. After they consented, we videotaped subjects in their natural living contexts across multiple sessions. We taped individuals for from 1 to 6 sessions (average: 4.4) during different times of the week and weekend days. Sessions lasted 2 to 5 hours. During piloting, we reviewed as a team the degree of behavioral variability observed within and across each observation session. We varied the length of observation sessions to verify the portion of a day required to capture longer events, and we varied the days (weekend, weekday) and times (morning, late afternoon) of observation to capture the widest range of behavioral patterns. Total taping time per subject ranged from 3 to 18.5 hours (average: 10.3 hours). We gathered a total of 93 hours of video.
We modeled our ethnographic technique on “go-along” ethnography.93 In go-alongs, an ethnographer accompanies the subject on daily routines, participating and listening while documenting. As described in Table 1, ethnographers are nondirective and behave naturalistically. They carry a semiprofessional, high-definition camera measuring 14” × 6” × 6” with all accessories. The act of recording requires no monitoring. The ethnographer passively allows the camera to run, carrying it with a shoulder strap or setting it down. The camera has a retractable monitor. Ethnographers check the frame by glancing down and never look through the eyepiece. A tiny, highly-sensitive omnidirectional microphone is pinned to the ethnographer, and a positional microphone is mounted on top of the camera. The subject is not wired.This technique is extremely discreet. Onlookers often do not know that filming is taking place, only that someone is carrying a camera, which is relatively common in Los Angeles, where data collection took place. The action is imperfectly framed at times (e.g., while ethnographer and subject walk to the store, the subject is seen obliquely from waist to nose only), but this did not impede data coding, because the audio track combined with visual cues is sufficient to clarify subject behaviors.
Feature | Strategy |
---|---|
Nondirective | Remind subject: “Do whatever you’d do if I were not here,”73as often as necessary; give no other instructions; do not express preferences for subject’s plans; do not help the subject make decisions; be explicit that you can follow subject anywhere. |
Naturalistic | Be within action alongside subject; passively allow camera to run; let camera hang at hip or sit on table; never hold camera to face or look through eyepiece; when appropriate (e.g., at a bank counter), step back from action; dress casually; be friendly and relaxed; ask and answer questions as a peer would do. |
As stated above, participant reactivity to the method is an important consideration. We debated several aspects of data collection with regard to their potential to create reactivity. We filmed subjects from afar, but subjects found this to be unnerving, and onlookers took more notice. Mounting a camera and leaving it to run has produced unusual subject reactivity in other studies,14,64 and our subjects rarely stayed in one room throughout data collection. Leaving a camera to run also yielded less rich data, because people do not narrate their actions while alone, but, when accompanied, their motivations and intentions are often verbalized. Pilot subjects verbalized planning (e.g., “I need to get off in two stops,” or “We'd better leave now to get there before 4.”) and described their behaviors (e.g., to whom a phone call was made). Without this narration, coders would need to make countless inferences, such as whether the subject planned to link errands together. Although accompanying the subject introduced a stimulus, many pilot subjects found the situation mundane because they were often accompanied by case-managers or peers. Finally, we aimed to minimize reactivity by not informing subjects of the coding categories and by giving them no instructions for performance, so they would not know how to demonstrate skills or hide deficits. Pilot data collected on the issue of reactivity are provided below.
Code Development
After collecting data, we developed data-analytic approaches to convert the spontaneous, unstructured behavior captured on video into reliable units.94,95 We drew on neuropsychological theory and practice to guide code development. The research team began by reviewing existing paradigms for measuring domains of neurocognition.We also studied strategies for decomposing and scoring complex behaviors, the literature on goal-formulation, problem-solving therapy, and diary methods for functional assessment. Each literature area provided hypotheses about the categories of behaviors that might represent the everyday manifestations of cognitive and functional skill. One author (EB) conducted surveys or consultations with six senior neuropsychologists and two rehabilitation researchers to explore ideas about the everyday behaviors that correspond to cognitive domains. The responses were diverse, but did provide some viable suggestions for assessing cognitive skill from daily activities and were relevant to the content validity of our measures.
Some behaviors identified by these experts as cognitive could not be rated from video. Respondents mentioned behaviors that most subjects would not perform spontaneously (e.g., memorizing a grocery list, listening to a 60-minute lecture). For most behaviors, the subject’s skill would also be difficult to rate because a normative standard for performance has not been established. Finding one's way around a new building—identified as an everyday example of visual learning and memory—is not a skill that could be reliably rated because no standard sequence or time-limit could signify when it is done well. Remembering a piece of information may reflect memory skills, but having forgotten information cannot always be judged as a significant instance of forgetting. Moreover, many skills are not verbalized. Reading others’ emotional states is typically performed tacitly. The most obviously cognitive tasks (e.g., making change, reading a map) were too rare, varied, or incompletely verbalized to be scored.
Next, we began a collaborative process of viewing the video to consider which visible behaviors could be coded and which could be reflective of the skills and domains of cognition and functioning. We avoided potential bias due to expectancy effects on the part of raters71 by keeping all members of the research team blind to subjects’ performance on the MCCB throughout code development and coding.We devised four criteria for the codes. Coded behaviors: 1) are observed, not inferred or reported; 2) exclude the ethnographer; 3) include reliable descriptors; and 4) reflect skills, rather than deficits.
Observed, Not Reported, Action
The validity of self-reported information can be difficult to gauge.96,97 Subjects frequently described social interactions, plans for solving problems, or intentions for the future. Subjects talked about hopes of returning to school or tasks like refilling medications that they planned to finish that day. Such statements seemed functionally relevant, but they could not be reliably differentiated from unverifiable reports. One subject described a plan to move into a shelter and get a job registering voters in order to qualify for a housing voucher. Another talked frequently about the businesses in the neighborhood he frequented, but had only once left his apartment after 5 days of visits. Unlike reports, subjects’ actions were observable. Subjects set plans for the day and then saw them through. They worked through challenges, such as looking for information on the computer. The team focused on accurately measuring these examples of “action in the moment.”
Exclude the Ethnographer
As described above, the presence of a friendly ethnographer accompanying the subject improved the quality of the data we gathered. However, to minimize the impact of observer effect on the data, interactions with the ethnographer are not coded as activities or as social interactions. Nonetheless, subjects did sometimes engage with ethnographers in problem-solving behavior that appeared ethnographer-independent. For instance, when a subject spontaneously suggests to the ethnographer that, “you can get change for the bus at the liquor store,” he has inadvertently engaged the ethnographer in problem-solving. As described below, naturalistic problem-solving often involves the people around the subject, and our measure of subjects’ problem-solving skill includes behaviors in which the ethnographer is engaged, such as in the above example. However, in all cases, we witnessed subjects pursuing identical problem-solving with others in their environment. To lessen their potential impact on subjects’ problem-solving, ethnographers were explicitly trained not to help subjects solve problems or make decisions (e.g., to wait for the subject to push the elevator button, even if the ethnographer knows where the subject intends to go).
Reliable Indicators
We take advantage of the continuous time-record of the video to code the duration of many activities. Other reliable indicators include concrete behaviors used to measure relatedness (e.g., using the interlocutor's name, making eye contact, asking follow-up questions, gestures), and the activities that indicate the complexity of subjects’ time use (e.g., making a phone call, going to the library, watching TV).
Code Skills, Not Deficits
In general, skills are more reliably observed than deficits. The team often could not concur in identifying the absence of an action, a lack of memory for facts, or inappropriate inactivity. For instance, we aimed to identify instances in which a subject ignored problems in his or her environment, yet we rarely agreed. Reliable codes and rating scales were developed by focusing on observed skills (e.g., seeking out a companion, generating multiple solutions to a problem).
Results
Community Performance Indicators (CPIs)
We call the final codes community performance indicators (CPIs). The codes (see Figure 1) measure four domains of everyday behavior as seen in the video: 1) problem-solving; 2) social interaction; 3) behavioral activity level; and 4) goal pursuit. Here, we describe the development of these codes, drawing on findings from 93 hours of videotape. The four CPI codes are briefly summarized in Table 2. In what follows, we describe in detail the justification, logic, and coding of the CPIs.
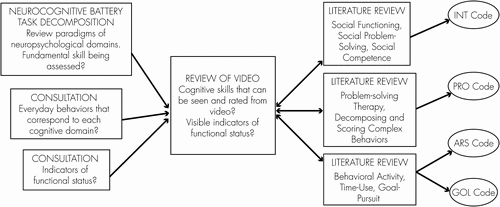
CPI Domain | Description of Behavior Tracked | Selected Variables Generated |
---|---|---|
Problem-Solving (PRO) | Management of a novel circumstance, satisfaction of a need or fixing of present complications; planning ahead; some helping behaviors; type of problem-solving skill used | Frequency of problem-solving behavior; average problem-solving skill-level |
Social Interaction (INT) | Quality and quantity of observed social interactions | Average duration, frequency, relatedness of interactions; frequency of initiation of interactions; percent of total time spent in interaction |
Activity Level (ARS) | Complexity and initiative of activities pursued in each 15 minutes of video; subject’s use of sequencing and multi-tasking in daily behaviors | Average highest activity level; average predominant activity level; variability of activity level from day to day |
Goal Pursuit (GOL) | Time allocation across six domains of activity pursuit; whether subject pursues or receives these activities | Percent time spent on no goal pursuit; percent time spent on pursued goals; percent time spent on received goals |
1. Naturalistic Reasoning and Problem-Solving (PRO Code)
Deficits in executive functioning, or reasoning and problem solving, are some of the most consistently identified cognitive problems in schizophrenia.98,99 Also, individuals with schizophrenia demonstrate deficits in social problem-solving, including problem-recognition, problem-definition, and generating alternatives.100–102 Naturalistic instances of everyday problem-solving are common, often verbalized, and observable on videotape.
For guidance to define a category of problem-solving as performed in-situ, the team looked to neuropsychological test paradigms. In these paradigms, reasoning and problem-solving is a process by which problems are identified and solved by abstract reasoning.103 Test paradigms for this domain assess a variety of skills, including spatial skills,104,105 set-shifting, response initiation and suppression, and nonverbal components rule selection.106 We focused on the Wisconsin Card-Sort Test (WCST), the Rey-Osterrieth Complex Figure Test (RCFT),107 the Tower of London,108 the Independent Living Scales–Problem-Solving Factor subscale (ILS–PB),109 and the Neuropsychological Assessment Battery–Mazes (NAB–Mazes). The Tower of London and the RCFT involve manipulating unusual stimuli to achieve a highly structured goal, making these tests difficult to compare with real-world problems.110 In the video, we could find corollaries to the set-shifting skills tested by the WCST, to the time-limited problem-solving required for the NAB–MAZES, and the skills required to identify a solution to a hypothetical problem as posed in the ILS–PB. These tests require the subject to recognize that a novel circumstance presents itself, to respond effectively to that circumstance, to generate solutions in a timely manner, and/or to weigh a range of potential solutions.
Social problem-solving assessments and problem-solving therapies emphasize similar skills. Problem-solving therapies stress the identification of problems, positive problem-solving orientation, solution-generation, and effective response. Social problem-solving assessments test skills in identifying problems (called receiving), generating solutions (processing), and choosing effective solutions (sending). To map these skills to video data, we looked for reliable indicators that the subject faced a challenge (e.g., when a subject did not know how to turn on the oven). However, mere identification of a problem (“I need some cigarettes;” “I need a bigger apartment.”) could not be coded, because, in some of these instances, problem-solving could reasonably be postponed or might never be pursued. We code problem-solving behavior only when the identification of a problem is followed by action.
Our first few subjects demonstrated an aptitude for problem-identification and solution-generation in the course of efforts to help others. One subject repeatedly clarified what the ethnographer would need to do to take the bus back to his car. He explained how much change was needed, pointed out the corner at which to wait, estimated the wait-time, advised the ethnographer how to know when the bus approached the appropriate street, and so on. On other occasions, he provided information to orient the ethnographer (“I'm going to change my shirt, and then we can go.”). The subject was anticipating hypothetical problems and aiming to prevent them.
Then, we recognized many anticipatory behaviors that did more than fix a present challenge. Subjects converted a situation that was not problematic per se into an opportunity to improve the present. These behaviors involved generating options. One subject took a seat on a bus bench as she and the ethnographer walked past. “I'm not taking the bus; I'm just going to rest here,” she said; she was tired, and she took advantage of the bench to address that. Another subject looked at his watch as he took the bus to class; “we will have time to have another cigarette before class,” he said. Another subject, recalling the need to go downstairs, pointed to the elevator and asked, “We could take the elevator, or we could take the stairs. Which should we take?” Both anticipating potential problems and identifying opportunities require the same planning and reasoning skills as problem-solving.
After review of these examples and successive trials, we defined the problem-solving code: We mark problem-solving when we see problem-identification, positive problem-orientation, and solution-generation together. We include “helping behaviors” and explanations for others when they are both a response to an explicit need and when they have implications for action in the moment. In other words, problem-solving (PRO) behaviors are those in which subjects are seen to manage a novel circumstance, satisfy a need, or fix complications in the present. PRO behaviors may also be explicit, in-the-moment attempts to improve a situation. PRO behaviors include planning whether there is time to stop at the store and telling another person how to get to a landmark when such a need is expressed. In pilot data, raters agreed 78.3% (standard error [SE]: 0.09) of the time that problem-solving was present or absent. Across all nine subjects, PRO behaviors occurred approximately once per hour. However, problems could be identified and solved in successive stages over a considerable period of time (such as when a subject said, “I need to get a ride from my sister,” and made three phone calls to three different people over the course of an hour). The time required to solve a problem was not rated. Instead, we rated the kinds of skills used to solve each problem. Problem-Solving Skill, a 3-point scale, rewards anticipatory and creative—as opposed to rote and rudimentary—problem-solving. Since, in our pilot work, problem-solving instances occurred approximately once per hour, we could achieve adequate sampling in an observational period of 10–15 hours. The interrater reliability for PRO skill rating was excellent (kappa: 0.87; SE: 0.05), and the internal-consistency reliability, or day-to-day stability of PRO skill use (i.e., from one session to the next), was good (Cronbach’s alpha: 0.68).
2. Social Interactions (INT Code)
Social cognitive deficits and impairments in social functioning are high-priority areas in schizophrenia research.111,112 Social cognitive deficits may mediate the relationship between neurocognition and functioning,2 and social functioning itself is an important outcome.113 An NIMH workshop defined social cognition in schizophrenia as comprising five skill areas: theory of mind (or mental state attribution), social perception, social knowledge, attributional bias, and emotional processing.114 In general, these processes are too discrete or implicit to be identified in videos. Statements of attributional bias can be coded in transcripts,115 but we did not have structured narratives for comparison across subjects.
Pilot subjects spent a great deal of time reporting social behaviors (e.g., “I'm going to class tomorrow with my brother.”). One subject described friends down the hall, a boyfriend, and a granddaughter. Yet subjects' reports of interactions did not always correspond to social activity. One subject mentioned frequently talking to God, and another talked intimately about Oprah Winfrey; a third spoke aloud to someone else when the ethnographer left the room; and many of the individuals mentioned by subjects were never seen to visit or call. We found these reports of social interactions difficult to evaluate. If we could not confirm that the subject really spent the afternoon with a brother, as reported, we could not justify coding this information as an indicator of social activity. And, although we found it significant that some subjects reported receiving substantial psychosocial support from relationships that were best understood as intra- rather than inter- personal (e.g., Oprah Winfrey), we felt we could not code these as social ties. Ultimately, we instead chose to design a coding strategy to take advantage of video ethnography’s unique ability to document manifest social interactions. Video can document the interactions with others that actually take place in the course of subjects' everyday routines, an aspect of subjects' social lives that is very rarely studied.
In our coding, social interactions are timed from beginning to end. They begin when the subject engages in an interaction and end when the interlocutors part; the joining in of a third individual is counted as a new interaction. Interactions that take place over the phone, by text message, or e-mail are rated identically as in-person interactions. Tracking duration allows for calculation of the average length of interactions, the percent of interactions that are short (less than 30 seconds) or long (e.g., longer than 3 minutes), and the percent of a subject’s total time spent in interaction. Interaction duration measurements reached very high interrater agreement (kappa: 0.99), but stability of mean interaction duration from session to session was not high; α reliability improved to 0.51 when Sessions 1 and 2 were compared with Sessions 3 and 4, suggesting that longer periods of observation may be required to assess average interaction length.
Also, we rated the quality of the interaction with a scale of relatedness. We adapted the Maryland Assessment of Social Competence100 to develop a 3-point scale that rates whether the subject displays efforts to extend the length of an interaction or facilitate the level of engagement. Use of the interlocutor's name, follow-up questions, eye contact, reactive affect, gestures, and intonation all indicate relatedness. The presence of several of these features yields a Relatedness score of 2 for the interaction, and their absence yields a score of 0. Interrater reliability was good for the Relatedness scale (kappa: 0.75; SE: 0.10), and stability across sessions was high (α: 0.84).
3. Behavioral Activity Level (ARS Code)
Time-budget approaches have demonstrated a clear relationship between activity level and clinical improvement in schizophrenia.116,117 Engagement in social activities, employment, and activities of daily living (ADL) competence are key components of assessments of functioning.118,119 In video examples, behavioral activity level was an obvious difference between subjects. The initiative, effort, goal-direction, and complexity of daily activities varied across subjects and across sessions. Behavioral activity level appeared salient to neurocognitive capacity and could be reliably tracked in the video.
We adapted an existing scale of activity level to rate behavioral activity in brief increments of video. The original scale120,121 rates the activities in a diary the subject completes with an interviewer, scoring 4 time-periods in the day. It is scaled toward active, organized, and motivated behaviors, with subjects who participate in passive, solitary activities receiving lowest scores. Our adaptation, the 5-point Activity Rating Scale (ARS), rates the level of complexity and initiative required for subjects' behaviors seen in the video. We added activities commonly seen on video (e.g., phone calls) to the scale. The five points of the ARS scale are anchored by both specific behaviors (e.g., eating a meal, using a microwave) and behavioral descriptors (e.g., doing simple tasks one after the other, an isolated activity requiring some effort). The scale remains weighted toward complex and effortful behaviors and toward multitasking (ARS=4) and sequencing of behaviors (ARS=3). Time in which a subject cooks “from scratch” while fielding a phone call receives a 4; time in which a subject watches TV receives a 0. We give an ARS rating for each 15 minutes of video; 15-minute increments showed better range and interrater reliability than 10- or 20-minute increments.
The ARS scale captures the quantity of activity (e.g., how busy subjects were) and its organizational quality (e.g., whether tasks were sequenced together toward an explicit goal). For example, two subjects spent most of their first observed sessions in their neighborhoods. The first subject walked from class to the library to return a video and pick up another. Then he went across the street to pick up food for dinner. Then he walked to the bus stop and caught a bus home. In 45 minutes, he pursued tasks requiring some effort and initiative, and he also sequenced these tasks together to pursue them efficiently. He received ARS scores between 2 and 3 for the time, with a total score of 8. The second subject also spent 45 minutes walking in his neighborhood. He stopped at stores, often to say hello to a clerk, but he did not buy anything. He greeted those he recognized on the street. He decided which direction to walk in the moment, without a planned route or a final destination. Once, he yelled across the street to someone he knew. Although his walk through the neighborhood was as busy as the first subject’s, and whereas, superficially, he was engaged in the same tasks (e.g., going to the store), he lacked initiative and organization. He passively received an activity as it came into view (e.g., wandering into a store). He moved from one activity to the next without direction. As a result, this subject received ARS scores between 1 and 2 for the 45 minutes, with a total score of 4 (the score of 2 was the result of seeking to engage the company of the acquaintance across the street).
In fact, each 15 minutes is assigned 2 ARS scores, a highest and a predominant score, because some complex activities were extremely brief. If a subject is watching TV, but then makes a phone call and leaves a message, which takes 30 seconds, the subject receives a highest score of 2 and a predominant score (defined as the complexity of activity pursued for at least 7.5 minutes) of 0 for the 15 minutes. Use of the highest score increases the range of the scale used by coders and gives credit to subjects for short but complex behaviors. Yet, because it credits very brief behaviors within a background of inactivity, the predominant score is also used to reflect the overall level of activity. Both ARS highest (kappa: 0.77; SE: 0.09) and ARS predominant (kappa: 0.93; SE: 0.05) showed excellent interrater reliability and adequate alpha reliability across sessions (0.79 and 0.70 respectively).
4. Naturalistic Goal Pursuit (GOL Code)
Finally, we developed the goal-pursuit scale (GOL) to describe time use over longer blocks of time than the ARS scale and to indicate whether subjects actively pursued certain experiences. The GOL scale provides a global assessment of subjects’ time use. Although the skills tracked with the GOL scale reflect categories of functioning, cognitive skills—such as executive functioning,122 metacognition,123 social cognition,124 and verbal memory125—could play substantial roles in the pursuit of these activities. The GOL scale is modeled on time-budget approaches.126–128 We categorize the activity seen on video into one of six domains denoting the type of activity (socializing, leisure, spirituality, activities of daily living, health, and vocation/education). The domains were adapted from the Activity Pattern Indicators scale129,130 and from a taxonomy developed by Bogardus and colleagues.131 In addition to these six goal domains, subjects occasionally pursued no goal, such as when napping or conversing only with the ethnographer. A seventh category—no goal pursuit—was created for those time-blocks. Multiple raters showed good reliability (84.6% agreement; SE 0.06) in assigning goal domains.
In addition to the goal domain, blocks of time are rated as pursued or received, denoting whether subjects passively take part or actively seek out the activity. In this sense, like the ARS scale, the GOL scale credits motivated activities. For example, time during which a subject travels from home to attend church receives a GOL code of “spirituality; pursued;” time during which a subject sits on the porch at a group-home talking with peers receives a GOL code of “socializing; received.” This emphasis is consistent with self-determination theory, which states that facilitating internal motivation fosters competence and potential;132 it is also consistent with mental health and medical interventions133 that aim to foster autonomy and competence. For these reasons, we use the GOL code to distinguish between activities that are a response to stimuli in the immediate environment (received) and those that reflect an intentional effort to expand beyond those stimuli (pursued). Interrater reliability was excellent in distinguishing pursued versus received goals (92.3% agreement; SE: 0.04), and session-to-session stability was adequate (α: 0.59).
Community Performance Indicators (CPIs): Additional Psychometric Properties and Correlations With the MCCB
We pursued a further check on face validity by asking subjects to rate the degree to which they believed the CPIs represent important domains of their community functioning. On Likert-scale items, subjects agreed that setting goals and pursuing them, being able to solve problems, and setting plans are important to them. All agreed that talking with others and being active are activities they pursue more often when doing well. All but one subject agreed that it is important to them to leave their apartment during the day. Regarding reactivity, we asked subjects to complete Likert-scale items after the second and final observation session. Almost all subjects reported forgetting about the videotaping after 30 minutes or after the first session. In open-ended responses, 8 of 9 subjects described their behavior as the same as their usual behavior during filming; the ninth reported “being more polite.” We also asked three members of the recruitment team who knew the subjects from the clinic context to comment on their behavior on video. All three strongly agreed that the subjects behaved naturally on videotape. These results mirror reactivity findings in other studies,134,135 the majority of which find no reactivity to observational approaches.74–78
Results of correlational analyses between the MCCB and CPIs are described more fully elsewhere.1 We comment here on a few findings that support the validity of the approach. First, our sampling strata based on MCCB scores was achieved, with statistically significant differences (Kruskall-Wallis chi-square 6.0, df: 1, p <0.01) between the two groups of subjects high and low on MCCB composite and domain scores. Second, the high- and low-neurocognition subjects showed statistically significantly differences on four CPI variables, one from each CPI domain, in the predicted direction (better neurocognition corresponding to better CPI scores; chi-square, [df: 1] 3.0–6.0; p <0.05 [one-tailed], in all cases using the Kruskal-Wallis test statistic). Third, the Pearson correlations between MCCB composite scores and these four CPI variables ranged from 0.54 to 0.77 (p <0.01 to 0.07), mirroring the between-group findings. Fourth, the Pearson correlation between the MCCB composite and a z-score CPI composite derived from these four variables was 0.82 (p <0.01). We partially attribute this elevated correlation to our strategy of sampling extreme groups. Fifth, the CPI domains showed a pattern of specific correlations with neurocognitive domains. For example, while Working Memory and Visual Learning had strong associations across the CPI domains, Verbal Learning, Reasoning/Problem-Solving, and Social Cognition had strong associations with CPI domains of Social Interaction and Problem-Solving (r >0.42; p <0.05 in all cases). These findings were very similar when using a nonparametric correlation coefficient.
Discussion
This article provides a thorough description of the video ethnography method and the coding categories we developed to explore the link between everyday behaviors and neurocognitive constructs. As described elsewhere,1 we achieved high interrater reliability in using these CPIs to code 93 hours of video. In our sample of 9 community-dwelling subjects who scored high or low on the MCCB, variables from all four CPI domains demonstrated correlations with both MCCB composite and domain scores. On the basis of our pilot data, we believe the CPIs constitute a valid approach to exploring the cognitive content of everyday behaviors and to testing the ecological validity of neurocognitive and functional measures. The four CPI domains do not track all aspects of everyday behavior, but they capture a wide range of behaviors and correspond theoretically to domains assessed with neurocognitive and functional measures. Also, because they describe fundamental features of everyday life (e.g., time-use, activity-complexity, level of social interaction), these domains of behavior seem likely to represent mechanisms by which functioning occurs. They also provide a framework for future observational approaches that develop alternative or elaborated measurement strategies.
Despite remarkable advances in our understanding of neurocognition in schizophrenia, we know very little about how neurocognition affects everyday behaviors in usual contexts. Video ethnography provides a rich record of behavior in context, allows for viewing and reviewing of data to reliably measure a range of behaviors, does not require prompts or self-report, and is feasible with individuals with schizophrenia. Measurements like the CPIs, which assess naturalistic behaviors, can rate the quality and quantity of the everyday experiences that treatment interventions ultimately aim to improve. Practically, such data could facilitate translation of neurocognitive research findings to clinicians and could stimulate neurobiological research by decomposing complex phenotypes (e.g., cognitive impairment) and disease manifestations (e.g., functional impairment). Fundamentally, data about naturally-occurring behaviors may help to clarify the relevance of research constructs to the lived experience of those with schizophrenia.
Differences Between Naturalistic Behaviors and Neurocognitive Constructs
We encountered several complexities in the behavioral observation of cognition. These complexities require attention in future research. As described above, numerous skills assessed in neurocognitive tests could not be directly viewed or reliably rated. The team could find no reliable markers in the video for skills in working memory, verbal learning and memory, visual learning and memory, attention and vigilance, and speed of processing. We tried but failed to track several other cognitive behaviors, including perspective-taking and emotional attribution. We also attempted to track joking and sarcasm as they emerged naturalistically. A scale was developed to assess aspects of metacognition, like noting shared circumstances (e.g., the weather) or making self-evaluative statements. In all cases, we faced immediate reliability challenges. Many of these behaviors would have required the coder to make inferences about the subject's intentions or to score activities for which no normative standard is available. Given the relevance of these skills to the psychopathology of schizophrenia, we would encourage further exploratory work to develop rating scales for the naturalistic manifestations of these cognitive skills.
Moreover, neuropsychological problem-solving test paradigms differ in important ways from the problem-solving behaviors we witnessed in everyday life. First, everyday problem-solving can rarely be divided into social and nonsocial types. Both identifying and solving problems involve interactions with others, and the skills a subject uses in solving his own problems are also used in anticipating others’ problems. Second, the salience of identification, solution-generation, and effectiveness varies for everyday problems. In real life, as Koren et al. say, “the person is free to choose which aspects of the question to relate to and which to ignore, what degrees of confidence to impart, what perspective to adopt, how much detail to volunteer, and so forth (p 310).”18 In everyday life, problems do not announce themselves; they emerge slowly or are brought to the fore by the subject, and they usually evolve in ways that depend on the subject’s reaction. The nature of the appropriate response is rarely highly determined. Problem-solving can continue over a long period of time, not because of lack of skill, but because a subject continues to generate new options. Subjects can convert a problem into an opportunity, pursue adaptive avoidance, or focus selectively on a subproblem that can be successfully managed.
Finally, the team could not rate the degree of difficulty of naturalistic problems and could not always agree on when problems were completely solved. Some naturalistic problems were readily solved (e.g., a subject forgot a key and walked back to get it); yet, even here we could not judge the complexity of the challenge. Were problems like figuring out the VCR difficult? Should we use our perception or the subject's? For other problems, it was not easy to judge whether the problem had been adequately solved or whether a better possible solution remained. For instance, in response to an inquiry, a subject said that she believed that parking was allowed on a particular street at that time, but she neither seemed certain nor went down to the street to confirm this. Self-corrections—as in the case of the subject who realized she got on the bus going in the wrong direction—could be equally difficult to judge as effective or timely. Unlike mazes or set-shifting exercises, real-world problems present a variety of potential resolutions, and they may lack closure through no fault of the problem-solver.
Limitations and Future Directions
We believe that the videorecording protocol provides a representative snapshot of subjects' everyday functional performance. However, the pilot study had several limitations, and some questions could only be explored in a larger, heterogeneous sample. Clearly, data from such a small, select sample do not establish the ecological validity of the MCCB. Only data from a larger sample could test correlations between CPIs and a range of cognitive and functional measures. Data from a large sample-size study would also be needed to further decompose and characterize the everyday behaviors that show strong correlations with neurocognition. Moreover, although our pilot sample included subjects with paranoid symptoms, selectivity bias of the approach should be assessed to clarify whether disorganized and paranoid patients are able to consent and participate, as this would affect the generalizability of the results. Consistent with findings from other video studies,134,135 our subjects reported little reactivity to the video approach. We cannot exclude the possibility that subjects avoided functional behaviors or underperformed when with ethnographers, although we did not see evidence of this in pilot subjects. In a larger study, selective sampling of videotaped hours (i.e., excluding the first hour from all sessions; excluding the first session for each subject) could be used to explore subject reactivity during different periods of videorecording. Other psychometric features of the CPIs can be further explored, including the variance of these measures in different settings and the day-to-day stability (i.e., test–retest) of the variables. Also, a large study sample could clarify the total observation time required to conclusively document CPI domains.
The video ethnography approach we used is labor-intensive and not feasible for routine research or clinical use at this point. We are continuing this work to explore the psychometric attributes mentioned above and the potential for adaptations. For instance, a variety of briefer observational techniques (e.g., structured observation instruments, in-vivo coding techniques, scan-sampling methods) have been developed in other fields and used in large studies or as outcome measures in controlled trials.136–139 For example, brief observational instruments140,141 have been used to explore whether the frequency of child and parent negative behavior in the home or clinic decreases after an intervention.142 The video ethnography method may also have uses in other research areas, such as autism and neurodegenerative disorders, where there is a need to link neurobiological findings to everyday behaviors. Findings on executive control in autism, for instance, can show conflicting results because of a lack of consensus about the construct itself and its real-world behavioral correlates.143 CPIs or related measures could be used to analyze naturalistic video data from autistic individuals in order to understand executive control in everyday contexts. The video ethnography approach could also be used with individuals with limited or no expressive communication, such as those with aphasia or nonverbal autism, or with limited insight into their condition, such as those with frontotemporal dementia.144 Overall, this approach holds promise for many areas of research that desire or require attention to the ecological validity of their measures and constructs.
1 : A video ethnography approach to assessing the ecological validity of neurocognitive and functional measures in severe mental illness: results from a feasibility study. Schizophr Bull 2011Medline, Google Scholar
2 : Longitudinal studies of cognition and functional outcome in schizophrenia: implications for MATRICS. Schizophr Res 2004; 72:41–51Crossref, Medline, Google Scholar
3 : Targeting schizophrenia research to patient outcomes. Am J Psychiatry 2006; 163:353–355Crossref, Medline, Google Scholar
4 : Assessment of community functioning in people with schizophrenia and other severe mental illnesses: a white paper based on an NIMH-sponsored workshop. Schizophr Bull 2007; 33:805–822Crossref, Medline, Google Scholar
5 : Delineating psychomotor slowing from reduced processing speed in schizophrenia. Cogn Neuropsychiatry 2008; 13:457–471Crossref, Medline, Google Scholar
6 : Cognitive patterns in subtypes of schizophrenia. Eur Psychiatry 2002; 17:155–162Crossref, Medline, Google Scholar
7 : Disorganization and reality distortion in schizophrenia: a meta-analysis of the relationship between positive symptoms and neurocognitive deficits. Schizophr Res 2010; 121:1–14Crossref, Medline, Google Scholar
8 : Is executive impairment associated with schizophrenic syndromes? a meta-analysis. Psychol Med 2009; 39:1–12Crossref, Google Scholar
9 : Are psychotic psychopathology and neurocognition orthogonal? a systematic review of their associations. Psychol Bull 2009; 135:157–171Crossref, Medline, Google Scholar
10 : Endophenotypes: bridging genomic complexity and disorder heterogeneity. Biol Psychiatry 2009; 66:988–989Crossref, Medline, Google Scholar
11 : A review of cognitive training in schizophrenia. Schizophr Bull 2003; 29:359–382Crossref, Medline, Google Scholar
12 : Childhood videotaped social and neuromotor precursors of schizophrenia: a prospective investigation. Am J Psychiatry 2004; 161:2021–2027Crossref, Medline, Google Scholar
13 : How to assess the clinical impact of treatments on patients, rather than the statistical impact of treatments on measures. Int J Methods Psychiatr Res 2011; 20:63–72Crossref, Medline, Google Scholar
14 : Natural problems of naturalistic video data, in Video-Analysis Methodology and Methods: Qualitative Audiovisual Data Analysis in Sociology. Edited by Knoblauch HRaab JSoeffner H-G. Oxford, UK, Peter Lang, 2005, pp 181–190Google Scholar
15 : Ecological momentary assessment. Annu Rev Clin Psychol 2008; 4:1–32Crossref, Medline, Google Scholar
16 : Research Methods: A Modular Approach. Belmont, CA, Wadsworth Publishing, 2008Google Scholar
17 : Current and emergent applications of neuropsychological assessment: problems of validity and utility. Prof Psychol Res Pr 1990; 21:171–176Crossref, Google Scholar
18 : Real-world cognitive—and metacognitive—dysfunction in schizophrenia: a new approach for measuring (and remediating) more “right stuff.” Schizophr Bull 2006; 32:310–326Crossref, Medline, Google Scholar
19 : Ecological validity of neuropsychological testing. Boca Raton, FL, CRC Press, 1996Google Scholar
20 : Ecological validity in neuropsychological assessment: a case for greater consideration in research with neurologically intact populations. Arch Clin Neuropsychol 2006; 21:327–337Crossref, Medline, Google Scholar
21 : Improving the ecological validity of executive functioning assessment. Arch Clin Neuropsychol 2006; 21:217–227Crossref, Medline, Google Scholar
22 : UCSD Performance-Based Skills Assessment: development of a new measure of everyday functioning for severely mentally ill adults. Schizophr Bull 2001; 27:235–245Crossref, Medline, Google Scholar
23 : The ecological validity of neuropsychological tests: a review of the literature on everyday cognitive skills. Neuropsychol Rev 2003; 13:181–197Crossref, Medline, Google Scholar
24 : A contextual study of daily living strategies in neurocognitively impaired adults with schizophrenia. Qual Health Res 2006; 16:1217–1235Crossref, Medline, Google Scholar
25 : Observing Children in Their Natural Worlds: A Methodological Primer. Mahwah, NJ, Lawrence Erlbaum, 2004Google Scholar
26 : The systematic observation of children: rediscovery and advances. Behav Assess 1989; 11:201–217Google Scholar
27 : Observing Interaction: An Introduction to Sequential Analysis, 2nd Edition. Cambridge, UK, Cambridge University Press, 1997Crossref, Google Scholar
28 : Conducting a functional analysis of behavior, in Behavioral Approach to Assessment of Youth With Emotional/Behavioral Disorders: A Handbook for School-Based Practitioners. Edited by Fiedler MJBCR. Austin, TX, Pro-Ed, 1996, pp 83–180Google Scholar
29 : Methodological issues in direct observation: illustrations with the Living in Familial Environments (LIFE) coding system. J Clin Child Psychol 1995; 24:193–203Crossref, Google Scholar
30 : The Stream of Behavior. New York, Appleton–Century–Crofts, 1963Google Scholar
31 : Gorillas in the Mist Houghton Mifflin Company, 1983Google Scholar
32 : Language environments of autistic and normal children matched for language age: a preliminary investigation. J Autism Dev Disord 1982; 12:43–55Crossref, Medline, Google Scholar
33 : A peek behind the fence: naturalistic observations of aggressive children with remote audiovisual recording. Dev Psychol 1995; 31:548–553Crossref, Google Scholar
34 : Observations of bullying and victimization in the schoolyard. Can J Sch Psychol 1998; 31:41–60Crossref, Google Scholar
35 : Analogue assessment of child behavior problems. Psychol Assess 2001; 13:36–45Crossref, Medline, Google Scholar
36 : Introduction to the special section on clinical applications of analogue behavioral observation. Psychol Assess 2001; 13:3–4Crossref, Medline, Google Scholar
37 : The veridicality of punitive childhood experiences reported by adolescents and young adults. Child Abuse Negl 2000; 24:411–423Crossref, Medline, Google Scholar
38 : Mother–child interactions in ADHD and comparison boys: relationships with overt and covert externalizing behavior. J Abnorm Child Psychol 1994; 22:247–265Crossref, Medline, Google Scholar
39 : Avoiding conflict: mothers as tacticians in the supermarket. Child Dev 1983; 54:233–240Crossref, Google Scholar
40 : Methodological issues in the direct observation of parent–child interaction: do observational findings reflect the natural behavior of participants? Clin Child Fam Psychol Rev 2000; 3:185–198Crossref, Medline, Google Scholar
41 : Assessing the quality of family observations: A comparative analysis. J Marriage Fam 1986; 48:361–373Crossref, Google Scholar
42 : Childhood depression and family interaction. J Clin Child Psychol 1995; 24:77–88Crossref, Google Scholar
43 : Observational measures of parent–child interactions: an introductory review. Child Adolesc Ment Health 2003; 8:136–143Crossref, Google Scholar
44 : Use of structured interviews and observational methods in clinical settings, in Child and Adolescent Psychiatry, 5th Edition. Edited by Rutter MBishop DVMPine DS. Oxford, England, Blackwell Publishing, Ltd., 2009Google Scholar
45 : Attributions and behavior in functional and dysfunctional marriages. J Consult Clin Psychol 1996; 64:569–576Crossref, Medline, Google Scholar
46 : The nuts-and-bolts of behavioral observation of marital and family interaction. Clin Child Fam Psychol Rev 1998; 1:195–213Crossref, Medline, Google Scholar
47 : A comparison of the National Ambulatory Medical Care Survey (NAMCS) measurement approach with direct observation of outpatient visits. Med Care 2004; 42:276–280Crossref, Medline, Google Scholar
48 : Illuminating the “black box:” a description of 4,454 patient visits to 138 family physicians. J Fam Pract 1998; 46:377–389Medline, Google Scholar
49 : How valid are medical records and patient questionnaires for physician profiling and health services research? a comparison with direct observation of patients visits. Med Care 1998; 36:851–867Crossref, Medline, Google Scholar
50 : Process-product research on teaching: a review of criticisms. Elem Sch J 1989; 89:253–300Crossref, Google Scholar
51 : Generalizable observation of the teaching process. Educ Psychol Meas 1982; 42:467–478Crossref, Google Scholar
52 : Implementation and child effects of teaching practices in Follow-Through classrooms. Monogr Soc Res Child Dev 1975; 40:1–133Crossref, Medline, Google Scholar
53 : Curriculum pacing: some empirical data in mathematics. J Curric Stud 1978; 10:75–81Crossref, Google Scholar
54 : Assessment of interpersonal problem-solving skills. Psychiatry 1990; 53:329–339Crossref, Medline, Google Scholar
55 : Patterns of maternal responding in postpartum mothers with schizophrenia. Infant Behav Dev 2008; 31:532–538Crossref, Medline, Google Scholar
56 : Mother–infant interaction in postpartum women with schizophrenia and affective disorders. Psychol Med 1999; 29:991–995Crossref, Medline, Google Scholar
57 : Engagement of patients with psychosis in the consultation: conversation analytic study. BMJ 2002; 325:1148–1151Crossref, Medline, Google Scholar
58 : Ethnomethodology’s Program. Soc Psychol Q 1996; 59:5–21Crossref, Google Scholar
59 : From naturally-occurring data to naturally-organized ordinary activities: comment on Speer. Discourse Stud 2002; 4:531–537Crossref, Google Scholar
60 : Video ethnography and ethnoarcheological tracking, in The Work and Family Handbook: Multi-Disciplinary Perspectives and Approaches. Edited by Pitt-Catsouphes MKossek EESweet S. Mahwah, NJ, Lawrence Erlbaum Associates, 2005, pp 387–410Google Scholar
61 : Ethnography: Principles in Practice, 2nd Edition. New York, Routledge, 1995Google Scholar
62 : Phenomenological ethnography in sociology and anthropology. Ethnography 2003; 4:275–288Crossref, Google Scholar
63 : Analysing work activities in face-to-face interaction using video, in Qualitative Methods. Edited by Silverman D. London, Sage, 1997, pp 183–200Google Scholar
64 : Body Movement and Speech in Medical Interaction. Cambridge, Cambridge University, 1986Crossref, Google Scholar
65 : Cognition in the Wild. Boston, MA, Massachusetts Institute of Technology, 1995Google Scholar
66 : “Natural” and “contrived” data: a sustainable distinction? Discourse Stud 2002; 4:511–525Google Scholar
67 : Causal explanation, qualitative research, and scientific inquiry in education. Educ Res 2004; 33:3–11Crossref, Google Scholar
68 : Methods to improve reliability of video-recorded behavioral data. Res Nurs Health 2009; 32:465–474Crossref, Medline, Google Scholar
69 : Mathematical dimensions of qualitative research. Symbolic Interact 2000; 23:399–402Crossref, Google Scholar
70 : Observational study of behavior: sampling methods. Behaviour 1974; 49:227–267Crossref, Medline, Google Scholar
71 . Detecting and describing preventive intervention effects in a universal school-based, randomized trial targeting delinquent and violent behavior. J Consult Clin Psychol 2000; 68:296–306Google Scholar
72 : Naturalistic observations in clinical assessment, in Advances in Psychological Assessment. Edited by McReynolds P. San Francisco, Jossey-Bass, 1975, pp 42–95Google Scholar
73 : Comparisons of behavior transactions between conduct-disordered children and their mothers in the clinic and at home. J Abnorm Child Psychol 1985; 13:169–183Crossref, Medline, Google Scholar
74 : Reactivity effects during naturalistic observation of distressed and nondistressed families. J Fam Psychol 1994; 8:354–363Crossref, Google Scholar
75 : A device for recording automatic audio tape recording. J Appl Behav Anal 1971; 4:151–156Crossref, Medline, Google Scholar
76 : Methodological issues in naturalistic observations: some problems and solutions for field research, in Behavior Change: Methodology, Concepts and Practice. Edited by Hamerlynck LAHandy LCMash EJ. Champaign, IL, Research Press, 1973, pp 7–67Google Scholar
77 : Reactivity to home observation: a comparison of audio recorded behavior with observers present or absent. J Appl Behav Anal 1975; 8:181–185Crossref, Medline, Google Scholar
78 : Recording children’s conversations at home and at nursery school: a technique and some methodological considerations. J Child Psychol Psychiatry 1979; 20:225–232Crossref, Medline, Google Scholar
79 : Time, context, and subjective experiences in schizophrenia. Schizophr Bull 1989; 15:233–244Crossref, Medline, Google Scholar
80 : Psychosis reactivity to cannabis use in daily life: an experience sampling study. Br J Psychiatry 2010; 196:447–453Crossref, Medline, Google Scholar
81 : Analytic strategies for understanding affective (in)stability and other dynamic processes in psychopathology. J Abnorm Psychol 2009; 118:195–202Crossref, Medline, Google Scholar
82 : Emotion in the daily lives of schizophrenia patients: context matters. Schizophr Res 2009; 115:379–380Crossref, Medline, Google Scholar
83 : Feasibility and validity of computerized ecological momentary assessment in schizophrenia. Schizophr Bull 2008; 34:507–514Crossref, Medline, Google Scholar
84 : Differential diagnosis of seizure disorders: a conversation analytic approach. Soc Sci Med 2007; 65:712–724Crossref, Medline, Google Scholar
85 : Negotiating “depression” in primary care: a qualitative study. Soc Sci Med 2009; 69:1137–1143Crossref, Medline, Google Scholar
86 Barnhill JW (ed): Approach to the Psychiatric Patient. Washington, DC, American Psychiatric Publishing, Inc., 2009Google Scholar
87 : Do people with schizophrenia who have objective cognitive impairment identify cognitive deficits on a self-report measure? Schizophr Res 2008; 105:156–164Crossref, Medline, Google Scholar
88 : Memory and attention performance in psychiatric patients: lack of correspondence between clinician-rated and patient-rated functioning with neuropsychological test results. J Int Neuropsychol Soc 2004; 10:623–633Crossref, Medline, Google Scholar
89 : A genome screen for quantitative trait loci influencing schizophrenia and neurocognitive phenotypes. Am J Psychiatry 2008; 165:1185–1192Crossref, Medline, Google Scholar
90 : Neurocognitive endophenotypes in a multiplex multigenerational family study of schizophrenia. Am J Psychiatry 2007; 164:813–819Crossref, Medline, Google Scholar
91 : Neurocognition as a stable endophenotype in bipolar disorder and schizophrenia. J Nerv Ment Dis 2006; 194:255–260Crossref, Medline, Google Scholar
92 : Cognitive performance and functional competence as predictors of community independence in schizophrenia. Schizophr Bull 2010; 36:381–387Crossref, Medline, Google Scholar
93 : Street Phenomenology: The Go-Along as Ethnographic Research Tool. Ethnography 2003; 4:455–485Crossref, Google Scholar
94 : Micro- and macro-measurement of family-systems concepts. J Fam Psychol 1989; 3:137–157Crossref, Google Scholar
95 : A comparison of a global and a microanalytic coding system: implications for future trends in studying interactions. Behav Assess 1989; 11:81–100Crossref, Google Scholar
96 : Assessing cognitive deficits in bipolar disorder: are self-reports valid? Psychiatry Res 2005; 136:43–50Crossref, Medline, Google Scholar
97 : Systematic comparison of subjective and objective measures of quality of life at 4-year follow-up subsequent to a first episode of psychosis. J Nerv Ment Dis 2004; 192:805–809Crossref, Medline, Google Scholar
98 : What are the functional consequences of neurocognitive deficits in schizophrenia? Am J Psychiatry 1996; 153:321–330Crossref, Medline, Google Scholar
99 : Executive functioning in schizophrenia: a thorough examination of performance on the Hayling Sentence Completion Test compared to psychiatric and non-psychiatric controls. Schizophr Res 2009; 114:84–90Crossref, Medline, Google Scholar
100 : An empirical method for assessing social problem-solving in schizophrenia. Behav Modif 1995; 19:267–289Crossref, Medline, Google Scholar
101 : Evaluation of social problem-solving in schizophrenia. J Abnorm Psychol 1994; 103:371–378Crossref, Medline, Google Scholar
102 : Evaluation of problem-solving and communication skills of persons with schizophrenia. Psychiatry Res 1998; 78:197–206Crossref, Medline, Google Scholar
103 : Using the MATRICS to guide development of a preclinical cognitive test battery for research in schizophrenia. Pharmacol Ther 2009; 122:150–202Crossref, Medline, Google Scholar
104 : A comparison of qualitative scoring systems for the Rey-Osterrieth Complex Figure Test. Clin Neuropsychol 1997; 11:381–390Crossref, Google Scholar
105 : Spatial working memory and problem-solving in schizophrenia: the effect of symptom stabilization with atypical antipsychotic medication. Psychiatry Res 2008; 160:316–326Crossref, Medline, Google Scholar
106 : Disentangling the prefrontal network for rule-selection by means of a non-verbal variant of the Wisconsin Card-Sorting Test. Hum Brain Mapp 2009; 30:1734–1743Crossref, Medline, Google Scholar
107 : Organizational strategies mediate nonverbal memory impairment in obsessive-compulsive disorder. Biol Psychiatry 1999; 45:905–916Crossref, Medline, Google Scholar
108 : The construct validity of the Tower of London DX as a measure of the executive functioning of ADHD children. Assessment 1998; 5:215–226Crossref, Medline, Google Scholar
109 : The Independent Living Scales as a measure of functional outcome for schizophrenia. Psychiatr Serv 2004; 55:1052–1054Crossref, Medline, Google Scholar
110 : Reliability and validity of a scoring system for measuring organizational approach in the Complex Figure Test. J Clin Exp Neuropsychol 2000; 22:640–648Crossref, Medline, Google Scholar
111 : Specific cognitive deficits and differential domains of social functioning impairment in schizophrenia. Schizophr Res 2006; 81:227–238Crossref, Medline, Google Scholar
112 : Social cognition in schizophrenia. Psychol Bull 1997; 121:114–132Crossref, Medline, Google Scholar
113 : The Schizophrenia Patient Outcomes Research Team (PORT): updated treatment recommendations, 2009. Schizophr Bull 2010; 36:94–103Crossref, Medline, Google Scholar
114 : Social cognition in schizophrenia: an NIMH workshop on definitions, assessment, and research opportunities. Schizophr Bull 2008; 34:1211–1220Crossref, Medline, Google Scholar
115 : Delusional discourse: an investigation comparing the spontaneous causal attributions of paranoid and non-paranoid individuals. Psychol Psychother 2004; 77:525–540Crossref, Medline, Google Scholar
116 : Daily time use as a measure of community adjustment for persons served by assertive community treatment teams. Am J Occup Ther 2003; 57:558–565Crossref, Medline, Google Scholar
117 : Time use of unemployed and employed single male schizophrenia subjects. Schizophr Bull 1996; 22:659–669Crossref, Medline, Google Scholar
118 : How neurocognition and social cognition influence functional change during community-based psychosocial rehabilitation for individuals with schizophrenia. Schizophr Bull 2007; 33:1247–1256Crossref, Medline, Google Scholar
119 : Social functioning and neurocognitive deficits in outpatients with schizophrenia: a 2-year follow-up. Schizophr Res 1999; 37:13–20Crossref, Medline, Google Scholar
120 : A pilot validation study of a new measure of activity in psychosis. Soc Psychiatry Psychiatr Epidemiol 2005; 40:905–911Crossref, Medline, Google Scholar
121 : A validation of a new measure of activity in psychosis. Schizophr Res 2006; 85:288–295Crossref, Medline, Google Scholar
122 : Executive functions and the natural habitat behaviors of children with autism. Autism 2002; 6:365–381Crossref, Medline, Google Scholar
123 : The intricate relationship between monitoring and control in metacognition: lessons for the cause-and-effect relation between subjective experience and behavior. J Exp Psychol 2006; 135:36–69Crossref, Google Scholar
124 : Theory of Mind in first-episode schizophrenia patients: correlations with cognition and personality traits. Schizophr Res 2010; 119:115–123Crossref, Medline, Google Scholar
125 : Neurocognitive predictors of financial capacity across the dementia spectrum: normal aging, mild cognitive impairment, and Alzheimer’s disease. J Int Neuropsychol Soc 2009; 15:258–267Crossref, Medline, Google Scholar
126 : Time use among people with psychiatric disabilities: implications for practice. Psychiatr Rehabil J 2009; 32:177–191Crossref, Medline, Google Scholar
127 : Time use of people living with schizophrenia in a North London catchment area. Br J Occup Ther 2003; 66:46–54Crossref, Google Scholar
128 : A weighted time budget approach for the assessment of cognitive and social activities. Soc Psychiatry Psychiatr Epidemiol 1993; 28:184–188Crossref, Medline, Google Scholar
129 : Quality of life and social integration of severely mentally ill patients: a longitudinal study. J Psychiatry Neurosci 1997; 22:249–255Medline, Google Scholar
130 : Rehabilitation indicators: their nature and uses of assessment, in Rehabilitation Client Assessment. Edited by Bolton BCook D. Baltimore, MD, University Park Press, 1980, pp 102–117Google Scholar
131 : A taxonomy for goal-setting in the care of persons with dementia. J Gen Intern Med 1998; 13:675–680Crossref, Medline, Google Scholar
132 : The ‘‘what’’ and ‘‘why’’ of goal pursuits: human needs and the self-determination of behavior. Psychol Inq 2000; 11:227–268Crossref, Google Scholar
133 : Testing a self-determination theory process model for promoting glycemic control through diabetes self-management. Health Psychol 2004; 23:58–66Crossref, Medline, Google Scholar
134 : Using video-recorded consultations for research in primary care: advantages and limitations. Fam Pract 2000; 17:422–427Crossref, Medline, Google Scholar
135 : Reactions of patients to video recording of consultations in general practice. Br Med J (Clin Res Ed) 1985; 291:315–317Crossref, Medline, Google Scholar
136 : Can instructional and emotional support in the first-grade classroom make a difference for children at risk of school failure? Child Dev 2005; 76:949–967Crossref, Medline, Google Scholar
137 Observational record of the caregiving environment. Charlottesville, VA, National Institute of Child Health and Human Development Early Child Care Research Network, 1996Google Scholar
138 : Building a science of classrooms: Application of the CLASS framework in over 4,000 U.S. early-childhood and elementary classrooms. New York, NY, Foundation for Child Development, 2007Google Scholar
139 : Conceptualization, measurement, and improvement of classroom processes: standardized observation can leverage capacity. Educ Res 2009; 38:109–119Crossref, Google Scholar
140 : The dyadic parent–child interaction coding system: standardization and validation. J Consult Clin Psychol 1981; 49:245–250Crossref, Medline, Google Scholar
141 McMahon RJ, Forehand SLF: Helping the Noncompliant Child: Family-Based Treatment for Oppositional Behavior. New York, Guilford, 1981Google Scholar
142 : The long-term effectiveness and clinical significance of three cost-effective training programs for families with conduct-problem children. J Consult Clin Psychol 1989; 57:550–553Crossref, Medline, Google Scholar
143 : Understanding executive control in autism spectrum disorders in the lab and in the real world. Neuropsychol Rev 2008; 18:320–338Crossref, Medline, Google Scholar
144 : Repetitional responses in frontotemporal dementia discourse: asserting agency or demonstrating confusion? Discourse Stud 2010; 12:465–500Crossref, Google Scholar