Reward Sensitivity (Behavioral Activation System), Cognitive, and Metacognitive Control in Gambling Behavior: Evidences From Behavioral, Feedback-Related Negativity, and P300 Effect
Abstract
The present research explored the main factors able to influence the subjects’ choices in the case of decisions and distinguish between high- and low-risk decisions. Behavioral responses (Iowa Gambling Task [IGT]), meta-cognitive strategy, and event-related potential (feedback-related negativity [FRN] and P300) effects were used as predictive markers of gambling behavior. Behavioral activation system (BAS)-reward measure was applied to distinguish between high-BAS and low-BAS. It was found that higher-BAS opted in favor of the immediate reward, with a concomitant dysfunctional metacognition of their strategy. Finally, a consistent “reward bias” affected the high-BAS performance reducing the P300 and FRN in response to unexpected (loss) events. The cortical localization (sLORETA) of ERPs showed the contribution by distinct anterior and posterior areas.
One of the most important challenges of our life is to take control of our decisions. Decisions require distinguishing between high- and low-risk options and calibrating the choice of each decision made. Previous research tried to explore the main factors able to influence the subjects’ choices and strategies in the case of decisions: the ability to distinguish between high- and low-risk situations and the learning effect based on the previous experience was tested using typical decisional task such as the Iowa Gambling Task (IGT).1–3
Indeed, in IGT participants are presented with four decks from which to select cards to try to win money. Two of the decks are disadvantageous, with a general loss, because they present not only larger rewards but also occasional large losses. In contrast, advantageous decks result in a gain, because they present smaller rewards but also smaller losses. Generally, high-risk options imply the chance of a great reward but also high risk to have a loss. By contrast, low-risk options are often characterized by a lower reward but also low risk to have a loss. Thus, low-risk options often entail better long-term strategy with a final gain, despite the initial reduced short-term gain. Some caveats were revealed by the IGT. Although the IGT has achieved prominence, it is not without its critics. Criticisms have been raised over both its design and its interpretation. For example, research by4 found some confounding results embedded in the original design of IGT, and this confound made IGT serial studies misinterpret the effect of gain-loss frequency as final-outcome for somatic marker hypothesis. Moreover, some alternative tasks found reverse finding of IGT.5 Finally, Dunn and colleagues6 considered the role of IGT to support the somatic marker hypothesis, pointing out some limitations to the experimental paradigm.
However, in general IGT was found to be sensitive to gambling, especially in relationship with rewarding mechanisms. Specifically, some types of patients, for example those with deficits to ventromedial prefrontal cortex (VMPFC), appear unable to learn about which option in IGT is associated with long-term win strategy.7,8 That is they opt in favor with immediate reward, without considering the long-term functional strategy. Moreover, healthy subjects were also tested in order to elucidate the individual differences that influence the decisional processes, making their strategies more or less advantageous.
Indeed, in previous research it was found that some of the main factors capable of influencing gambling behavior are the reward system impairment on one hand and executive function deficits on the other. From the neuroanatomical point of view, it was demonstrated that VMPFC is a key structure in the decisional processes, it being critical for the executive functions (such as inhibition, planning, and cognitive flexibility), and for processing emotional and motivational information related to reward, in which more medial structures (such as insular cortex and cingulate cortex) are relevant.9
However, little is known about individual differences in reward mechanisms and the role of the executive functions, mediated by frontal system, with particular reference to gambling behavior. For this reason, it is relevant to better explore the contribution of some motivational component in mediating functional or dysfunctional reward mechanism, such as the behavioral inhibition system (BIS) and behavioral activation system (BAS), which measure represents an usable tool to test this reward-sensitivity.10–17 Second, we intended to directly monitor the role of some executive cognitive and metacognition in gambling behavior.
Gray’s model has tried to explain the behavioral motivational responses based on BAS/BIS dichotomy.18 The BAS system should be responsible for both approach and active behaviors; emotions associated with these behaviors generally induce the subject to approach an event/object that has generated the emotional response. The BAS is conceptualized as a motivational system that is sensitive to signals of reward, nonpunishment, and that is important for engaging behavior toward a reward or away from a threat. Moreover, BAS has been associated with feelings of optimism and aggression,19,20 whereas extreme levels of BAS have been linked to impulsivity disorders. Conversely, highly sensitive BIS people inhibit behavior in response to stimuli that are novel, innately feared, and conditioned to be aversive. The aversive motivational system is responsive to nonreward, avoiding negative experience negative or painful outcomes. Thus, the BIS is conceptualized as an attentional system that is sensitive to cues of punishment, nonreward, andfunctions that interrupt ongoing behavior in order to facilitate the processing of these cues in preparation for a response. In the BIS framework, “inhibition” refers to the abrogation of behavior in reaction to an expected or unexpected stimulus.21,22 Higher BIS activation is associated with enhanced attention, arousal, vigilance, and anxiety, and very strong BIS measure corresponds to anxiety-related disorders,23 whereas very weak BIS measure relates to primary psychopathy.24 Gray also held that BIS functioning is responsible for the experience of negative feelings such as fear and anxiety in response to these cues.25,26
Both activation and inhibition motivations are paralleled by the reward and punishment contingencies, and the IGT is argued to be capable of indexing punishment-reward conditions. We pointed out BAS was conceptualized as a motivational system that is sensitive to signals of reward and nonpunishment, engaging behavior toward a reward and away from a loss. Reward serves as a positive reinforcement for action (approach behavior), whereas punishment promotes negative reinforcement for avoidance (withdrawal). It was also emphasized that, whereas a normal level of BAS positively affects the emotional positive attitudes, extreme levels have been linked to impulsivity disorders, and extreme levels of BIS induce anxiety-related disorders.23,24
Therefore, insensitivity for punishment together with strong reward dependence results in a disadvantageous pattern of decision-making. More reward-dependent subjects should make more risky, disadvantageous choices on the IGT.27,28 BAS construct, specifically BAS-reward subscale, is supposed to be able to distinguish subjects who are potentially more reward-dependent. However, no previous study directly considered the significance of Carver and White’s BIS/BAS measures for gambling behavior, by comparing the high- versus low-BAS construct and specifically BAS-reward subscale, with IGT performance.
In regard to the executive functions and metacognition, it was shown that impaired working memory can lead to poor decision-making capacity, with a consequential inability to plan the best long-term strategy, to inhibit the immediate reward-seeking, and to organize a functional behavioral response.9,29 In particular, these functions under uncertain conditions, flexibility and adaptation in behavior were required to preserve the processing of consequences of previous decisions and actions.30
Recently, some research contributed to clarify the role of cognition and metacognition in gambling behavior, and some specific ERP effects, such as the FRN and P300 effect, were considered the neurocognitive correlates of decisional behavior in case of both functional and dysfunctional conditions.
The first ERP effect is a typical mediofrontal negativity, peaking at about 200–350 ms after the onset of the feedback stimulus that signals an unfavorable compared with favorable outcome.31–34 It is involved in performance monitoring, and it was observed that it is probably cortically generated near the MFC, mainly the anterior cingulate cortex (ACC).35 In addition, processing underlying the FRN are triggered by phasic dopaminergic signals, which code reward prediction error. These prediction error signals may then be conveyed to the ACC where they lead to adjustments in subsequent action selection and FRN production as an ERP effect.36
A second relevant ERP deflection, the P300, was used to explore the impairment of the executive functions in decisional processes that is the difficulty in updating the incoming contextual information. The P300 is the ERP component commonly investigated during feedback processing. Peaking around 300–600 ms after stimulus onset at posterior recording sites, P300 has been shown to be sensitive to the significance and occurrence probability of a stimulus37,38 as well as task complexity.39 The increasing amplitude of this positive deflection might represent the necessity to restore adjunctive information to updating the context31,40,41 when an unattended event is observed. Thus, it was found that more unexpected outcomes (as in case of losses) generated an increased P300 in comparison with more expected (gains) outcomes.
Therefore, taken together these two ERP measures should signal the increased inability to adopt an adequate cognitive strategy in response to a decisional context. Thus, we expected that more high-BAS subjects show inability to reward predictor error monitoring mainly in case of loss options. It would be signaled by the absence of FRN increased amplitude in response to these negative outcomes. Second, the inability to update the context typically related to unattended conditions could induce a substantial equivalence of the P300 amplitude in response to gains and losses. These ERP effects should be explained by a bias in reward sensitivity and a concomitant deficit in strategic planning.
A second hypothesis was related to a general worse performance by high-BAS in comparison to low-BAS, mainly in the case of high BAS-reward subscale. Indeed, these subjects should be less able to control their strategy during IGT, being oriented by a rewarding-bias in their behavioral options at IGT.
Third, these impairment in decisional processes should be also supported by a general absence of a coherent meta-representation about the subject’s his own strategy. The analysis of the self-knowledge of the incoming behavioral strategy across the trials should reveal this lack and poor representation. Specifically, we aim to explore the concomitant deficit in the metacognitive skills related to the subjective representation about the pianification, flexibility, efficacy, and self-awareness of their own strategy. Finally, these features should be directly related to the cognitive (behavioral) and ERP (FRN/P300) measures.
Methods
Subjects
Twenty-two healthy volunteers took part in the study (10 women, age range 19–25, M=23.78, SD=2.60). They were undergraduate students of the Catholic University of Milan, all right-handed and with normal or corrected-to-normal visual acuity. In a preliminary phase of the research, two expert clinicians applied a Structured Clinical Interview and evaluated the general psychopathological profiles of the subjects and their direct family members. No neurological or psychiatric pathologies were observed based on responses to the interview for DSM-IV, (SCID 199742; Beck Depression Inventory, BDI-II 199643), for the subjects or immediate family. Also, the absences of documented head injury or neurological disorders were considered based on the subjects clinical history. No payment was provided for their performance. They gave informed written consent for participating in the study, and the research was approved by the ethics committee of the institution where the work was carried out.
BIS/BAS Scores
BIS and BAS scores were calculated for each subject by using the Italian version of Carver and White Questionnaire (1994).44 It included 24 items (20 score-items and 4 fillers, each measured on 4-point Likert scale), and two total scores for BIS (range=7–28; items 7) and BAS (range=13–52; items 13). BAS also includes three subscales (reward, five items; drive, four items; and fun seeking, four items). The questionnaire was submitted to the subject after completing the experimental phase. Based on these measures, two total scores (BIS and BAS total) and three BAS subscale scores were calculated. The mean values and standard deviations (SDs) for each scale were for BIS: 19.63(3.16); BAS: 38.32(3.10); reward: 16.18(1.90); drive: 14.30(2.23); and fun seeking: 13.88(3.19), respectively. Finally, Cronbach’s alpha was calculated for BIS (0.88) and BAS (0.79) and separately for each BAS subscale (reward 0.87; drive 0.76, and fun seeking 0.77). Based on these subscale ratings we considered two subgroups of subjects: high-BAS and low-BAS subjects. The first group includes subjects with high BAS Reward subscale (more than 18, mean+1 SD); the second group includes subjects with low BAS Reward subscale (less than 14, mean −1 SD).
Iowa Gambling Task
The IGT was used1 (adaptation for an online Italian version). Subjects were seated in a comfortable chair in front of a PC screen (1280/1024 pixel). The PC was placed approximately 80 cm from the subject, with a visual horizontal angle of 4° and a vertical angle of 6°. They used a stimpad to choose the card and they had no restriction of time to respond, although they were required to make a decision within a brief time interval. After the experimental phase, the subjects were submitted to a debriefing phase, with the postevaluation questionnaire.
Behavioral and Metacognitive Measures
We subdivided the 100 cards selections into five blocks of 20 cards each. For each block, we counted the number of selections from decks A and B (disadvantageous) and the number of selections from decks C and D (advantageous), and then derived the net score for that block [(C+D) – (A+B)] (response index, rI).45
A postexperiment questionnaire was used to test the general self-knowledge of the cognitive strategy adopted during the task. It was composed of four questions: the general use of a planned strategy (“how much did you use a planned strategy during the task?,” metacognition of planning); the change of the strategy across the trials (“did you change your strategy during the task,” the metacognition about the flexible strategy); the sensation of a win/loss strategy (“how were you effective in your strategy during the task?,” the metacognition of efficacy); and the awareness of applying a strategy during the task (“were you aware of your strategy during the task?,” metacognition on the self-awareness). Each of these different aspects was explored by using a 5-point Likert scale, asking the subjects to quantify their behavior from 1 (total absence of that behavior) to 5 (presence in maximum extent of that behavior). To establish this degree, the subjects were invited to evaluate their behavior related to the total duration of the task (100 trials).
EEG Recordings and Data Reduction
EEG recordings were performed with a 64-channel DC amplifier (SYNAMPS system) and acquisition software (NEUROSCAN 4.2) during task execution. An ElectroCap with Ag/AgCl electrodes was used to record EEGs from active scalp sites referred to the earlobes (10/20 system of electrode placement46). Data were acquired using a sampling rate of 500 Hz, with a frequency band of 0.01 to 50 Hz. An off-line common average reference was successively computed to limit the problems associated with the signal-to-noise ratio.47 In addition, two EOG electrodes were sited on the outer canthi to detect eye movements. The impedance of the recording electrodes was monitored for each subject prior to data collection and was always below 5 kΩ. After performing EOG correction and visual inspection, only artifact-free trials were considered (rejected epochs, 3%; no differences between groups). The signal was visually scored, and portion of the data that contained artifacts were removed to increase specificity. Blinks were also visually monitored. Ocular artifacts (eye movements and blinks) were corrected using an eye-movement correction algorithm that employs a regression analysis in combination with artifact averaging.48 An averaged waveform (off-line) was obtained for each condition (not less than 25 epochs were averaged). The peak amplitude was quantified relative to the 100 ms pre-stimulus, and the onset was coincident with the appearance of the feedback on the monitor,49 taking into account the most negative peak and positive value within the two temporal windows of 150–300 and 300–400 ms post-stimulus, respectively.
Two peak profiles were calculated for the advantageous and disadvantageous decks, respectively, and distinct analyses were applied to each the average profiles. Subsequently, localization (four sites: frontal, central, temporoparietal, and occipital) and lateralization (three sides: left, central, and right) factors were considered in applying statistical analysis. Specifically, we measured left, central, and right frontal (F3, Fz, F4), middle-central (Cz, C3, C4), temporoparietal (P3/T7, Pz, P4/T8; the left and right localizations were obtained as the mean value of parietal and temporal sites) and occipital (Oz, O1, O2) brain activity. The mean latency of the two deflections was approximately 220 and 320 ms.
To localize the source of neural activity, we used the low resolution electromagnetic tomography (sLORETA) method.50,51 In the present study, we calculated the source localization for every subject and condition at the mean 220 and 310 peak (averaged across subjects and cortical sites). A voxel-wise nonparametric statistic implemented in sLORETA was used.
Results
Behavioral Measures
Two orders of data analysis were performed for behavioral and ERP measures. The behavioral measures were subjected to a two-way analysis of variance (ANOVA), in which the between-subjects factor (2, high-BAS and low-BAS) and the within-subjects factor (5, blocks) was applied to the rI (Table 1). Type I errors associated with inhomogeneity of variance were controlled by decreasing the degrees of freedom using the Greenhouse-Geiser epsilon. Figure 1 represents the rI as a function of group and block. Since gender effect was not significant at a preliminary analysis, we did not consider this factor in the successive analyses.
Measure | Metacognition | |
---|---|---|
High-BAS | Mean | SD |
Planning | 3.45 | 0.11 |
Flexibility | 2.88 | 0.13 |
Efficacy | 2.67 | 0.10 |
Self-knowledge | 2.96 | 0.15 |
Low-BAS | ||
Planning | 4.09 | 0.12 |
Flexibility | 3.41 | 0.13 |
Efficacy | 3.80 | 0.18 |
Self-knowledge | 3.93 | 0.15 |
TABLE 1. Mean and SD Values of the Metacognitive Measures as a Function of Group (High-BAS Versus Low-BAS)a
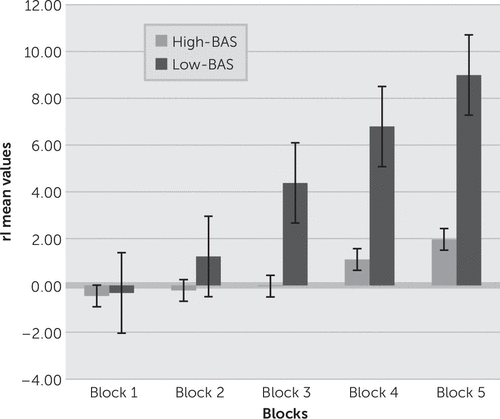
FIGURE 1. rI as a Function of Group (High-BAS Versus Low-BAS) and Block A significant rI increasing was observed for low-BAS across the five blocks in comparison with high-BAS.
As shown, a significant main effect was found for group (F(1,21)=10.98, p=0.001, η2=0.41), and group × block (F(4,21)=9.12, p=0.001, η2=0.39). Moreover, as revealed by post hoc analysis (contrast analysis, with Bonferroni corrections for multiple comparisons), increased rI was found for the second, third, fourth, and fifth block for low-BAS than high-BAS (all p<0.01). On the contrary, the first block did not show significant differences between the groups (F(1,21)=1.16, p=0.23, η2=0.12).
Metacognitive Measures
As reported by the postexperiment questionnaire, significant differences were found for the four dependent measures (planning; flexibility; efficacy; self-knowledge) (Table 1). The high-BAS group showed a significant impairment in reporting their strategy (F(1,21)=10.54, p=0.001, η2=0.41), considering the degree of flexibility (F(1,21)=10.11, p=0.001, η2=0.40), evaluating the efficacy (F(1,21)=9.33, p=0.001, η2=0.37), and being self-aware (F(1,21)=9.60, p=0.001, η2=0.38) of the strategy in comparison with low-BAS group.
ERP Data
Morphological analysis of ERPs showed two significant negative deflections within the 150–300 and 300–400 ms temporal window. The ERP data were subjected to a four-way mixed-design ANOVA, in which the between-subjects group (2, high-, low-BAS) and within-subjects condition (2, AD, advantageous, DD, disadvantageous), lateralization (3), and localization (4) factors were applied to the peak amplitude variable.
FRN.
Significant main effects were found for condition (F(1,21)=9.08, p=0.001, η2=0.37), localization (F(3,21)=7.70, p=0.001, η2=0.34), condition × group (F(1,21)=7.78, p=0.001, η2=0.36), and condition × group × lateralization (F(2,21)=7.32, p=0.001, η2=0.32). The other main or interaction effects were not statistically significant. Generally, the FRN effect was mainly increased in response to DD than AD and more anteriorly than centrally, temporoparietally, and occipitally distributed (all p<0.01). However, significant differences were found as a function of group: high-BAS showed decreased peak amplitude for DD than low-BAS. Moreover, whereas low-BAS subjects showed significant increased peak measure for DD in comparison with AD (F(1,21)=9.61, p=0.001, η2=0.39), no significant difference was found for high-BAS (F(1,21)=1.10, p=0.10, η2=0.10) (Figure 2). Finally, low-BAS showed an increased FRN in response to DD in frontal left more than central (F(1,21)=6.77, p=0.001, η2=0.31) and right (F(1,21)=7.13, p=0.001, η2=0.33) side (Figure 3).
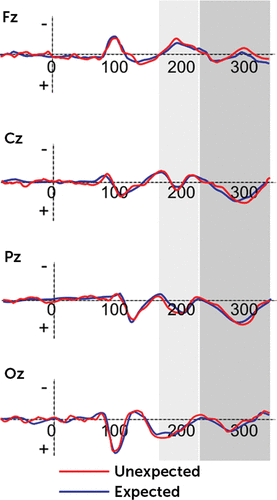
FIGURE 2. Mean Peak ERP Amplitude of FRN/P300 for High-BAS Group
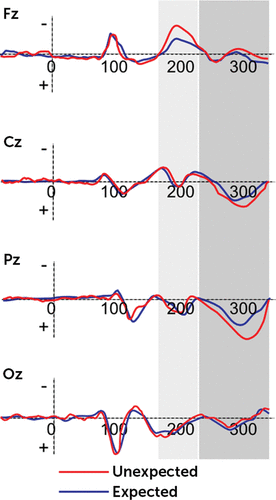
FIGURE 3. Mean Peak ERP Amplitude of FRN/P300 for Low-BAS Group
P300.
Significant main effects were found for condition (F(1,21)=11.13, p=0.001, η2=0.41), localization (F(3,29)=9.50, p=0.001, η2=0.39), and condition × group (F(1,21)=8.81, p=0.001, η2=0.39). On the contrary, the other main or interaction effects were not statistically significant. The P300 effect was mainly increased in response to DD than AD, and it was more temporoparietally than frontally, centrally, and occipitally distributed (all p<0.01). Moreover, as shown by post hoc comparisons, significant differences were found as a function of group: high-BAS showed decreased P300 peak amplitude for DD than low-BAS. However, both low-BAS and high-BAS subjects showed significant increased peak measure for DD in comparison with AD (respectively F(1,21)=8.98, p=0.001, η2=0.37; F(1,21)=9.01, p=0.001, η2=0.39) (Figures 2 and 3). Finally, both high-Bas and low-BAS showed an increased P300 within the parietal site.
Source Analysis
To estimate the localization of the source of the cortical differences for FRN/P300 deflections, sLORETA was performed comparing the two conditions (AD and DD) for each deflection. Significant differences were revealed for both the FRN and P300. The algorithm localized the source of this differential activation to the dorsolateral prefrontal cortex (t=4.91, p<0.01) (BA9, x=−4, y=45, z=15) and the anterior ACC (t=5.60, p<0.01) (BA24, x=4, y=31, z=8) for FRN effect; to the superior temporal gyrus (t=6.09, p<0.01) (BA39, x=53, y=−53, z=15) for P300 effect (Figure 4A–C). No other site was found to differentiate the two ERP effects as a function of the two conditions.
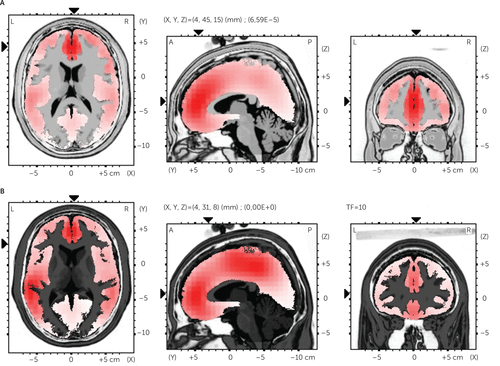
FIGURE 4. Results of the sLORETA Analysis The image shows the LORETA slices in Talairach space for the estimated source of activation differences (DD compared with AD) for the 220 (A and B) and 310 (C) ms.
Discussion
The main objective of the present study was to investigate the cognitive and neuronal correlates of decision-making during IGT in healthy individuals scoring high or low on reward sensitivity construct (BAS-reward). The following main results were found and discussed.
First, high-BAS subjects demonstrated a more dysfunctional behavior in choosing their strategy during IGT: they increased bad choices in comparison to low-BAS. This effect was observed during all the task duration (across the five blocks). This effect was also accompanied by a dysfunctional metacognitive representation of their strategy: high-BAS subjects were unable to evaluate and to reconstruct the cognitive strategy they adopted, in term of efficacy, flexibility, and self-knowledge. It was previously found that subjects with deficient mechanisms critical for decision-making, such as the ability to cognitively self-represent their own behavioral planning, are more susceptible to pursuing actions that are rewarding in the short term, even when these actions lead to deleterious consequences in the long term.45 A sort of reward-bias may explain both the bad strategy and the inability to correctly represent the performed behavior (poor metacognition).
Second, In regard to the ERP effects, significant differences were evidenced between the high-BAS and low-BAS. Feedback about win versus loss triggered a FRN in both groups, but in high-BAS this was unable to support a clear distinction between gain and losses based on the outcome feedback. Indeed the standard and normal FRN increasing related to unexpected (loss) options was partially absent in high-BAS group. A general FRN amplitude enhancement can be interpreted as indicator for outcomes worse than expected,36 and it marks the subject’s ability to correctly match the expected outcome (to have opted for the good card) with the external and real outcome (to have chosen a good or a bad card). The anomalous FRN profile for the high-BAS subjects would underline a significant deficit in their reward prediction.
In regard to the P300 effect, the observation of slightly larger P300 amplitudes after unexpected feedback in both low-BAS and high-BAS might index the general preserved ability to discriminate between more attended and unattended options, and the increased P300 could represent the stimulus salience and “novelty” when it is unexpected.37,52 However, it should be noted that high-BAS showed a decreased P300 in response to losses compared with low-BAS. This effect may be explained with a partial impairment in subjects’ attentional responsiveness to the less expected and unattended external condition.
Taking together these behavioral, metacognitive, and ERP results could suggest a general limitation in some executive functions, which are mainly related to the inability to adequately shift the personal cognitive strategy taking into account the bad previous behavioral outcomes. These results may also point out the difficulty in adequately directing their attention to the more relevant feedback (the bad behavior), as shown by the FRN effect. Moreover, the P300 effect could reinforce the FRN effect: the reduced “attentive” response toward the bad choices in high-BAS may have impaired the feedback control toward more dysfunctional choices.
In addition, also a reward-bias impact may be supposed to explain the present results. Specifically, the ERP cortical localization showed different brain generators for the two ERP effects. The more frontal and ventral localization of the FRN may show the direct relationship between this ERP negativity and the reward mechanisms. It could also explain a possible implication by ACC.53 In general, this frontal cortical network was found to be able to characterize the subjective behavior in response to decisional processes, when a gain/loss decision has to be taken. Specifically, the ventromedial and ACC contribution, as shown by sLORETA in case of FRN cortical generators, makes relevant the role of the reward system in managing the decisional processes.54 Therefore, the absence of a functional response by high-BAS could be explained by considering their reward-bias.
In contrast, in regard to the second ERP, the more posterior (parietal) distribution of P300 could directly link this deflection to the working memory functions.55 This posterior localization may also suggest that the P300 that we found is implicated in updating function about unattended information with respect of the previous knowledge.56 In the case of high-BAS, this specific function could be impaired. In addition, the posterior-parietal area which mainly contributed to generate the P300 effect might support the action planning, the control of the action significance for the overall behavioral strategy, and the production of a flexible shift of the planning as a function of the external requests. Also in this case, a significant impairment of these cognitive functions could be supposed in high-BAS.
To summarize, the present findings indicate that individuals scoring very high on reward component (BAS-reward) attribute higher motivational salience to immediate reward (losing immediate option) compared with more delayed (gaining delayed option) conditions. This is reflected in the reduction of behavioral functional choices, the absence of a coherent metacognitive representations, the reduction of the classic “enhanced effect” for FRN and P300 in case of unexpected (loss) choices. Our results clearly underlined that feedback and attentional mechanisms, mediated respectively by FRN and P300 deflections, act as cognitive regulators of the strategic behavior. Deficiencies concerning feedback mechanisms were apparent in those individuals (high-BAS) who focused on reward (reward bias) than individuals (low-BAS) who did not base their decisions on immediate reward. Thus, we propose to consider reward salience as an important aspect in feedback processes in subjects with high-risky attitudes. The present results also offer a valid background to explain the cortical and behavioral mechanisms underlying gambling behavior or drug addiction.27,28 Moreover, they potentially support specific treatment strategies to intervene to reduce the reward-bias effect in gambling behavior, by conditioning the subjects’ response to a delayed reward more than to an immediate gain. In parallel, they may suggest the utility to integrate this reward-related intervention to a more specific cognitive support, to induce a realistic representation of the situation based on a functional metacognitive strategy.
1 : Insensitivity to future consequences following damage to human prefrontal cortex. Cognition 1994; 50:7–15Crossref, Medline, Google Scholar
2 : Different contributions of the human amygdala and ventromedial prefrontal cortex to decision-making. J Neurosci 1999; 19:5473–5481Crossref, Medline, Google Scholar
3 : Affective judgment and beneficial decision-making: ventromedial prefrontal activity correlates with performance in the Iowa Gambling Task. Hum Brain Mapp 2006; 27:572–587Crossref, Medline, Google Scholar
4 : Immediate gain is long-term loss: Are there foresighted decision makers in the Iowa Gambling Task? Behav Brain Funct 2008; 4:13Crossref, Medline, Google Scholar
5 : Is deck B a disadvantageous deck in the Iowa Gambling Task? Behav Brain Funct 2007; 3:16. Available at doi:
6 : The somatic marker hypothesis: a critical evaluation. Neurosci Biobehav Rev 2006; 30:239–271Crossref, Medline, Google Scholar
7 : Subcortical and cortical brain activity during the feeling of self-generated emotions. Nat Neurosci 2000; 3:1049–1056Crossref, Medline, Google Scholar
8 : Distinct portions of anterior cingulate cortex and medial prefrontal cortex are activated by reward processing in separable phases of decision-making cognition. Biol Psychiatry 2004; 55:594–602Crossref, Medline, Google Scholar
9 : Impaired decision-making related to working memory deficits in individuals with substance addictions. Neuropsychology 2004; 18:152–162Crossref, Medline, Google Scholar
10 : BIS and BAS correlates with psychophysiological and cortical response systems during aversive and appetitive emotional stimuli processing. Motiv Emot 2012; 36:218–231Crossref, Google Scholar
11 : BIS/BAS, cortical oscillations and coherence in response to emotional cues. Brain Res Bull 2009; 80:151–157Crossref, Medline, Google Scholar
12 : BIS/BAS responses to emotional cues: Self-report, autonomic measure and alpha band modulation. Pers Individ Dif 2009; 47:858–863Crossref, Google Scholar
13 : Behavioral Inhibition, Behavioral Activation, and Affective Responses to Impending Reward and Punishment: The BIS/BAS Scales. J Pers Soc Psychol 1994; 67:319–333Crossref, Google Scholar
14 : The three arousal model: implications of gray’s two-factor learning theory for heart rate, electrodermal activity, and psychopathy. Psychophysiology 1980; 17:87–104Crossref, Medline, Google Scholar
15 : Electrodermal hyporeactivity and antisocial behavior: does anxiety mediate the relationship? J Affect Disord 2000; 61:177–189Crossref, Medline, Google Scholar
16 : A critique of Eysenck's theory of personality, in A model for personality. Edited by Eysenck HJ. Berlin, Springer-Verlag, 1981, pp 246–276Crossref, Google Scholar
17 : Uncertainty, neuromodulation, and attention. Neuron 2005; 46:681–692Crossref, Medline, Google Scholar
18 : Latent inhibition: the nucleus accumbens connection revisited. Behav Brain Res 1997; 88:27–34Crossref, Medline, Google Scholar
19 : Behavioral activation and inhibition in everyday life. J Pers Soc Psychol 2000; 78:1135–1149Crossref, Medline, Google Scholar
20 : The neuropsychology of anxiety: an enquiry into the functions of the septo-hippocampal system, 2nd ed. Oxford, Oxford University Press, 1987Google Scholar
21 : Electrodermal hyporeactivity and antisocial behavior: does anxiety mediate the relationship? J Affect Disord 2000; 61:177–189Crossref, Medline, Google Scholar
22 : Uncertainty, neuromodulation, and attention. Neuron 2005; 46:681–692Crossref, Medline, Google Scholar
23 : Attention deficit disorder and behavioral inhibition system: the relevance of the neuropsychological theory of Jeffrey A. Gray, in Attention deficit disorder: Criteria, cognition, intervention. Edited by Bloomingdale LM, Sergeant JA. Oxford, Pergamon Press, 1998, pp 117–125Google Scholar
24 : Validating a distinction between primary and secondary psychopathy with measures of Gray’s BIS and BAS constructs. J Abnorm Psychol 2005; 114:319–323Crossref, Medline, Google Scholar
25 : Framework for a taxonomy of psychiatric disorder, in Emotions: Essays on Emotion Theory. Edited by van Goozen SHM, Van de Poll E, Sergeant JA, Abingdon, United Kingdom, Psychology Press, 1994, pp 29–59Google Scholar
26 : Brain systems that mediate both emotion and cognition. Cogn Emotion 1987; 4:269–288Crossref, Google Scholar
27 : Reward-system effect (BAS rating), left hemispheric “unbalance” (alpha band oscillations) and decisional impairments in drug addiction. Addict Behav 2014; 39:1026–1032Crossref, Medline, Google Scholar
28 : Reward bias and lateralization in gambling behavior: BAS and alpha band analysis. Psychiatry Res 2014; (in press)Crossref, Medline, Google Scholar
29 : A somatic marker theory of addiction. Neuropharmacology 2009; 56(Suppl 1):48–62Crossref, Medline, Google Scholar
30 : Prefrontal cortex and drug abuse vulnerability: translation to prevention and treatment interventions. Brain Res Brain Res Rev 2011; 65:124–149Crossref, Google Scholar
31 : FRN and P300 ERP effect modulation in response to feedback sensitivity: the contribution of punishment-reward system (BIS/BAS) and behaviour identification of action. Neurosci Res 2010; 66:162–172Crossref, Medline, Google Scholar
32 : Veridical and false feedback sensitivity and punishment-reward system (BIS/BAS): ERP amplitude and theta frequency band analysis. Clin Neurophysiol 2010; 121:1502–1510Crossref, Medline, Google Scholar
33 : Lateralisation effect in comprehension of emotional facial expression: a comparison between EEG alpha band power and behavioural inhibition (BIS) and activation (BAS) systems. Laterality 2010; 15:361–384Crossref, Medline, Google Scholar
34 : Reward prediction error signals associated with a modified time estimation task. Psychophysiology 2007; 44:913–917Crossref, Medline, Google Scholar
35 : Decision-making in Blackjack: an electrophysiological analysis. Cereb Cortex 2007; 17:865–877Crossref, Medline, Google Scholar
36 : The neural basis of human error processing: reinforcement learning, dopamine, and the error-related negativity. Psychol Rev 2002; 109:679–709Crossref, Medline, Google Scholar
37 : Brain potentials associated with expected and unexpected good and bad outcomes. Psychophysiology 2005; 42:161–170Crossref, Medline, Google Scholar
38 : Problem gamblers exhibit reward hypersensitivity in medial frontal cortex during gambling. Neuropsychologia 2011; 49:3768–3775Crossref, Medline, Google Scholar
39 : On quantifying surprise: the variation of event-related potentials with subjective probability. Psychophysiology 1977; 14:456–467Crossref, Medline, Google Scholar
40 : P300 and stimulus categorization: two plus one is not so different from one plus one. Psychophysiology 1980; 17:167–178Crossref, Medline, Google Scholar
41 : P300 and tracking difficulty: evidence for multiple resources in dual-task performance. Psychophysiology 1980; 17:259–273Crossref, Medline, Google Scholar
42 : Structured Clinical Interview for DSM-IV Axis II Personality Disorders, (SCID-II). Washington, DC, American Psychiatric Press Inc., 1997Google Scholar
43 : Beck Depression Inventory II manual. San Antonio, TX, The Psychological Corporation, 1996Google Scholar
44 : Behavioral inhibition, behavioral activation, and affective responses to impending reward and punishment: The BIS/BAS scales. J Pers Soc Psychol 1994; 67:319–333Crossref, Google Scholar
45 : Decision-making and addiction (part I): impaired activation of somatic states in substance dependent individuals when pondering decisions with negative future consequences. Neuropsychologia 2002; 40:1675–1689Crossref, Medline, Google Scholar
46 : The ten-twenty electrode system of International Federation EEG. Electroencephalogr Clin Neurophysiol 1958; 10:371–375Google Scholar
47 : Using a common average reference to improve cortical neuron recordings from microelectrode arrays . J Neurophysiol 2008; 101:1679–1689Crossref, Medline, Google Scholar
48 : A solution for reliable and valid reduction of ocular artifacts, applied to the P300 ERP. Psychophysiology 1986; 23:695–703Crossref, Medline, Google Scholar
49 : An electrophysiological index of changes in risk decision-making strategies. Neuropsychologia 2013; 51:1397–1407Crossref, Medline, Google Scholar
50 : Standardized low-resolution brain electromagnetic tomography (sLORETA): technical details. Methods Find Exp Clin Pharmacol 2002; 24(Suppl D):5–12Medline, Google Scholar
51 : Low resolution electromagnetic tomography: a new method for localizing electrical activity in the brain. Int J Psychophysiol 1994; 18:49–65Crossref, Medline, Google Scholar
52 : ERP correlates of feedback and reward processing in the presence and absence of response choice. Cereb Cortex 2005; 15:535–544Crossref, Medline, Google Scholar
53 : Anterior asymetrical alpha activity predicts Iowa gambling performance: distinctly but reversed. Neuropsychologia 2004; 42:939–943Crossref, Medline, Google Scholar
54 : Reward encoding in the monkey anterior cingulate cortex. Cereb Cortex 2006; 16:1040–1055Crossref, Medline, Google Scholar
55 : Superior parietal cortex is critical for the manipulation of information in working memory. J Neurosci 2009; 29:14980–14986Crossref, Medline, Google Scholar
56 : Updating P300: an integrative theory of P3a and P3b. Clin Neurophysiol 2007; 118:2128–2148Crossref, Medline, Google Scholar