Corpus Callosum Pathology as a Potential Surrogate Marker of Cognitive Impairment in Diffuse Axonal Injury
Abstract
Diffuse axonal injury is a major form of traumatic brain injury. Neuropsychological assessments and high-resolution structural MRI were conducted using T1-weighted and diffusion tensor imaging. This study included 10 patients with diffuse axonal injury (all men, mean age 30.8±10.5 years) and 12 age- and sex-matched normal control participants. Patients with diffuse axonal injury had widespread volume reductions and lower fractional anisotropy in the corpus callosum (CC) compared with controls. Furthermore, cognitive processing speed was associated with reductions in white matter volume and fractional anisotropy in the CC. These findings suggest that CC pathology may be a potential surrogate marker of the cognitive deficits in these patients.
The pathology of diffuse axonal injury (DAI), a major form of traumatic brain injury (TBI), is characterized histologically by Wallerian-type axonal degeneration in the parasagittal white matter, corpus callosum (CC), and dorsal upper brainstem, resulting from shearing forces caused by sudden acceleration, deceleration, or rotation of the brain.1 Brain damage in DAI was originally thought to occur diffusely in the white matter.2
Advances in neuroimaging techniques have allowed the examination of structural brain alterations in patients with DAI. For example, voxel-based morphometry (VBM)3 has revealed the presence of brain-volume reductions in the frontal and temporal lobes, the cingulate, the CC, and in subcortical regions such as the thalamus and caudate in patients with DAI.4–6 These observations suggest that brain alterations in DAI are not restricted to the white matter, and that other specific brain regions are susceptible to injury as well. In addition, diffusion tensor imaging (DTI) studies have shown reductions in fractional anisotropy (FA), a measure of alterations in white matter microstructure, in various areas in patients with DAI, including the CC, internal/external capsule, and the superior/inferior longitudinal fascicles. Among these regions, the CC is thought to be especially vulnerable because robust FA reductions have been reported in this area.7–9
Symptoms after TBI primarily result from dysfunction in the damaged brain regions. Impairments in several cognitive domains are common after TBI, including memory and executive functions10 and the speed of information processing. Among these cognitive domains, impaired information processing speed is one of the most common deficits in patients with TBI.11 Information processing speed is a measure of the efficiency of cognitive function and is assessed using timed tests that challenge simple cognitive operations.12 This ability is a fundamental component of many cognitive functions.13 Studies in people with declining information processing speed, such as occurs in normal aging and some neuropsychiatric diseases, have suggested that white matter pathology is the neural basis of their impairments.14,15
The relationships between cognitive impairments and affected brain regions have been investigated in TBI. However, only a few studies have reported an association between neuropsychological performance and brain alterations in DAI. For example, volume reductions in gray matter structures were found to correlate with poor attention and memory deficits.16 In addition, studies have reported an association between white matter alterations (e.g., volume and microstructure) and cognitive functioning.17,18 However, to our knowledge, no study has comprehensively investigated the relationships between MRI measures derived from multiple neuroimaging modalities and cognitive impairments in DAI.
We hypothesized that a neural network comprising the CC may be associated with the cognitive impairments observed in DAI. This study aimed to assess structural brain damage in patients with chronic DAI and to examine the relationship between brain damage and cognitive impairments. We utilized VBM and DTI, along with neuropsychological assessments, to evaluate potential associations.
Methods
Participants
Participants were recruited from the outpatient clinic of the neuropsychology unit at the Kyoto University Hospital Department of Psychiatry. Inclusion criteria for patients were as follows: an injury sustained through significant trauma, loss of consciousness at the time of injury and >3 days of posttraumatic amnesia, a brain MRI scan showing possible diffuse pathology without large focal lesions, age >18 years, and occurrence of the injury at least 1 year before the study. Exclusion criteria were as follows: visual or visuoperceptual deficits, history of another TBI, history of drug abuse, or history of neurologic or psychiatric disorders before TBI onset. Predicted IQ was measured by the short-form Japanese Version of the National Adult Reading Test,19,20 which is considered to reflect the premorbid IQ of patients with TBI.
Ten male patients between 19 and 44 years old (mean age, 30.8±10.5 years) with TBI participated in this study. Table 1 shows the demographic and clinical information for these patients. All participants had severe TBI, according to criteria by Arlinghaus et al.21 The comparison group consisted of 12 normal individuals (all men, aged 29.8±6.3 years) matched to the TBI group for age and sex.
Patients with DAI (N=10) | Healthy Controls (N=12) | ||||
---|---|---|---|---|---|
Characteristic | Mean | SD | Mean | SD | p Value |
Age (years) | 30.8 (19–47) | 10.5 | 29.8 (21–57) | 6.3 | n.s. |
Male gender (N) | 10 | 12 | |||
Right-handedness (N) | 10 | 12 | |||
Education (years) | 11.3 (9–14) | 1.7 | 15.5 (12–18) | 1.5 | <0.01 |
Predicted IQ (JART) | 94.1 (67–112) | 14.4 | |||
Age at onset (years) | 21.8 | 8.7 | |||
Months from onset | 106.9 (12–262) | 79.4 | |||
Severity (PTA days) | 62.5 (3–150) | 40.4 | |||
Cause of injury (N) | |||||
Traffic accident | 8 | ||||
Fall | 2 |
TABLE 1. Demographic and Clinical Characteristics of Participantsa
This study was approved by the Committee on Medical Ethics of Kyoto University and was carried out in accordance with the Code of Ethics of the World Medical Association. Written informed consent was obtained after participants received a complete description of the study.
Procedures
Neuropsychological assessment.
Neuropsychological assessment was performed using the WAIS-III. Full IQ, verbal IQ, and performance IQ were calculated based on the results of the subtests. Four IQ indices (verbal comprehension, perceptual organization, working memory, and processing speed) were measured to assess specific cognitive functional domains.
MRI acquisition and preprocessing.
All participants underwent MRI scans on a 3T whole-body scanner with a 40-mT/m gradient and a receiver-only eight-channel phased-array head coil (MAGNETOM Trio; Siemens, Erlangen, Germany). The scanning parameters of the T1-weighted three-dimensional magnetization-prepared rapid gradient-echo (3D-MPRAGE) sequence were as follows: TR, 2,000 ms; TE, 4.38 ms; TI, 990 ms; field of view, 225×240 mm; matrix, 240×256; resolution, 0.9375×0.9375×1.0 mm3; and 208 total axial sections without intersection gaps.
DTI scanning parameters were as follows: TE, 96 ms; TR, 10,500 ms; matrix, 96×96; field of view, 192×192 mm; 70 continuous axial slices of 2.0-mm thickness; 81 noncollinear axis motion probing gradient; and b=1,500 s/mm2. The b=0 images were scanned before each set of nine diffusion-weighted images (90 volumes in total).
Data Processing and Statistical Analysis
Regional brain volume analysis.
The 3D-MPRAGE data were processed and analyzed using an extension of Statistical Parametric Mapping 8 (SPM8; Wellcome Department of Imaging Neuroscience, London, U.K.) and the VBM8.1 toolbox (written by Christian Gaser; http://dbm.neuro.uni-jena.de/vbm) running in MATLAB software (2012b; MathWorks, Natick, MA). A unified segmentation model22 was used, which combined both normalization and segmentation parameters in a single generative model. The outputs from the segmentation procedure were rigid-body aligned segmentations for each participant. Based on individually registered gray matter, white matter, and CSF segmentations, an average diffeomorphic anatomical registration through the exponentiated Lie algebra [DARTEL]23 template of all patients was created in MNI space. The output images of gray matter, white matter, and CSF partitions were resliced into 1×1×1-mm voxels. The voxel values of segmented and normalized gray and white matter images were multiplied (modulated) by the Jacobian determinants obtained from nonlinear normalization steps. The resultant gray and white matter images were smoothed with Gaussian kernels of 12-mm full width at half maximum, and all analyses were performed on these resulting images.
To identify the brain regions where patients with TBI showed reductions relative to controls, a two-sample t test was performed using SPM8. The effect of age was excluded from the data as a nuisance covariate. First, a liberal statistical threshold of p<0.001 (uncorrected) was applied to depict the overall pattern of brain alterations. Second, for representative clusters that were still significant with a more conservative threshold of p<0.05 (family-wise error [FWE]), with an extent threshold of 100 voxels, brain density values for the clusters (eigenvariates) were extracted using the volume-of-interest (VOI) function in SPM8 and were used in the analyses described below.
DTI processing.
All DTI data processing was performed using the programs in the FMRIB Software Library (FSL) (version 4.1.4; University of Oxford, Oxford, U.K.). All source data were corrected for eddy currents and head motion by registering all data to the first b=0 image with affine transformation. The FA maps were calculated using the FMRIB’s Diffusion Toolbox program. The Tract-Based Spatial Statistics program (version 1.2), which is part of the FSL program, was used to calculate an average FA value for the whole brain. First, FA data for all participants were normalized into a common space using the FNIRT nonlinear registration tool. Normalized FA images were averaged to create a mean FA image, which was then thinned to create a “skeleton,” taking only the centers of white matter tracts common to all participants. Voxel values of each participant’s FA map were projected onto the skeleton by searching the local maxima along the perpendicular direction from the skeleton. The mean skeleton FA for each participant was calculated using the fslstats program. We extracted the regional FA values of the VOIs for the white matter.
Correlation of Brain Alterations With Clinical Variables and Neuropsychological Scores
Correlations of extracted brain density data and FA values of the VOIs with clinical variables (using posttraumatic amnesia [PTA] as an index of the severity of injury) as well as each neuropsychological test score (verbal IQ, performance IQ, and four IQ indices [verbal comprehension, perceptual organization, working memory, and processing speed] in the WAIS-III) were investigated. Data were analyzed using SPSS 21.0 software (IBM Corp., Armonk, N.Y.). Statistical significance was defined as p<0.05 for the correlation analyses.
Results
Neuropsychological Assessment
Table 2 shows the means and standard deviations for the standard IQs and the WAIS-III IQ indices. Patients with DAI had IQs approximately >1 SD below the mean for the standard scores. The mean full IQ in patients with DAI was 79.0±19.0, and all IQ index scores were lower than the standard scores. Among the IQ index scores, only the processing speed index was >2 SD below the mean.
Assessment | Diffuse Axonal Injury (N=10) | |
---|---|---|
Mean | SD | |
Full IQ | 79.0 | 10.9 |
Verbal IQ | 82.4 | 12.9 |
Performance IQ | 76.6 | 10.4 |
Verbal comprehension | 82.4 | 13.5 |
Perceptual organization | 82.7 | 11.8 |
Working memory | 80.7 | 13.6 |
Processing speed | 62.6 | 13.6 |
TABLE 2. Neuropsychological Assessment Scoresa
Regional Brain Volumes and FA Reductions in Patients Relative to Controls
The patients with DAI exhibited gray matter reductions in widespread regions of the brain, including the temporal lobe, cingulate gyrus, subcortical regions, and cerebellum. Furthermore, patients with DAI showed significant white matter reductions in the CC and brainstem (uncorrected, p<0.001) (Figure 1 and Table 3). With FWE (p<0.05) and a 100-voxel extent threshold, the group differences were still significant for the left parahippocampal gyrus, bilateral amygdala, left insula, right putamen, and right thalamus. With this statistical threshold, the group difference was still significant for the splenium of the CC. We focused on this white matter cluster in the CC as the VOI in the subsequent DTI analysis (Figure 2). The patients showed lower FA in this VOI (in the splenium of the CC) compared with controls. Figure 3A shows a scatterplot of volume and FA in the splenium of the CC. White matter volume and FA values in the splenium correlated significantly in the DAI group (r=0.954, p<0.0001) but not in the control group (r=0.065, p=0.841).
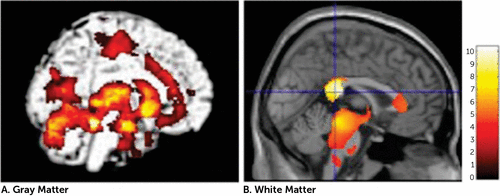
FIGURE 1. Regional Volume Reductions in Patients With Diffuse Axonal Injury Compared With Healthy Controls (Uncorrected, p<0.001)
Region | Cluster Center | Z Value | Cluster Size | ||
---|---|---|---|---|---|
X | Y | Z | |||
Gray matter | |||||
Parahippocampal gyrus | –18 | –40 | –18 | 5.94 | 45,057 |
Middle temporal gyrus | 46 | –58 | 4 | 4.61 | 106 |
Cingulate gyrus | 2 | 27 | 27 | 4.37 | 2,781 |
Precentral gyrus | –4 | –21 | 51 | 4.27 | 1,614 |
Supramarginal gyrus | 56 | –30 | 34 | 3.89 | 123 |
Inferior temporal gyrus | 52 | –33 | –24 | 3.87 | 104 |
Lateral occipital cortex | –40 | –70 | 13 | 3.76 | 101 |
Precentral gyrus | 28 | –28 | 57 | 3.68 | 370 |
Temporal pole | –36 | 15 | –23 | 3.59 | 220 |
Precentral gyrus | –30 | –28 | 54 | 3.55 | 121 |
White matter | |||||
Corpus callosum | 4 | –30 | 15 | 5.50 | 27,921 |
Cingulum | 15 | 24 | 42 | 4.79 | 1,607 |
Forceps minor | 12 | 38 | 10 | 4.23 | 2,506 |
Temporal lobe | 38 | –10 | –5 | 3.85 | 414 |
Brainstem | –3 | –33 | –51 | 3.43 | 208 |
Frontal lobe | –14 | 21 | 45 | 3.41 | 108 |
TABLE 3. Regions of Gray and White Matter Reductions in Patients With Diffuse Axonal Injury (Compared With Controls)
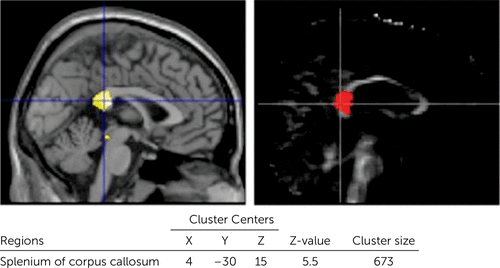
FIGURE 2. Extracted Region of Interesta
a [Left] Volume data using the volume of interest function. [Right] Fractional anisotropy data from the fractional anisotropy map (family-wise error, p<0.05; extent threshold, k<100).
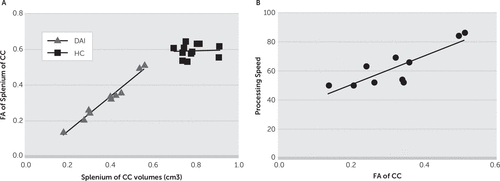
FIGURE 3. Fractional Anisotropy Valuesa
a [A] Scatterplot of volumes versus mean FA values for the cluster of voxels in the splenium of the CC. [B] Correlation between FA values for the CC versus processing speed scores. CC, corpus callosum; FA, fractional anisotropy.
Correlation Analyses for Brain Alterations and Cognitive Scores
The white matter density data and FA values were extracted from the cluster for the splenium of the CC in the DAI group. We calculated simple correlation coefficients for the MRI measures versus cognitive measures. Significant correlations were found between the processing speed index score in the WAIS-III and white matter density as well as FA values for the CC (processing speed versus white matter density: r=0.82, p=0.004; processing speed versus FA: r=0.85, p=0.002) (Figure 3B). There were no significant correlations between the other index scores or IQs and the MRI measures. Furthermore, PTA was not significantly correlated with CC morphological parameters (white matter density: r=−0.52, p=0.12; FA: r=−0.49, p=0.16).
Discussion
In this study, we investigated the relationship between brain alterations and cognitive impairments in patients with DAI using a combination of multiple structural imaging data. We found reductions in volume and white matter microstructure in the CC in the DAI group. These parameters were correlated with each other; patients with DAI with lower CC volumes showed greater reductions in CC FA values. In the neuropsychological tests, the DAI group showed impairments in all IQs and IQ index scores. The main finding was that impairment in processing speed in DAI correlated with structural alterations in the splenium of the CC.
Neuropsychological assessment revealed that patients with DAI exhibited reductions in all IQs and IQ index scores. Among the IQ index scores, only the processing speed was >2 SD below the standard mean. Processing speed has been most frequently investigated in participants with TBI, and impairments in this cognitive domain have been repeatedly reported.24–27 However, the injury types in the participants in the previous studies were heterogeneous; they included participants with various lesions. Our results suggest that if the injury type is specified as DAI, cognitive impairment is likely to be most prominent in the domain of processing speed. In this study, we used the IQ index score from the WAIS-III to measure processing speed. This method is one of several ways to measure processing speed. It is relatively complex, using the summed score from the digit symbol and symbol search subtests. Thus, in contrast with a simple task using reaction time, this index reflects abilities in working memory, executive functions, and sensory and psychomotor functions, in addition to processing speed per se.12 Furthermore, this index is specific to the visual modality. To investigate impairment in processing speed in patients with DAI in more detail, simple tasks (e.g., Part A of the Trail Making Test) or tasks using other modalities (e.g., Paced Auditory Serial Addition Test) are needed.
VBM analysis showed volume reductions in widespread regions, including the CC, in patients with DAI. These findings are largely in accordance with earlier VBM studies that showed changes in a variety of central subcortical areas.4–6,28 Regional volume reductions were most prominent in the splenium of the CC. Indeed, the CC is a region that is particularly vulnerable to shearing forces, or rotation of the brain due to trauma,2 and our result is consistent with previous studies.8,29,30
The patients with DAI showed lower FA, a measure of alterations in white matter microstructure, in the CC compared with controls. The FA findings were largely in accord with previous DTI studies in TBI.31–33 Axon damage is the major pathologic feature associated with reduced white matter microstructure after TBI.34 Our results revealed that the degree of reduction in FA value and the extent of volume reduction were strongly intercorrelated. This suggests that the remaining fibers are more severely damaged when the volume reduction is more severe. In mice, demyelination, remyelination, and excessive myelin are observed during white matter degeneration and recovery after TBI.35 Longitudinal studies are needed to elucidate the mechanisms underlying the strong relationship between FA values and fiber volumes in the CC observed in our study.
Significant correlations were found between the processing speed score in the WAIS-III and each of the MRI measures in the splenium of the CC. Similar relationships between FA in the splenium and processing speed were reported in children with DAI.36 Furthermore, a reduction in white matter microstructure in the CC appears to contribute to the age-related loss in cognitive processing speed.37 These observations, together with our present findings, suggest that CC pathological changes at least partially underlie the impairment in cognitive processing speed. Anatomically, the splenium of the CC contains pathways that connect the parieto-occipital regions of the two hemispheres.38 These regions are critical for visuospatial and attentional processes.39 Indeed, a functional MRI study showed that coordinated activity across multiple networks, including the parietal cortex, is essential for optimal cognitive processing speed.40 Thus, the disruption of these pathways may contribute to cognitive decline in patients with DAI by interrupting communication between critical cortical structures.
Similar to the severity and duration of loss of consciousness at onset, PTA serves as a marker of injury severity in TBI. However, we found no significant relationships between PTA and each of the MRI measures in the patients. The lack of a significant relationship may be attributable to the fact that our patient sample was in the chronic stage. This suggests that CC pathology in the chronic stage may not reflect injury severity in the acute stage. However, the clinical markers of injury severity (i.e., severity and duration of loss of consciousness at onset, and PTA) may be associated with pathology in other brain regions, such as the brainstem.
This study had several limitations. First, our sample size was small and did not include patients with mild TBI. Thus, this study should be regarded as preliminary. However, despite the small sample size, our results were statistically robust and agree with other clinical observations. Second, we focused on patients with chronic DAI. Consequently, we cannot generalize our findings to patients in the acute or subacute stage. Third, the premorbid IQ and educational level of two patients was low, although the mean premorbid IQ of the patient group as a whole was within the normal range. Because these patients were not able to finish high school because of brain injury, attributing the low premorbid IQ and educational level to the brain injury, and not to any preexisting low IQ, is reasonable. Fourth, because the neuropsychological assessment was conducted using only using the WAIS-III, we may not have assessed all aspects of the cognitive impairments. In addition, we extracted the IQ index score as a measure of processing speed. The processing index score of the WAIS-III is a complex measure using a score summed from a group of subtests. Thus, we cannot identify which aspects of processing speed are impaired. Further studies are needed using comprehensive and detailed tests to assess neuropsychological disturbances in TBI. We focused on structural changes in this study, although functional abnormalities are also found in the brains of patients with DAI. Thus, future studies combining structural and functional neuroimaging techniques are needed to clarify the neuropathological basis of cognitive impairment in patients with DAI.
1 : Current concepts: diffuse axonal injury-associated traumatic brain injury. Arch Phys Med Rehabil 2001; 82:1461–1471Crossref, Medline, Google Scholar
2 : Prospective comparative study of intermediate-field MR and CT in the evaluation of closed head trauma. AJR Am J Roentgenol 1988; 150:673–682Crossref, Medline, Google Scholar
3 : Voxel-based morphometry--the methods. Neuroimage 2000; 11:805–821Crossref, Medline, Google Scholar
4 : Traumatic brain injury and grey matter concentration: a preliminary voxel based morphometry study. J Neurol Neurosurg Psychiatry 2005; 76:984–988Crossref, Medline, Google Scholar
5 : Longitudinal changes in patients with traumatic brain injury assessed with diffusion-tensor and volumetric imaging. Neuroimage 2008; 42:503–514Crossref, Medline, Google Scholar
6 : A morphometric analysis of neuroanatomic abnormalities in traumatic brain injury. J Neuropsychiatry Clin Neurosci 2010; 22:173–181Link, Google Scholar
7 : Diffuse axonal injury: detection of changes in anisotropy of water diffusion by diffusion-weighted imaging. Neuroradiology 2003; 45:34–38Crossref, Medline, Google Scholar
8 : Diffuse axonal injury in severe traumatic brain injury visualized using high-resolution diffusion tensor imaging. J Neurotrauma 2007; 24:753–765Crossref, Medline, Google Scholar
9 : Diffusion tensor tractography-based analysis of the cingulum: clinical utility and findings in traumatic brain injury with chronic sequels. Neuroradiology 2014; 56:833–841Crossref, Medline, Google Scholar
10 : Cognitive and psychosocial outcome following moderate or severe traumatic brain injury. Brain Inj 1999; 13:489–504Crossref, Medline, Google Scholar
11 : INCOG recommendations for management of cognition following traumatic brain injury, part II: attention and information processing speed. J Head Trauma Rehabil 2014; 29:321–337Crossref, Medline, Google Scholar
12 : Information processing speed, in Encyclopedia of Clinical Neuropsychology. Edited by Kreutzer JS, DeLuca J, Caplan B. New York, Springer, 2011, pp 1317–1318Google Scholar
13 : When less is more and when more is more: the mediating roles of capacity and speed in brain-behavior efficiency. Intelligence 2009; 37:207–222Crossref, Medline, Google Scholar
14 : Brain white matter structure and information processing speed in healthy older age. Brain Struct Funct (Epub ahead of print, Aug 9, 2015)Medline, Google Scholar
15 : Functional connectivity changes and their relationship with clinical disability and white matter integrity in patients with relapsing-remitting multiple sclerosis. Mult Scler (Epub ahead of print, June 3, 2015)Google Scholar
16 : Regional cortical volume and cognitive functioning following traumatic brain injury. Brain Cogn 2013b; 83:34–44Crossref, Medline, Google Scholar
17 : Changes in white matter in long-term survivors of severe non-missile traumatic brain injury: a computational analysis of magnetic resonance images. J Neurotrauma 2005; 22:76–82Crossref, Medline, Google Scholar
18 : White matter integrity following traumatic brain injury: the association with severity of injury and cognitive functioning. Brain Topogr 2013; 26:648–660Crossref, Medline, Google Scholar
19 : Japanese Adult Reading Test (JART). Tokyo, Shinko-Igaku, 2007Google Scholar
20 : Estimation of premorbid IQ in individuals with Alzheimer’s disease using Japanese ideographic script (Kanji) compound words: Japanese version of National Adult Reading Test. Psychiatry Clin Neurosci 2006; 60:332–339Crossref, Medline, Google Scholar
21 : Neuropsychiatric assessment, in Textbook of Traumatic Brain Injury, 2nd ed. Edited by Silver JM, McAllister TW, Yudofsky SC. Washington, D.C., American Psychiatric Publishing, 2011, pp 5Crossref, Google Scholar
22 : Unified segmentation. Neuroimage 2005; 26:839–851Crossref, Medline, Google Scholar
23 : A fast diffeomorphic image registration algorithm. Neuroimage 2007; 38:95–113Crossref, Medline, Google Scholar
24 : Attentional control and slowness of information processing after severe traumatic brain injury. Brain Inj 2004; 18:257–272Crossref, Medline, Google Scholar
25 : Cognitive sequelae of diffuse axonal injury. Arch Neurol 2006; 63:418–424Crossref, Medline, Google Scholar
26 : Masking release, processing speed and listening effort in adults with traumatic brain injury. Brain Inj 2014; 28:1473–1484Crossref, Medline, Google Scholar
27 : Predictors of memory and processing speed dysfunctions after traumatic brain injury. Biomed Res Int 2014; 2014:129796Crossref, Medline, Google Scholar
28 : Cognitive sequelae of head injury: involvement of basal forebrain and associated structures. Brain 2005; 128:189–200Crossref, Medline, Google Scholar
29 : Diffuse axonal injury and traumatic coma in the primate. Ann Neurol 1982; 12:564–574Crossref, Medline, Google Scholar
30 : Traumatic brain injury: diffusion-weighted MR imaging findings. AJNR Am J Neuroradiol 1999; 20:1636–1641Medline, Google Scholar
31 : Diffusion tensor imaging during recovery from severe traumatic brain injury and relation to clinical outcome: a longitudinal study. Brain 2008; 131:559–572Crossref, Medline, Google Scholar
32 : White matter damage and cognitive impairment after traumatic brain injury. Brain 2011; 134:449–463Crossref, Medline, Google Scholar
33 : Mapping traumatic axonal injury using diffusion tensor imaging: correlations with functional outcome. PLoS One 2011; 6:e19214Crossref, Medline, Google Scholar
34 : Neuropathology of mild traumatic brain injury: relationship to neuroimaging findings. Brain Imaging Behav 2012; 6:108–136Crossref, Medline, Google Scholar
35 : Components of myelin damage and repair in the progression of white matter pathology after mild traumatic brain injury. J Neuropathol Exp Neurol 2015; 74:218–232Crossref, Medline, Google Scholar
36 : Diffusion tensor imaging in the corpus callosum in children after moderate to severe traumatic brain injury. J Neurotrauma 2006; 23:1412–1426Crossref, Medline, Google Scholar
37 : Cognitive processing speed in older adults: relationship with white matter integrity. PLoS One 2012; 7:e50425Crossref, Medline, Google Scholar
38 : Corpus callosal connection mapping using cortical gray matter parcellation and DT-MRI. Hum Brain Mapp 2008; 29:503–516Crossref, Medline, Google Scholar
39 : Hemispheric asymmetry in visuospatial attention assessed with transcranial magnetic stimulation. Exp Brain Res 2002; 143:426–430Crossref, Medline, Google Scholar
40 : Task-load manipulation in the Symbol Digit Modalities Test: an alternative measure of information processing speed. Brain Cogn 2013; 82:152–160Crossref, Medline, Google Scholar