Cognitive Performance in Subjects With Multiple Sclerosis Is Robustly Influenced by Gender in Canonical-Correlation Analysis
Abstract
The authors explored the relations between clinical/demographic characteristics and performance on a neuropsychological battery (eight tests) in a cohort (N=46) of multiple sclerosis (MS) subjects. Findings resulted from a secondary analysis of a study examining the relationships between imaging biomarkers in MS and cognitive tasks of executive functioning. The objective was to determine whether the overlapping test results could be judiciously combined and associated with clinical/demographic variables. Canonical-correlation analysis (CCA) was utilized, and it was found that differences between performance on untimed tests, and the sum of performance on timed Trail-Making Tests, Parts A and B, best matched clinical/demographic variables, and gender was the most important feature.
Multiple sclerosis (MS) is a neuroinflammatory disease that causes sensory and motor disturbances, diplopia, ataxia, bladder disturbance, fatigue, and cognitive dysfunction.1,2 Among these symptoms, cognitive impairments produced by MS, including impaired processing speed, attention, memory, and executive function, may adversely affect patients’ daily lives.3
A characteristic feature of MS is that more women are affected than men. In addition to prevalence, gender differences have been addressed in MS with respect to genetic susceptibility, clinical presentation, effects of sex hormones, immune system, and response to therapy.2 Previous studies have proposed several links between gender effects and neuroimaging findings, such as cortical atrophy, functional connectivity, and white matter changes.4–7 However, despite the clinical importance of cognition for overall well-being in the MS population, research on the direct association between gender and cognitive dysfunction has not been widely reported in MS.
Schoonheim et al.7 demonstrated that male subjects with MS performed more poorly than male controls in several cognitive domains, such as executive functioning, verbal memory, processing speed, working memory, attention, and psychomotor speed, yet these domains were relatively preserved in female subjects. They also observed a stronger correlation between subcortical volume and a cognitive marker (the average Z score of a battery of cognitive tests) in males with MS. In addition, significant white matter changes, such as lower fractional anisotropy, mean diffusivity, axial diffusivity, and radial diffusivity, in diffusion tensor imaging (DTI) data have been observed in male subjects with lower cognitive Z scores but not in female subjects whose cognition was relatively intact.6 In a functional magnetic resonance imaging (fMRI) study, male subjects demonstrated decreased resting-state functional connectivity, as well as lower network efficiency, in association with deteriorating cognitive performance,4 especially reduced visuospatial memory. While these studies focused on the relationship between cognitive dysfunction and functional/structural changes in the brain, less emphasis has been on the characterization of cognitive differences and gender effects in MS.
Several factors appear to be predictors of cognitive dysfunction in MS: early age at onset, male sex, secondary progressive course, neurodegeneration, and low baseline intelligence.8 Males are more likely to develop a severe disease course, including physical disability and cognitive impairments. In MS patients, it appears that cognitive dysfunction in females is less dependent on factors such as physical disability, while cognitive decline in males is correlated with Expanded Disability Status Scale (EDSS) score, age, disease duration, and education level.5 Another study found that female subjects performed better on the Mini-Mental State Examination, the Wisconsin Card Sorting Test (WCST), language assessment, and memory tests in the Repeatable Battery for the Assessment of Neuropsychological Status Update.9 Thus, males appear to be particularly cognitively vulnerable in MS.
The “clinical” assessment of cognitive function of individuals with MS must consider several factors, including but not limited to determining the priorities regarding which cognitive domains need to be assessed and possible physical limitations (i.e., motor difficulties in manipulating a pencil, vision problems such as oscillopsia), as well as the individual’s vulnerability to fatigue. The field of neuropsychology has addressed these issues with different testing approaches. Rao et al.10 created a 20-minute screening battery with a 71% sensitivity value and a 94% specificity value in its ability to identify cognitively impaired MS patients. Benedict et al.11 developed the Minimal Assessment of Cognitive Function in MS (MACFIMS) test battery, which distinguishes relapsing-remitting MS from secondary progressive MS. Verbal memory and executive function were most predictive of vocational status. This 90-minute battery includes an attentional working memory task (PASAT), a measure of processing speed (symbol digit modalities test), verbal and visual memory (CVLT II and BVMT-R), an executive sorting task (D-KEFS), a visual spatial task (JOL), and verbal fluency (COWAT). 3T MRI has shown that the MACFIMS subtests correlate with the number of cortical lesions, cortical volume, white matter lesions, and white matter volume.12 The Brief International Cognitive Assessment for MS (BICAMS) is another assessment tool for examining cognition in MS that has an international validation, has guidelines for defining significant change from repeated assessments, and is endorsed by the American Academy of Neurology as a cognitive measure for MS.13 This battery is completed in 15 minutes and includes the domains of information processing speed and verbal and visual memory.
In the present study, eight neuropsychological tests that employed executive function were administered to an MS cohort (13 males, 33 females). Since these tests all probe aspects of executive function, it is likely that performance on these individual tests will be related to (correlated with) one another, yet each test will provide some unique information. We aimed to determine whether there was an established overall pattern of neuropsychological deficits seen in MS and if this pattern of deficits could be predicted based on disease severity and other demographic factors, including gender. Our implicit hypothesis is that a combination of gender, age, education, and clinical indices will correspond with a combination of results seen in neuropsychological tests. We employed canonical-correlation analysis (CCA), a type of machine learning method used to identify patterns in large data sets. We show that a combination of demographic (i.e., gender, age, education, alone or in combination) and clinical (i.e., disease duration, severity of disability, affective states) indices accurately predict results of frontal lobe testing. When we investigated the specific combination of demographic factors that predicted neuropsychological test performance in MS, we found that gender had the greatest influence.
The results reported in the current study are from a larger study in which traditional tasks of executive functioning were administered as the test battery. The testing was not for clinical assessment purposes. Our original study solely assessed executive functioning and was not aimed at examining other cognitive domains such as memory or tasks found in MACFIMS or BICAMS. The test battery was selected for a specific research project that focused on executive functioning and the possible relationships between biomarkers in MS (i.e., myelin water, fMRI connectivity) and performance on neuropsychological tests that traditionally have been used to assess different aspects of executive function. The findings reported here represent a secondary analysis of the neuropsychological test results.
Materials and Methods
Participants
Forty-six relapsing-remitting MS subjects (13 males, 33 females) were enrolled in a study examining the relations between results of various MRI sequences (including DTI, myelin water imaging, lesion load, resting-state fMRI functional connectivity, and cortical thickness), cognitive performance, clinical measures, and demographic characteristics. For the purposes of the present study, we focused on clinical measures and demographic variables (Table 1), as the imaging will be the subject of another study. All the subjects fulfilled the McDonald criteria for MS diagnosis and were recruited from the MS clinic at the UBC Hospital. Referred individuals underwent a telephone screen (BK), and persons with significant untreated depression and/or other psychiatric illness, learning disabilities, history of drug or alcohol abuse, or steroid use in the last 3 months or those who showed evidence of an active flare were excluded from this study. Participants were also screened for adequate motor skills for their ability to manipulate a pencil, which would have direct impact on some of the tasks (i.e., coding and symbol search).
Variable | Female Mean±SD (Range) | Male Mean±SD (Range) |
---|---|---|
Demographics and clinical measures | ||
Age (years) | 41.1±10.3 (22–58) | 45.8±11.6 (26–60) |
Education (years)* | 15.3±2.2 (9–20) | 13.7±2.7 (8–18) |
Expanded Disability Status Scale | 2.3±1.6 (0–6) | 1.9±1.5 (0–5) |
Disease duration (months) | 129.0±86.2 (4–288) | 128.9±133.1 (12–432) |
Neuropsychological scores | ||
Wechsler Adult Intelligence Scale-IV digit spana | 9.0±2.5 (5–14) | 8.2±2.2 (4–12) |
Arithmetica | 9.0±2.6 (4–13) | 8.9±3.3 (4–16) |
Letter-number sequencinga | 9.6±2.7 (5–18) | 8.8±2.4 (5–14) |
Symbol searcha | 10.4±3.5 (3–18) | 9.2±3.2 (5–16) |
Codinga | 10.3±2.6 (5–15) | 9.0±2.1 (5–13) |
Working Memory Indexb | 93.7±12.4 (73–114) | 91.0±14.7 (73–114) |
Processing Speed Indexb | 101.9±16.0 (66–132) | 94.5±14.1 (72–117) |
Verbal Letter Fluency Testc | 41.0±10.7 (22–67) | 37.4±14.4 (12–62) |
Wisconsin Card Sorting Test errorsb | 93.1±13.7 (61–113) | 82.2±19.0 (55–121) |
Perseverative responsesb | 92.3±12.6 (62–112) | 86.2±23.6 (55–145) |
Perseverative errorsb | 91.1±12.9 (63–112) | 86.3±23.6 (55–145) |
Categories completed raw** | 5.5±1.1 (2–6) | 3.3±1.9 (1–6) |
Trials to complete first category raw** | 12.7±4.4 (10–33) | 38.6±38.3 (11–105) |
Failure to maintain set raw score | 0.5±0.8 (0–3) | 1.0±1.6 (0–5) |
Learning to learn raw score | N/Ad | N/Ad |
Trail-Making Test, Part A (raw score)* | 30.7±12.3 (18–80) | 40.3±13.3 (19–58) |
Z score | –0.7±1.5 (–5.7–1) | –1.2±1.4 (–3.1–0.87) |
Error (raw score) | 0.2±0.4 (0–2) | 0.1±0.3 (0–1) |
Trail-Making Test, Part B (raw score) | 74.2±61.0 (27–360) | 110.7±49.8 (44–216) |
Z score | –0.5±2.3 (–10.33–1.16) | –1.5±2.5 (–8.86–0.91) |
Error (raw score) | 0.4±1.0 (0–5) | 0.6±1.1 (0–3) |
Multiscore Depression Inventory total score (raw) | 31.9±22.4 (3–86) | 38.3±21.8 (8–75) |
T score | 47.3±8.9 (36–69) | 52.7±10.2 (38–70) |
State-Trait Anxiety Inventory stateb | 49.8±10.4 (35–81) | 50.2±9.7 (37–73) |
State-Trait Anxiety Inventory traitb | 54.6±12.5 (36–82) | 58.5±7.8 (48–72) |
Fatigue Severity Scale (raw score) | 38.2±15.2 (11–63) | 35.2±14.8 (9–55) |
TABLE 1. Demographics Characteristics, Clinical Measures, and Neuropsychological Scores
Ethics approval was issued by the University of British Columbia's Research Ethics Board, and all subjects provided signed informed consent.
Test Battery
As mentioned above, data collected for the original, larger study were not based on clinical assessments or interest in other cognitive domains such as memory. Therefore, test batteries used in assessments of MS populations such as MACFIMS or BICAMS were not utilized. The focus of the larger study was on executive functioning and the traditional tests utilized to measure aspects of this cognitive domain.
All subjects underwent eight psychometric measures assessing processing speed, working memory, executive function, and attention. This battery was not selected for clinical purposes. Tests included were the Wechsler Adult Intelligence Scale-IV (WAIS IV) subtests (digit span, arithmetic, letter number sequencing, symbol search, and coding), Verbal Letter Fluency Test, WCST, and Trail-Making Test, Parts A and B (TMT A and B). Composite index scores from the WAIS-IV were also obtained, including Working Memory Index (WMI), which utilized scores from digit span, arithmetic, and letter number sequencing subtests. The WAIS-IV Processing Speed Index (PSI) is based on the symbol search and Coding subtests. Clinical questionnaires were also administrated to examine affective status, which included the Multiscore Depression Inventory (MDI) and State-Trait Anxiety Inventory (STAI). The tests are summarized in Table 2. The other measure administered was a widely used fatigue measure in the MS field, the Fatigue Severity Scale (FSS). The duration of the assessment was approximately one hour. Raw cognitive scores were converted to standardized scores using published normative data that consider psychometric factors such as age, education, and gender. Disability rated by the Kurtzke EDSS was determined by a neurologist at the time of recruitment or scanning.
Test | Tested Ability |
---|---|
Wisconsin Card Sorting Task | Executive skills: concept formation, mental flexibility, perseveration detection |
Trails-Making Test, Parts A and B | Executive skills: attentional switching, sequencing |
Verbal Letter Fluency | Executive skills: spontaneous generation of information according to rules |
Working Memory Index (Wechsler Adult Intelligence Scale-IV subtests: digit span, arithmetic, and letter-number sequencing) | Executive skills: temporary holding and manipulation of information to formulate an answer |
Processing Speed Index (Wechsler Adult Intelligence Scale-IV subtests: coding and symbol search) | Processing skills: focused attention, visual scanning, and discriminating visual details under timed conditions |
TABLE 2. Administrated Tests and Corresponding Tested Abilities
Statistical Analyses
Two-sample t tests (two-tailed) were carried out with all the scores among males and females. Transformed scores were calculated in R (version 3.2.0), a language and environment for statistical computing, if normality did not hold. CCA14 was done in MATLAB (MathWorks) to determine whether demographic variables were related to cognitive scores. CCA was chosen to study complex multivariate data, and it can be considered one of the original “machine learning” algorithms that are now used to explore “big data” in many fields.15–17 The advantage of the CCA method is that it is an extension of regression that enables additional factors to be included. If we have two sets of correlated variables (e.g., the performance on the neuropsychological tests will likely be correlated, and some demographic factors such as disease duration and age will likely be correlated), CCA attempts to identify the linear combinations of these two sets of variables (i.e., vectors) that maximally correlate with each other. It is noteworthy that this will likely be a more powerful approach than regression where one could try and predict the neuropsychological performance on one test (i.e., in regression there is only one dependent variable).
In keeping with prior neuropsychological approaches, we analyzed timed and untimed tests separately. We performed CCA on cognitive scores divided into two groups (timed and untimed tests) and demographical variables. The timed group included four affective variables (MDI total t scores, STAI standardized state scores and trait scores, and FSS raw scores) and eight variables from the tests in which subjects were timed during performance, including three scaled scores in WAIS-IV (arithmetic, symbol search, and coding), Verbal Letter Fluency Test, and four measures of TMT (transformed TMT A scores, TMT A Z scores, transformed TMT B scores, and TMT B Z scores). Due to nonnormality, raw scores of TMT A and B were transformed by taking the square root of the reciprocal of the raw scores. The transformed score could be expressed as follows:
In the untimed group, four affective variables (same as timed group) and eight cognitive variables from the tests in which subjects were not timed were included. The eight cognitive variables were two scaled scores on WAIS-IV (digit span and letter number sequencing) and six measures on WCST (standard score of errors, perseverative response, perseverative errors, raw scores of categories completed, trials to complete first category, and failure to maintain set). Age, gender, education, EDSS scores, and disease duration were included as demographic variables in both groups.
Calculations were performed in leave-one-out fashion to control for multiple comparisons and to ensure the robustness of our results. Specifically, we excluded one subject at a time and performed the CCA analysis each time. The variability in the weightings, when each of the subjects was removed, was recorded. This gives an estimate of how much the results could change if more subjects were added.
Results
In demographic and clinical measures, only education was significantly different between males and females (Table 1). Female subjects had more years of education than male subjects (female/male: 15.3±2.2 and 13.7±2.7, uncorrected p=0.04, df=44). Among all the scores, the following tests showed significant differences between male and female subjects: WCST categories completed (female/male: 5.5±1.1 and 3.3±1.9, uncorrected p=1.97e-05, df=44), WCST trials to complete first category (female/male: 12.7±4.4 and 38.6±38.3, uncorrected p=3.32e-04, df=44), and TMT A (female/male: 30.7±12.3 and 40.3±13.3, uncorrected p=0.02, df=44) (Table 1), as well as Transformed TMT A (female/male: 0.2±0.0 and 0.2±0.0, uncorrected p=0.02, df=44) and B (female/male: 0.1±0.0 and 0.1±0.0, uncorrected p=0.003, df=44) scores.
CCA results showed that gender (mean canonical coefficient: timed=1.01, untimed=0.94) appears to be an important factor (i.e., weightings can be distinguished from zero) in the combination of demographic variables for both timed and untimed tests. In the untimed group, EDSS (mean canonical coefficient: 0.26) was influential in addition to gender. Age, education, and disease duration showed limited effects on both timed and untimed tests (mean canonical coefficient: timed/untimed=0.07/0.00, 0.03/−0.05, -0.02/−0.03, respectively) (Figures 1 and 2).
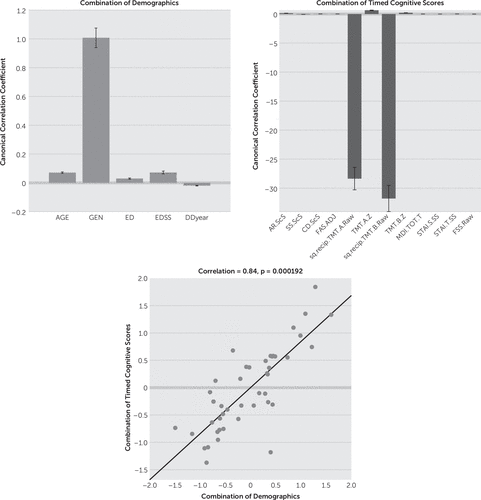
FIGURE 1. Canonical-Correlation Analysis (CCA) Results of Timed Cognitive Testsa
a The upper left panel shows that among five demographical variables, gender has a relatively strong effect in the combination. The upper right panel illustrates the weightings of every variable in the combination of timed cognitive scores based on their association with demographics (negative scores indicate females performing test better). TMT performances show strong effects (mean canonical coefficient: transformed TMT A and B=–28.3 and –31.80, respectively). The lower panel demonstrates the high correlation (r=0.84) between two sets of multivector (demographics and timed cognitive scores) with significance (p=0.0002). The error bars indicate how various the results are during the leave-one-out calculations. [AGE: age in years, GEN: gender, ED: education in years, EDSS: Expanded Disability Status Scale, DDyear: disease duration in years, AR.ScS: scaled WAIS Arithmetic, SS.ScS: standardized Symbol Search, CD.ScS: standardized Symbol Coding, FAS.ADJ: adjusted Verbal Letter Fluency, sq.recip.TMT.A.Raw: transformed Trail-Making Test, Part A scores, TMT.A.Z: Trail-Making Test, Part A Z scores, sq.recip.TMT.B.Raw: transformed Trail-Making Test, Part B scores, TMT.B.Z: Trail-Making Test, Part B Z scores, MDI.TOT.T: Multiscore Depression Inventory Total T scores, STAI.S.SS: standardized State-Trait Anxiety Inventory State scores, STAI.T.SS: standardized State-Trait Anxiety Inventory Trait scores, FSS.Raw: Fatigue Severity Scale Raw scores.]
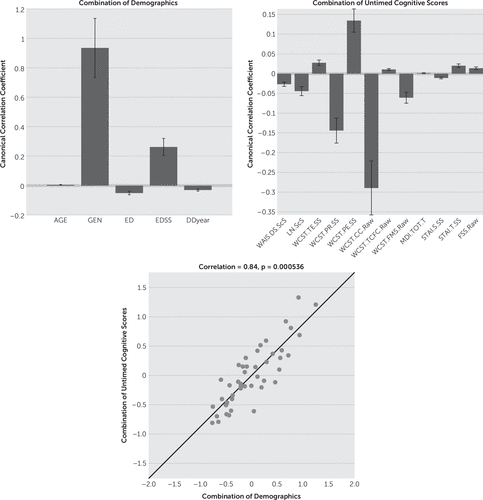
FIGURE 2. Canonical-Correlation Analysis (CCA) Results of Untimed Cognitive Testsa
a The upper left panel shows that gender and EDSS have strong effects in the combination of demographics compared with other variables. The upper right panel illustrates the weightings of every variable in the combination of untimed cognitive scores. WCST Errors showed weightings in male subjects as well as WCST Perseverative Errors, transit anxiety, fatigue, and WCST Trials to Complete First Category (mean canonical coefficient: 0.03, 0.14, 0.02, 0.01, and 0.01, respectively). WAIS IV Digit Span, WAIS IV Letter-Number Sequencing, WCST Perseverative Responses, WCST Categories Completed, Failure to Maintain Set, and state anxiety demonstrated effects in female subjects (mean canonical coefficient: –0.03, –0.04, –0.14, –0.29, –0.06, and –0.01, respectively). The lower panel demonstrates that these two sets of multivector (demographics and timed cognitive scores) are significantly correlated with each other (p<0.001, r=0.84). The errorbars indicate how various the results are during the leave-one-out calculations. [AGE: age in years, GEN: gender, ED: education in years, EDSS: the Expanded Disability Status Scale, DDyear: disease duration in years, WAIS.DS.ScS: scaled WAIS Digit Span, LN.ScS: scaled WAIS Letter Number Sequencing, WCST.TE.SS: scaled WCST Total Errors, WCST.PR.SS: standardized WCST Perserverative Responses, WCST.PE.SS: standardized WCST Perserverative Errors, WCST.CC.Raw: raw WCST Categories Completed, WCST.TCFC.Raw: raw scores of WCST Trails to Complete First Category, WCST.FMS.Raw: WCST Failure to Maintain Set, MDI.TOT.T: Multiscore Depression Inventory total T scores, STAI.S.SS: standardized State-Trait Anxiety Inventory State scores, STAI.T.SS: standardized State-Trait Anxiety Inventory Trait scores, FSS.Raw: Fatigue Severity Scale Raw scores.]
Since we assigned male gender as 1 and female gender as 0 in the calculation, the variables that showed positive canonical coefficient indicated higher scores in male subjects as long as gender also showed positive weightings. On the other hand, the tests on which female subjects obtained higher scores show negative canonical coefficients. This was because the model treated female gender (0) as “baseline.” The weightings of gender indicate the effects of male compared with female in combination of demographic variables, as well as the combination of cognitive scores. In the timed group, transformed TMT A and B scores showed strongly negative effects compared with other variables (mean canonical coefficient: –28.3 and –31.8, respectively) (Figure 1). On the contrary, the TMT A Z score demonstrated a positive mean canonical coefficient with 0.64 in the combination of cognitive scores, illustrating that there was a gender-specific pattern in timed cognitive tests where female subjects had higher transformed TMT A and B scores and male subjects had higher TMT A Z scores. In addition, cognitive scores and demographic variables were highly correlated with each other (correlation r=0.84, p<0.001 [Figure 1]), meaning that the linear combination of all the variables in demographics and all the variables in timed cognitive scores forms a significant model to explain and predict the data.
Figure 2 shows the results from the untimed group. Male subjects had higher scores (i.e., these scores showed higher weightings among male subjects) on WCST errors, WCST perseverative errors, trials to complete first category, trait anxiety, and fatigue (mean canonical coefficient: 0.03, 0.14, 0.01, 0.02, and 0.01, respectively), while female subjects obtained higher scores (i.e., these scores were more influential on the linear model of all scores among female subjects) on WAIS-IV digit span, WAIS-IV letter number sequencing, WCST perseverative responses, WCST categories completed, failure to maintain set, and state anxiety (mean canonical coefficient: –0.03, –0.04, –0.14, –0.29, –0.06, and –0.01, respectively). This implied that female subjects completed more categories on the WCST than males, but higher perseverative responses also indicated that females were potentially more prone to cognitive inflexibility as they changed strategies less frequently. Moreover, females also performed better on the digit span and letter-numbering sequencing tasks, meaning that they had better attentional abilities, since the scores of these two tests partially form WMI. Finally, the two sets of variables (combination of cognitive scores and combination of demographic characteristics) were highly correlated with each other in untimed group (correlation r=0.84, p<0.001 [Figure 2]), indicating that the linear combination of all the variables in demographics and untimed cognitive scores forms a model explaining the data.
Discussion
With the CCA method, we have reported that 1) there were gender-specific cognitive patterns in MS and 2) some demographical variables had stronger effects on cognitive performances. More importantly, utilizing a focused test battery of assessments sensitive to aspects of executive functioning, there were gender differences. The findings from the present study endorse the need for sensitivity to include both untimed and timed tasks in the cognitive assessment of individuals with remitting and relapsing MS.
Gender is an Influential Factor in Cognition Among MS Subjects
Gender differences have been reported in previous studies showing that females perform better than males on memory tasks and the WCST.4,9 Moreover, male sex has been speculated as a risk factor for development of severe cognitive decline.8 These studies investigated cognitive function based on the responses on cognitive tests among males and females separately. Given that cognition is a complex multidimensional entity that can be assessed within different domains,18 it is reasonable to assume that there are multiple intercorrelated variables modulating cognition. Therefore, we investigated the intercorrelation from combinations of demographic and cognitive variables through CCA, determining which linear combination of demographic and cognitive scores best represent the data among the two genders as a whole. CCA is an approach for exploring relations between two multivariate sets of variables and evaluating which linear combinations of variables can best explain the variability. In this study, the cognitive/affective scores and demographical indices were the two multivariate sets of variables in both groups. Since we did not normalize variables in our analysis, we could only interpret high weightings as “important factors” (i.e., significantly different from zero) rather than “the most important factors,” as they were not on the same scale. Several cognitive scores have been normalized based on normal population; therefore, it would not be reasonable to normalize all the scores again.
As shown in Figures 1 and 2, gender was a strong demographic factor in timed tests, and both gender and EDSS were influential in untimed tests, as the remaining variables had limited effects (weightings <0.1) (Figures 1 and 2). Demographical factors were taken into account in our model, which highlighted the fact that both EDSS and gender were influential factors for cognition in our data. Our results were in agreement with previous research showing that gender was a predictor for cognitive dysfunction in MS and male subjects generally showed worse performance.7,8
Education and affective states can directly affect cognition.19 However, in this study, education did not show effects on cognitive profile even though female subjects had higher education. Moreover, none of the affective variables were significantly different between the two genders, and all of them had limited weightings in CCA results. Although there were trends that the females in this study were more educated, it was not a significant difference (see Table 1). Therefore, we concluded that education and affective status did not significantly influence cognition in our cohort.
Distinct Cognitive Patterns in Male and Female Subjects With Remitting and Relapsing MS
Instead of evaluating cognition by individual tests, we assessed the cognitive profile in MS by including more than one variable in our calculation. Due to the complexity of human cognition, different cognitive performances have relationships with demographic variables. Therefore, we included all the cognitive and demographical variables in our model and separated cognitive scores into two groups: timed and untimed groups.
Because transformed TMT scores were the square roots of reciprocal TMT raw scores (which had opposite meanings to raw TMT scores), our interpretation of high weightings in TMT scores (Figure 1) is that female subjects performed better on both TMT A and B (i.e., faster responses). We conclude that set-shifting abilities assessed by TMT A and B were less impaired in female MS subjects than in males. Finally, the high correlation between the combination of demographic characteristics and combination of timed cognitive scores illustrated that these linear combinations were significant and robust enough to explain the data.
Figure 2 demonstrates that gender had strong weightings in the combination of demographic variables and untimed scores. Among all the untimed scores, male subjects obtained high weightings on WCST perseverative errors and errors, illustrating poor performance on the WCST. In contrast, females tended to perform better on the WCST because they had higher scores on WCST categories completed. However, they also had higher scores on WCST perseverative responses and failure to maintain set, which possibly indicates an inability to use feedback to modify their response behavior. It is interesting to know that perseverative behavior, whether errors or responses, are found among both genders. This is more indicative of the neuropathology (i.e., white matter damages reduce neuronal communication) seen in MS. In addition, females obtained higher scores on WAIS-IV digit span and letter-number sequencing tests, indicating that, compared with male subjects, their basic ability to sustain attention was less affected.
Finally, the untimed group also demonstrated high correlations between the combination of cognitive scores and combination of demographic variables, and again, gender and a subset of WCST scores were the strong factors that explained cognitive patterns.
Separating Timed/Untimed Tests is More Sensitive to Detect Gender Differences in MS
Gender differences on cognitive tasks have been long established.20 Separating the timed from nontimed tasks is an important, growing trend in the analysis of neuropsychological test data in MS.21
We also analyzed timed and untimed scores together, but the model was not significant. The current results indicate that analyzing timed and untimed scores separately is more effective in distinguishing cognitive profiles between male and female MS subjects.
Limitations
There were only 13 male subjects in our study, and only one of them had a high EDSS score, which raises the issue of sample size and that the tested population may not have been sufficiently representative of the male MS population. Although we obtained a fairly sufficient amount of data for each individual, the total number of subjects was not as large as that of other studies that focused on behavioral data. This was because the original design of the study was to investigate the association between behavioral data (clinical indices and neuropsychological scores) and neuroimaging findings such as functional connectivity and structural integrity. This cohort was relatively large for imaging studies but small for analyses of neuropsychological variables. In our cohort, females had more education then males (approximately 2 years), but the range of years of education was very similar for both males and females. It is quite possible that this education advantage may be contributing to cognitive reserve and better performance on psychometric tests. This will need to be replicated in future studies. In addition, this study only examined the relations between demographic variables and multiple measures of executive function. The same approach should be implemented to study how demographics are related to other neuropsychological functions. Finally, since our aim was to investigate the “relationships” and “combinations” between variables in MS, we did not include healthy subjects in the analyses.
Executive Functioning and Localization
As mentioned previously, our original investigation was to examine executive functioning and connectivity in patients with remitting and relapsing MS. The test battery for this study was based on widely used tasks in assessing executive functioning.
In neuropsychology, our understanding of brain functioning and localization has evolved over time and has been challenged with the development of imaging techniques such as fMRI. More importantly, skilled clinicians have become astutely aware that tasks such as the WCST may not be localized specifically to certain brain regions as originally proposed, for example, to the dorsolateral prefrontal cortex or to the left hemisphere.22 However, there are many variables contributing to the diversity of findings, including what WCST administration technique was used, were the norms based on normal controls, neurological patients (i.e., patients with epilepsy) versus psychiatric populations. Again, a skilled clinician has awareness of these findings but can still utilize the task to comment on impairment on executive skills such as concept formation, set switching, maintaining set, and ability to utilize feedback to change behavior, as well as providing evidence for perseveration.
Conclusions
Taken together, we conclude that gender is one of the influential factors on cognitive performances in subjects with MS. This study utilized cognitive tasks specifically involving frontal connectivity. Moreover, there are specific cognitive patterns in MS subjects. First, TMT A and B tests have the strong weightings that distinguish from zero in female subjects, meaning that female subjects performed TMT tests better than males. Second, in untimed tests, male subjects made more errors and female subjects performed better on the WCST. Finally, our results imply that with this particular test battery, female subjects with MS were less cognitively impaired than male subjects. Our study is unique in that we have discovered gender differences exist on selected executive tasks and report a trend that gender differences may also exist on timed tasks versus untimed tasks, which has become an important area of focus in the cognitive assessment of individuals with remitting and relapsing MS. These findings need to be replicated but promote the importance of selecting tests and test batteries that are sensitive to these issues.
To our knowledge, no prior research has examined the relation between cognition and demographic variables using CCA in MS. With this approach, we were able to reveal gender-specific cognitive patterns in MS. These findings have implications for not only the assessment of cognitive functioning in MS, but also our understanding of sex differences in cognition in the presence of chronic illnesses.
1 : Multiple sclerosis: diagnosis, differential diagnosis, and clinical presentation, in Handbook of Clinical Neurology Elsevier, 2014, pp 269–290Crossref, Google Scholar
2 : Sex and gender issues in multiple sclerosis. Ther Adv Neurol Disord 2013; 6:237–248Crossref, Medline, Google Scholar
3 : Cognitive dysfunction in multiple sclerosis: Assessment, imaging, and risk factors. J Rehabil Res Dev 2006; 43:63–72Crossref, Medline, Google Scholar
4 : Gender-related differences in functional connectivity in multiple sclerosis. Mult Scler 2012; 18:164–173Crossref, Medline, Google Scholar
5 : Gender-related effect of clinical and genetic variables on the cognitive impairment in multiple sclerosis. J Neurol 2004; 251:1208–1214Crossref, Medline, Google Scholar
6 : Sex-specific extent and severity of white matter damage in multiple sclerosis: implications for cognitive decline. Hum Brain Mapp 2014; 35:2348–2358Crossref, Medline, Google Scholar
7 : Subcortical atrophy and cognition: sex effects in multiple sclerosis. Neurology 2012; 79:1754–1761Crossref, Medline, Google Scholar
8 : Risk factors for and management of cognitive dysfunction in multiple sclerosis. Nat Rev Neurol 2011; 7:332–342Crossref, Medline, Google Scholar
9 : Sex differences in cognitive impairment in multiple sclerosis. Clin Neuropsychol 2002; 16:472–480Crossref, Medline, Google Scholar
10 : Cognitive dysfunction in multiple sclerosis. I. Frequency, patterns, and prediction. Neurology 1991; 41:685–691Crossref, Medline, Google Scholar
11 : Validity of the minimal assessment of cognitive function in multiple sclerosis (MACFIMS). J Int Neuropsychol Soc 2006; 12:549–558Crossref, Medline, Google Scholar
12 : Identification and clinical impact of multiple sclerosis cortical lesions as assessed by routine 3T MR imaging. AJNR Am J Neuroradiol 2011; 32:515–521Crossref, Medline, Google Scholar
13 : Recommendations for a Brief International Cognitive Assessment for Multiple Sclerosis (BICAMS). Mult Scler 2012; 18:891–898Crossref, Medline, Google Scholar
14 : Principles of Multivariate Analysis: A User’s Perspective. New York, Clarendon Press, 1988Google Scholar
15 : Putting things in even better order: the advantages of Canonical correlation analysis. Ecology 1993; 74:2215–2230Crossref, Google Scholar
16 : Fast active appearance model search using canonical correlation analysis. IEEE Trans Pattern Anal Mach Intell 2006; 28:1690–1694Crossref, Medline, Google Scholar
17 : Canonical correlation analysis for feature-based fusion of biomedical imaging modalities and its application to detection of associative networks in Schizophrenia. IEEE J Sel Top Signal Process 2008; 2:998–1007Crossref, Medline, Google Scholar
18 : New thinking: the evolution of human cognition. Philos Trans R Soc B Biol Sci 2012; 367:2091–2096Crossref, Medline, Google Scholar
19 : MS patients with depressive symptoms exhibit affective memory biases when verbal encoding strategies are suppressed. J Int Neuropsychol Soc 2005; 11:514–521Crossref, Medline, Google Scholar
20 : The new science of cognitive sex differences. Trends Cogn Sci 2014; 18:37–45Crossref, Medline, Google Scholar
21 : Does slowed processing speed account for executive deficits in multiple sclerosis? Evidence from neuropsychological performance and structural neuroimaging. Rehabil Psychol 2014; 59:422–428Crossref, Medline, Google Scholar
22 : The Wisconsin Card Sorting Test and the cognitive assessment of prefrontal executive functions: a critical update. Brain Cogn 2009; 71:437–451Crossref, Medline, Google Scholar